Antenna radiation pattern predictions with machine learning
2021 IEEE Conference on Antenna Measurements & Applications (CAMA)(2021)
摘要
A machine-learning based method to characterize integrated antennas is presented. The technique allows fast characterization with significantly reduced complexity compared to the previous antenna tests with separate scanned probe and receiver. The broadband reflection from a quasirandom target conveys the antenna characteristics in the reflection coefficient or S11 -parameter measurement. A neural network is trained to retrieve the beam characteristics from the measured reflection coefficient S11 . The antenna measurement setup is simulated as a reflection measurement with the antenna under test (AUT) facing quasirandom reflective mask. The reflection coefficient is calculated as the coupling coefficient between the AUT radiated field and the back-reflected field at 75-110 GHz, and it is fed to a fully-connected neural network and trained to the beam-steering angles and beamwidths. The predicted median beam direction error is 4.1° and beamwidth error is 2.2°. The technique is promising, as it allows for antenna characterization without scanned or rotated antennas, yet providing sufficient accuracy for antennas with moderate directivity.
更多查看译文
关键词
antenna radiation pattern predictions,machine learning,machine-learning based method,integrated antennas,fast characterization,previous antenna tests,separate scanned probe,receiver,broadband reflection,quasirandom target,antenna characteristics,S11-parameter measurement,beam characteristics,measured reflection coefficient,antenna measurement setup,reflection measurement,AUT,quasirandom reflective mask,coupling coefficient,fully-connected neural network,beam-steering angles,beamwidths,median beam direction error,antenna characterization,frequency 75.0 GHz to 110.0 GHz
AI 理解论文
溯源树
样例
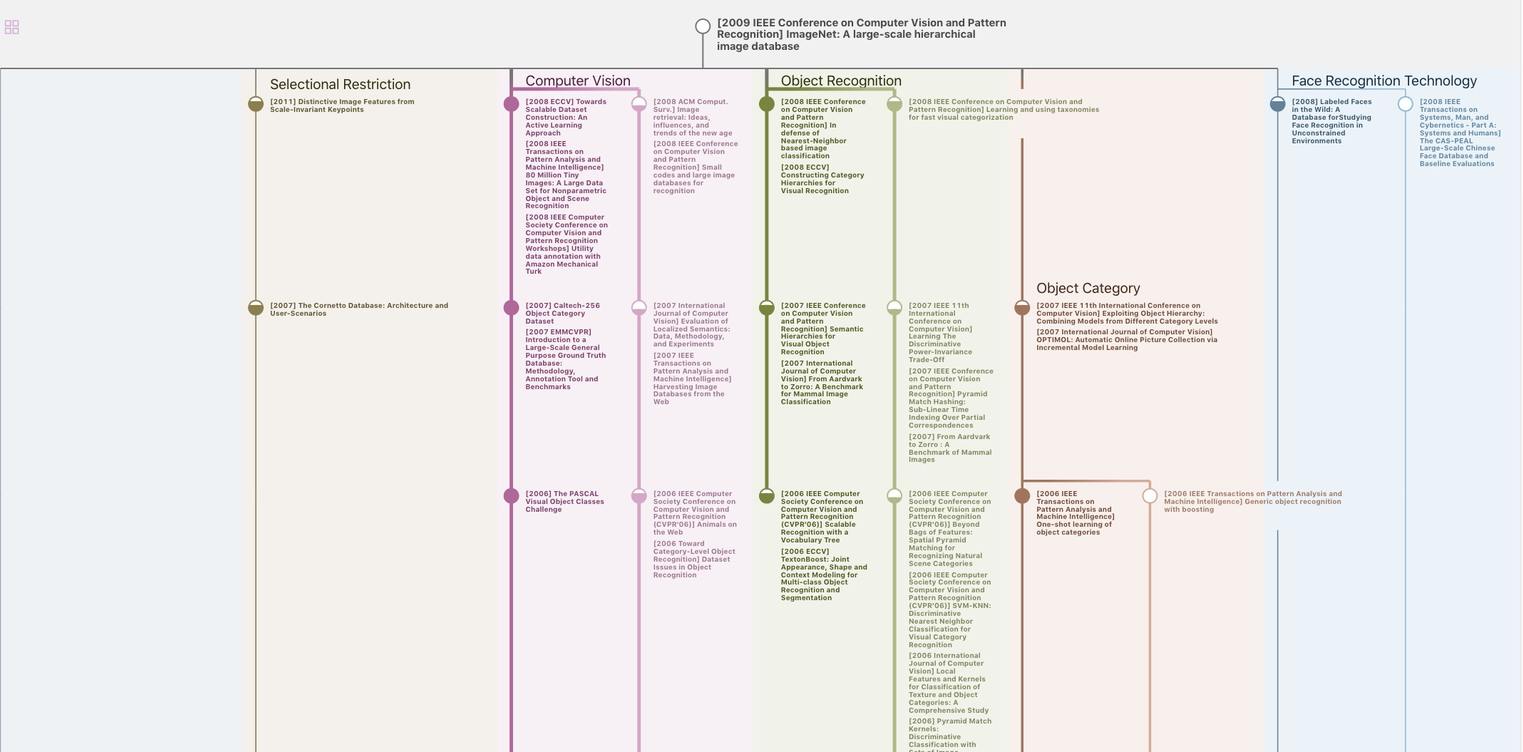
生成溯源树,研究论文发展脉络
Chat Paper
正在生成论文摘要