Physics-informed neural network for predicting electric field distributions and permittivities of circular split-ring resonators
AI and Optical Data Sciences III(2022)
摘要
Physics informed neural networks (PINNs) solve supervised learning tasks by incorporating partial differential equations describing the governing physics. We use a PINN based on Maxwell’s equations in the frequency domain to predict the electrical permittivity parameters, and hence the electric fields, of circular split-ring resonator-based metamaterials thereby bypassing full-wave solutions based on finite-element methods. We demonstrate the use of a PINN for the inverse prediction of the electrical permittivity of a circular split ring resonator metamaterial given the spatial e-field distributions at the resonant frequency. Our results validate the PINN framework for the inverse retrieval of permittivities from field distributions.
更多查看译文
关键词
permittivities,electric field distributions,electric field,neural network,physics-informed,split-ring
AI 理解论文
溯源树
样例
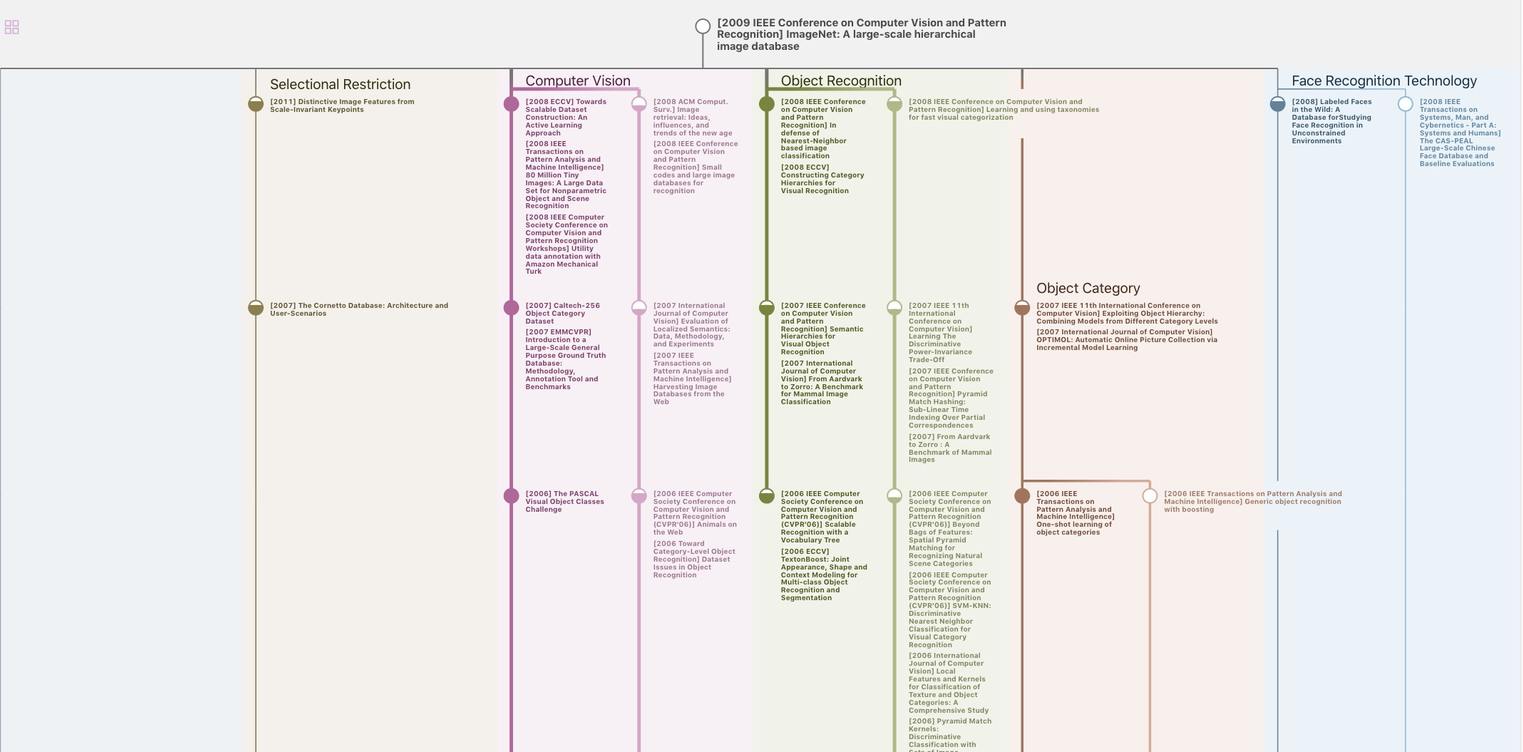
生成溯源树,研究论文发展脉络
Chat Paper
正在生成论文摘要