Latest concrete materials dataset and ensemble prediction model for concrete compressive strength containing RCA and GGBFS materials
CONSTRUCTION AND BUILDING MATERIALS(2022)
摘要
Testing the compressive strength of concrete using machine learning approaches has high importance for civil engineering. Machine learning approaches provides high accuracy with reduced cost and time. However, such approaches require concrete composition data detailing the type and quantitative ratio of different materials such as water, cement, and aggregate, etc. This study incorporates a dataset where the composition of 125 different kinds of materials has been recorded. Extensive literature review has been carried out for data collection and annotation. The dataset includes traditional, as well as, advanced materials containing both RCA (recycled concrete aggregate) and GGBFS (ground granulated blast furnace slag) along with other main ingredients of concrete mix. Adding RCA and GGBFS to the concrete mix helps to produce environment friendly concrete because these materials are waste and by-products. However, increasing the number of ingredients in the concrete complicates the prediction of concrete compressive strength. Increasing RCA for concrete reduces its mechanical strength which is managed by adding GGBFS, however, the strength depends on the ratios of RCA and GGBFS. So, the compressive strength prediction of concrete containing RCA and GGBFS is crucial task for ensuring the safety of construction projects. This study presents two ensemble models for the accurate prediction of compressive strength new concrete that contains RCA and GGBFS. First ensemble, LRF, combines the LR (linear regression) and RF (random forest) through soft voting. For the second ensemble, CNN (convolutional neural networks) and LSTM (long short term memory) are leveraged. Models' performance is evaluated through several well-known metrics including R-2 (R-square), root mean square, mean absolute error, and mean square error. Results indicate that LRF and CNN-LSTM achieve the highest R-2 scores of 0.93 and 0.96, respectively than the state-of-the-art models. LRF is more efficient as compared to CNN-LSTM in terms of computational time. Compared to the traditional concrete strength estimation, machine learning-based compressive strength prediction is accurate, robust and precise.
更多查看译文
关键词
Recycled coarse aggregate,Ground granulated blast furnace slag,Concrete compressive strength,Ensemble classifier,Machine learning
AI 理解论文
溯源树
样例
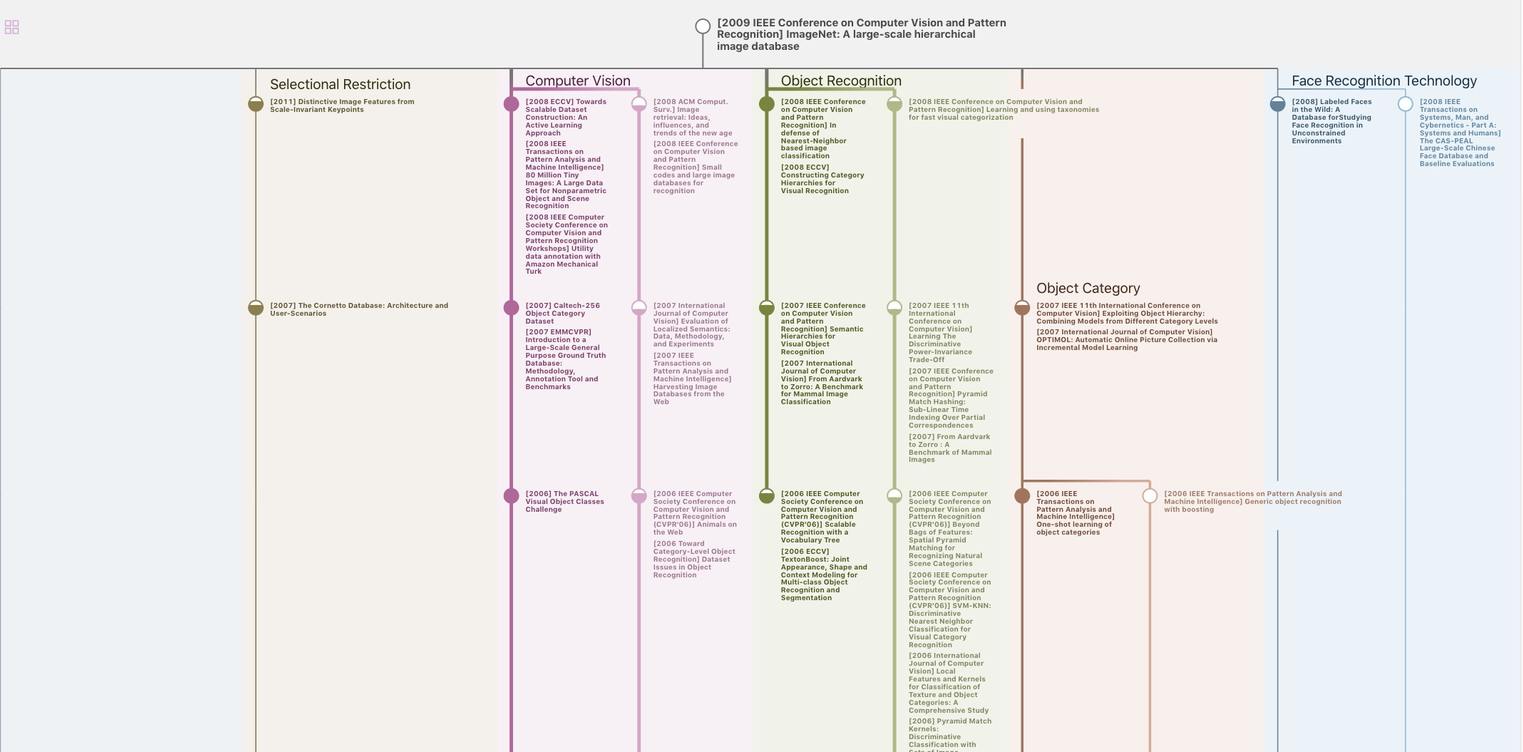
生成溯源树,研究论文发展脉络
Chat Paper
正在生成论文摘要