A random grouping-based self-regulating artificial bee colony algorithm for interactive feature detection
Knowledge-Based Systems(2022)
摘要
As the dimensionality and complexity of datasets increase, such as biological data, the demand for fast and efficient technologies to detect relevant feature information from these data is also increasing. Extensive progress has been made in the development of evolutionary algorithms (EAs), which can detect the association between a single feature and class label in high-dimensional data. However, when facing the high-dimensional problem caused by interactions between features, the search space of EAs increases exponentially with an increase in the number of features, resulting in unsatisfactory solution quality. Therefore, this study proposed a random grouping-based self-regulating artificial bee colony algorithm (RCABC). RCABC first decomposes all dataset features into a series of dynamic subsets using a dynamic random grouping (DRG) strategy. Thereafter, a self-regulating bee colony optimizer (S-Optimizer) is used to detect relevant interactive features in each subset. This study demonstrates the superiority of the proposed algorithm through experimental results on synthetic and real-world biological datasets.
更多查看译文
关键词
Evolutionary algorithm,Dynamic random grouping,High-dimensional data,Interactive features
AI 理解论文
溯源树
样例
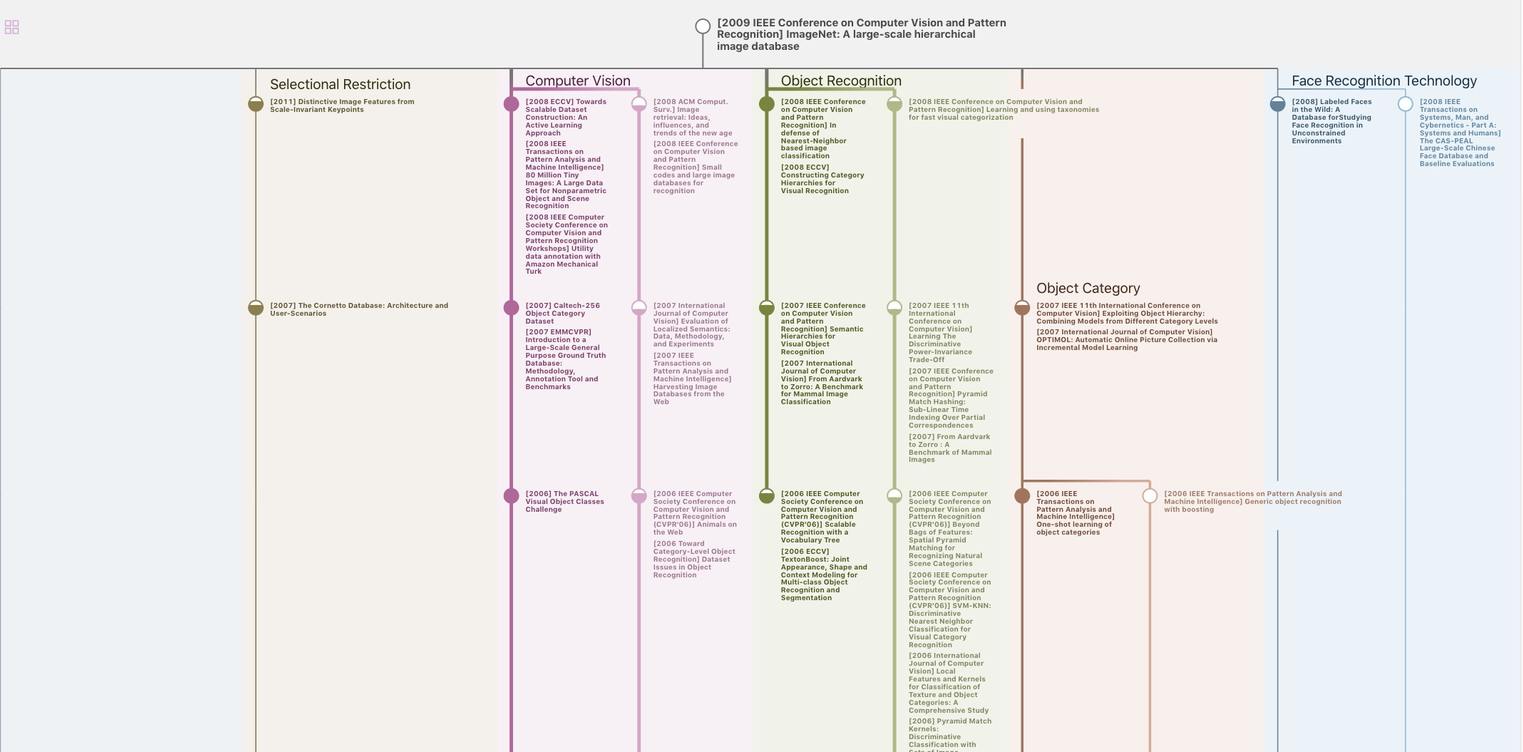
生成溯源树,研究论文发展脉络
Chat Paper
正在生成论文摘要