A fusion representation for face learning by low-rank constrain and high-frequency texture components
Pattern Recognition Letters(2022)
摘要
Face image processing has always been an important field of artificial intelligence. In machine learning tasks, samples are vectorized into a data matrix. Hence, different faces lie in several respective low-rank subspaces, which can be effectively captured by Low-Rank Representation (LRR) learning. However, most technologies usually use the raw data directly but ignore the weight among different information of the image itself. Following the intuition that human beings distinguish different people through the lineaments and wrinkles of faces which are indeed the texture components of face images, it is believed that representation learning will benefit from introducing the texture components of samples into the information fusion. Inspired by Multi-view learning models which can reconcile the knowledge gained from different views, a fusion framework is proposed in this letter, named High-Frequency texture components Low-Rank Representation (HFLRR). In HFLRR, the high-frequency texture components of samples will be extracted by Fast Fourier Transformation and Butterworth filtering firstly. Subsequently, the texture information will be introduced into the subspace learning procedure. An effective optimization solution is presented for this framework and the experimental results show that the proposed algorithm outperforms the state-of-art methods on several real-world face datasets. (C) 2022 Elsevier B.V. All rights reserved.
更多查看译文
关键词
High-frequency signal,Texture component,Low-Rank representation
AI 理解论文
溯源树
样例
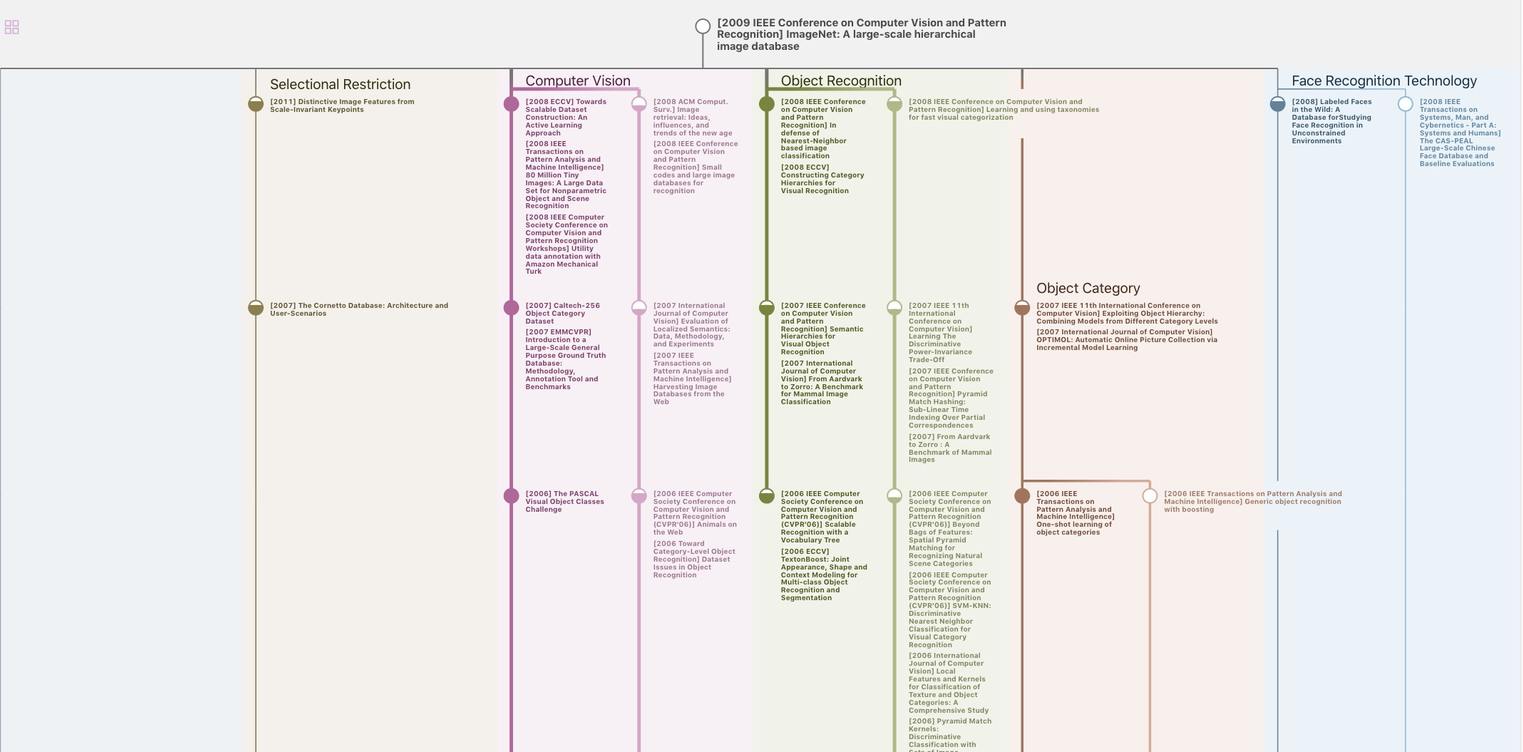
生成溯源树,研究论文发展脉络
Chat Paper
正在生成论文摘要