Beyond Global Average Pooling: Alternative Feature Aggregations for Weakly Supervised Localization
PROCEEDINGS OF THE 17TH INTERNATIONAL JOINT CONFERENCE ON COMPUTER VISION, IMAGING AND COMPUTER GRAPHICS THEORY AND APPLICATIONS (VISAPP), VOL 4(2022)
摘要
Weakly supervised object localization (WSOL) enables the detection and segmentation of objects in applications where localization annotations are hard or too expensive to obtain. Nowadays, most relevant WSOL approaches are based on class activation mapping (CAM), where a classification network utilizing global average pooling is trained for object classification. The classification layer that follows the pooling layer is then repurposed to generate segmentations using the unpooled features. The resulting localizations are usually imprecise and primarily focused around the most discriminative areas of the object, making a correct indication of the object location difficult. We argue that this problem is inherent in training with global average pooling due to its averaging operation. Therefore, we investigate two alternative pooling strategies: global max pooling and global log-sum-exp pooling. Furthermore, to increase the crispness and resolution of localization maps, we also investigate the application of Feature Pyramid Networks, which are commonplace in object detection. We confirm the usefulness of both alternative pooling methods a, well as the Feature Pyramid Network on the CUB-200-2011 and OpenImages datasets.
更多查看译文
关键词
Computer Vision, Pooling, Weakly Supervised Object Localization, Weakly Supervised Segmentation
AI 理解论文
溯源树
样例
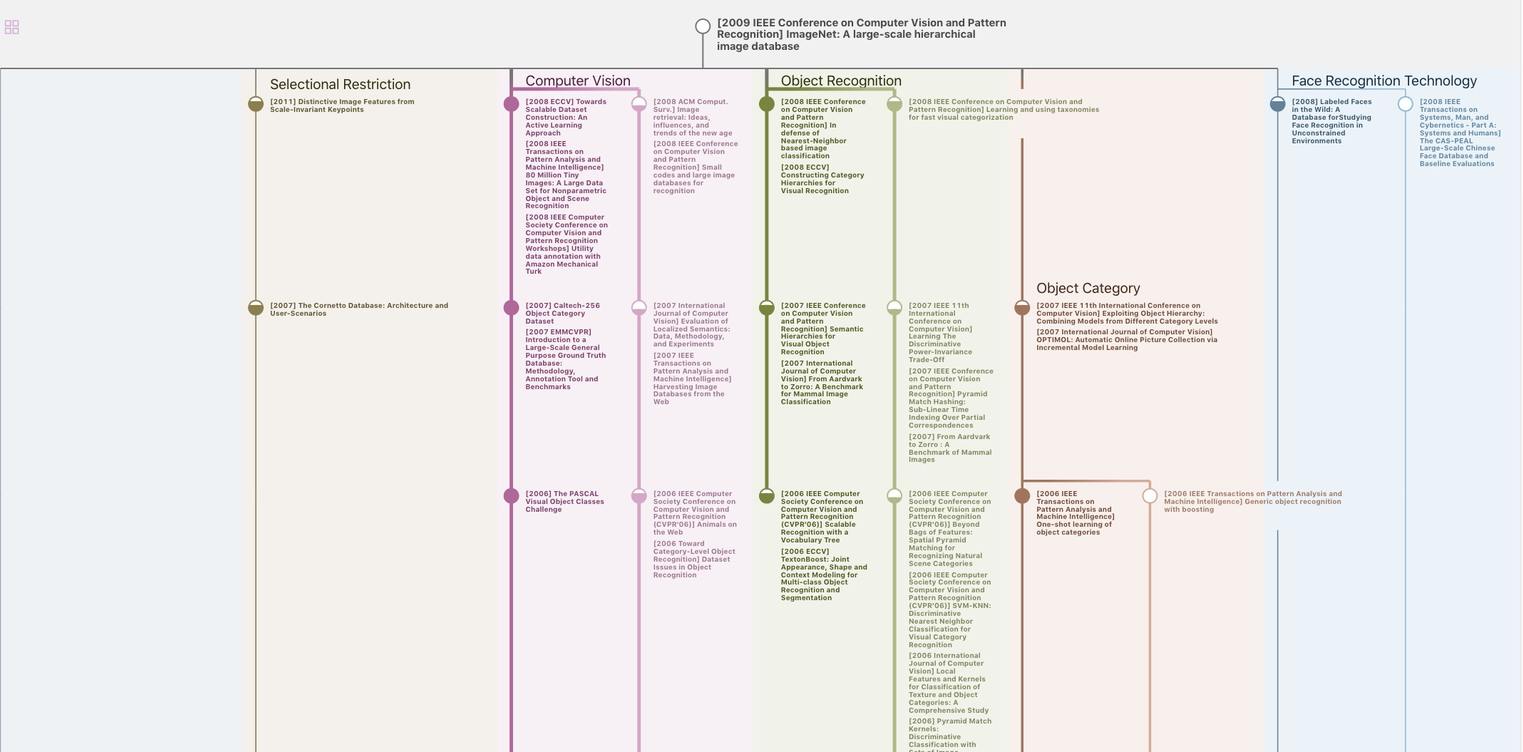
生成溯源树,研究论文发展脉络
Chat Paper
正在生成论文摘要