Wearable multi-sensor data fusion approach for human activity recognition using machine learning algorithms
SSRN Electronic Journal(2022)
摘要
Wearable sensor based human activity recognition (HAR) has a broad range of applications in healthcare, fitness, smart home, and surveillance. In spite of the substantial amount of computational research on HAR, the open challenges in multi-sensor based activity recognition including complex temporal data, identifying discriminative feature vectors from multi-modal data and dimensionality reduction require considerable research attention. Hence, in this paper, using multi-resolution time-frequency analysis of received signal strength (RSS) between the wearable sensors, we present a supervised machine learning (ML) based activity recognition framework. The multi-sensor activity data acquired using the wireless sensor network (WSN) nodes and inertial-sensor embedded in a smartphone are decomposed using the discrete wavelet transform (DWT) and the empirical mode decomposition (EMD) techniques for extracting the prominent feature vector. Using the discriminative statistical features from DWT along with the entropy features from EMD, the four ML classifier models such as support vector machine (SVM), K-nearest neighbour (KNN), ensemble classifier (EC), and decision tree (DT) are trained to classify various human activities. The efficacy of the proposed design is assessed on a publicly available dataset from UCI. The experimental results assessed using the confusion matrix and parallel coordinate plot (PCP) substantiate that the proposed ML based HAR framework can achieve a maximum classification accuracy of 99.63% and is superior to several state-of-the-art ML techniques.
更多查看译文
关键词
HAR,Wearable sensors,DWT,EMD,WSN,Supervised classifiers
AI 理解论文
溯源树
样例
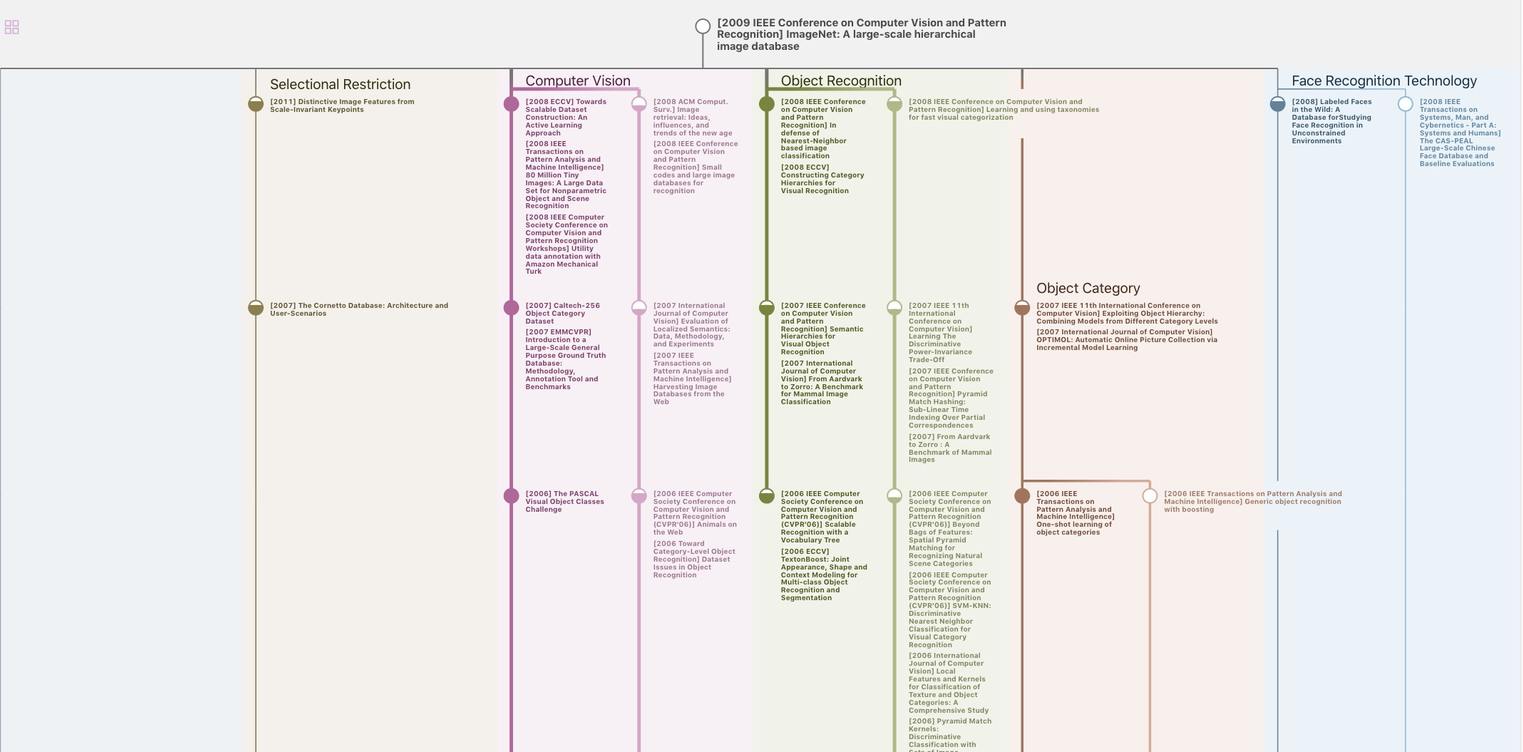
生成溯源树,研究论文发展脉络
Chat Paper
正在生成论文摘要