Abstract WMP64: Multimodal Bayesian Modeling Of Stroke Severity
Stroke(2022)
摘要
Introduction: Understanding the relations between lesions and outcomes is a particularly promising avenue to support tailored stroke care. We here employed a novel Bayesian framework integrating lesion location and functional lesion connectivity, i.e., lesion network mapping data, aiming to augment the prediction of stroke severity and the interpretability of lesion-symptom associations in a multi-center cohort of acute ischemic stroke (AIS) patients. Methods: Analyses relied on 1,077 AIS patients of the MRI-GENIE study [age: 64.2(14.7), 38%women]. NIHSS-based stroke severity was modeled via Bayesian linear regression. Structural location and functional connectivity information was represented by ten unique patterns each. We compared the out-of-sample predictive performances of structural vs. structural-functional models in Bayesian model comparisons. In ancillary analyses, we extracted functional connectivity pattern relevant for stroke severity and repeated analyses after stratifying for lesion size (50% of patients with larger lesions). Models were generally adjusted for age, sex, comorbidities and total DWI lesion volume. Results: Model comparison indicated a benefit of the combined structural-functional stroke severity prediction model compared to the structural one (model weights: 82% vs. 18%). This benefit of the combined model became even more apparent in case of patients with large lesions (97% vs. 3%). Positive lesion connectivity to the bilateral ventral attention (VA) and somatomotor networks, as well as temporal default mode network (DMN) predicted higher severity, while positive connectivity to more medial parts of the DMN and the visual network predicted lower severity. Additionally, negative lesion connectivity to the bilateral DMN, somatomotor, dorsal attention, and control networks predicted higher, and negative connectivity to the right control and bilateral VA networks predicted lower severity. Conclusions: Model comparisons indicated a benefit of integrating structural and functional information for the prediction of stroke severity, especially in case of larger lesions. Lesion connectivity data can enhance our understanding of why specific lesion locations can lead to symptoms post-stroke.
更多查看译文
AI 理解论文
溯源树
样例
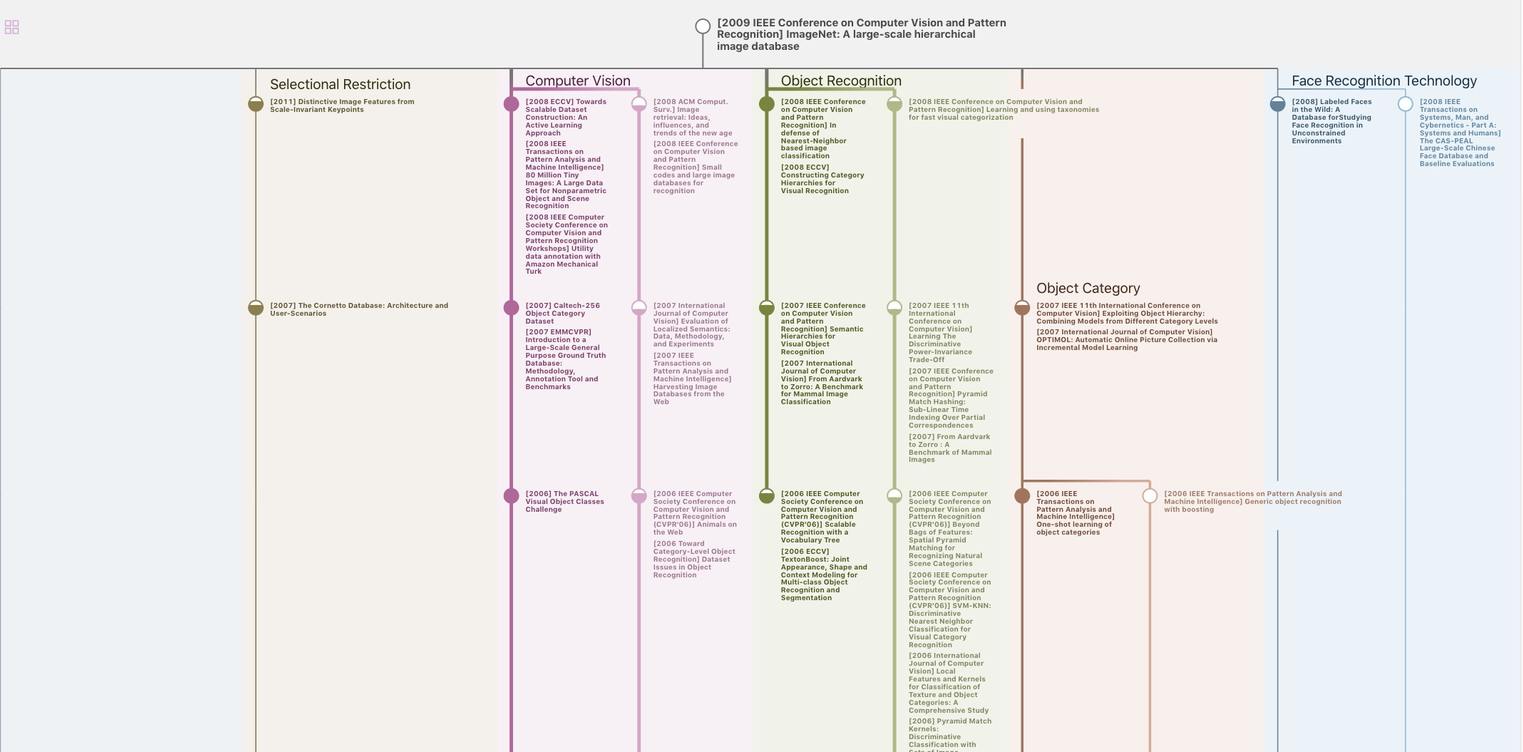
生成溯源树,研究论文发展脉络
Chat Paper
正在生成论文摘要