Machine learning predicts all-cause mortality in patients with severe aortic regurgitation
European Heart Journal - Cardiovascular Imaging(2022)
摘要
Abstract Funding Acknowledgements Type of funding sources: None. Background Aortic regurgitation (AR) is a common valvular lesion associated with left ventricular (LV) enlargement and dysfunction and increased risk of death with onset of symptoms, significant LV enlargement, or systolic dysfunction. The current guidelines are based on symptoms, LV size and LV function to evaluate the candidacy for surgery in patients with severe AR. Purpose We propose a machine-learning (ML) based algorithm to identify patients at risk for death from AR independent of aortic valve replacement (AVR) surgery (patients were censored at AVR event unless the operation occurred within 100 days of the first visit, in which case baseline AVR is included as a predictor). Methods The model was trained with 5-fold cross-validation on a dataset of 1035 patients. Model performance is reported on an independent dataset of 207 patients. Optimal predictive performance was observed with a Conditional Random Survival Forest model. A subset of 19 / 41 variables were selected for inclusion in the final model. Variable selection was performed with 10-fold cross validation using Random Survival Forest model; variables that were on an average selected by less than 6/10 splits were excluded. Results The mean age of patients was 60 ± 17 years and 198 (18%) were females. The variables included in the models were age, end-diastolic volume, end systolic dimension, body surface area, ejection fraction, NYHA class, body mass index, diastolic blood pressure, bicuspid valve, regurgitant volume, filling pressure, mid ascending aorta diameter, mitral tissue early relaxation velocity, pulmonary artery systolic pressure, and comorbidities, and the relative variable importances averaged across five splits of cross validation in each repeat were evaluated (Figure). The predictive AUC for predicting survival of the best-performing model was 0.84 at 1 year, 0.86 at 2 years, and 0.87 overall, respectively. Conclusion Using commonly observed echo parameters and patient characteristics we successfully trained a ML model to predict survival in patients with severe AR. This technique could identify high-risk patients who would benefit from early intervention, thereby improving patient outcomes. Abstract Figure.
更多查看译文
AI 理解论文
溯源树
样例
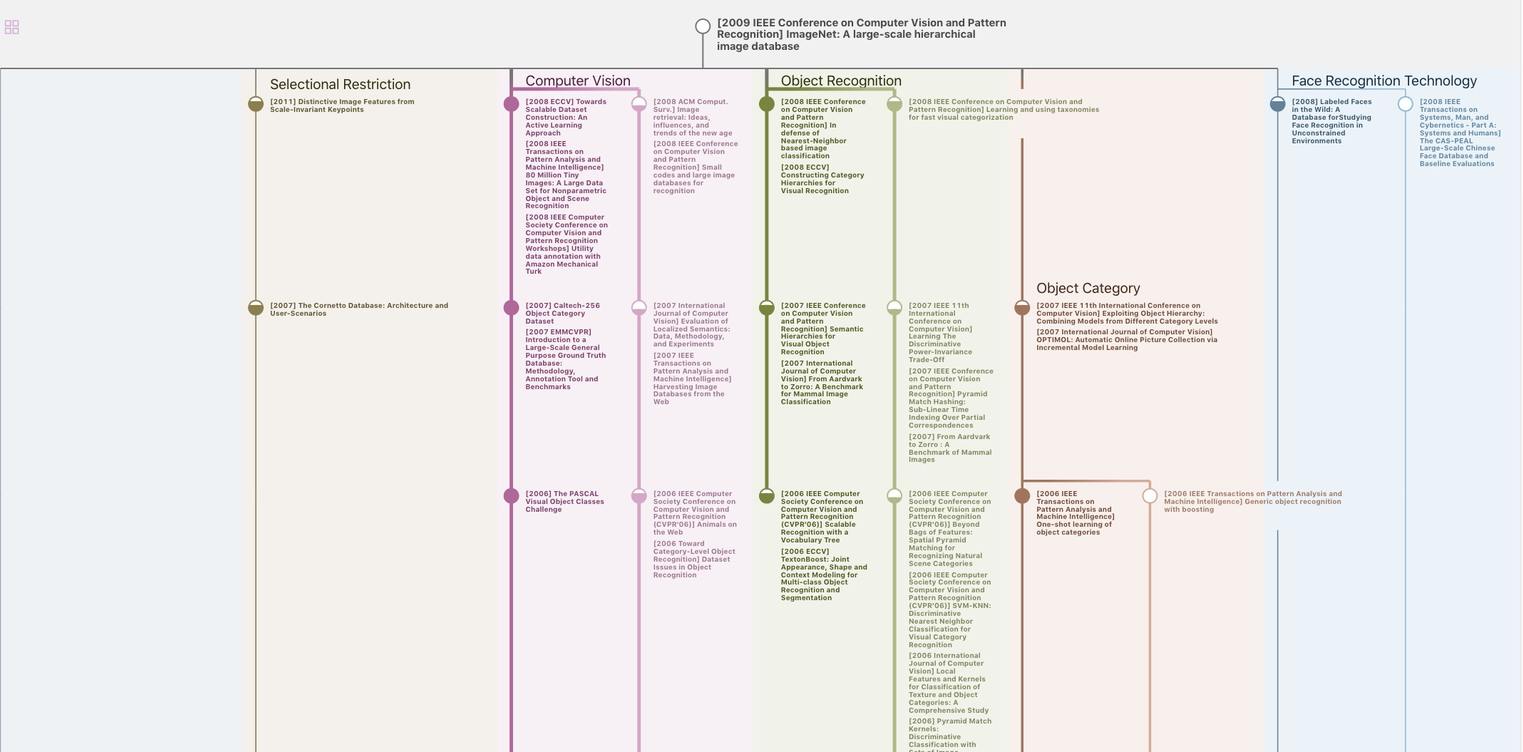
生成溯源树,研究论文发展脉络
Chat Paper
正在生成论文摘要