Tumour segmentation with deep learning model trained on immunostain-augmented pixel-accurate labels – Application on whole slide images of breast carcinoma
Pathology(2022)
摘要
Introduction: Whole slide images are high resolution and data intensive. Traditional manual annotation is unable to produce high-definition pixel accurate labels. Augmentation by corresponding immunostain images overcomes operator dependent annotation limits. Methods: H&E slides from breast carcinoma resection specimens were retrieved and reviewed for presence of tumour. The H&E slides with tumour were scanned using whole slide scanner Leica AT2 at 0.25 um per px. AE1/3 (pancytokeratin) stain was performed on the same slides after de-staining the H&E dyes. The scanned AE1/3 images were aligned to the H&E images and immunoreative areas were used to generate annotations of tumour areas to the corresponding H&E slides. The annotations were checked and modified by two independent assessors, converted into binary masks. The cases were randomly assigned to the training or testing cohort with a train-test split of 3:1. The masks and paired H&E images were fed into a U-Net based deep learning model for tumour detection and segmentation. Results: The annotations resulting from the AE1/3 image were pixel accurate and the assessors were only required to highlight the rough tumour areas in bulk only. A total of 101 specimens were included, 75 and 26 were assigned to the training and validation cohort. The algorithm achieved a per-pixel accuracy of 0.928 (0.827–0.998) for tumour segmentation in the testing cohort. The tumour segmentation results were able to precisely identify carcinoma areas from peritumoral stromal area. Conclusion: Immunostain-augmentation increases annotation accuracy and efficiency, resulting in pixel-accurate annotations, from which deep learning generates models that precisely maps tumour on whole slide images. Immuonstain-augmentation with deep learning has potential to provide large scale and reliable data for cancer staging, cellular and microenvironment spatial analysis and three-dimensional tumour reconstruction.
更多查看译文
关键词
Whole Slide Imaging,Cancer Imaging,Tumor Heterogeneity,Medical Image Analysis,Medical Imaging
AI 理解论文
溯源树
样例
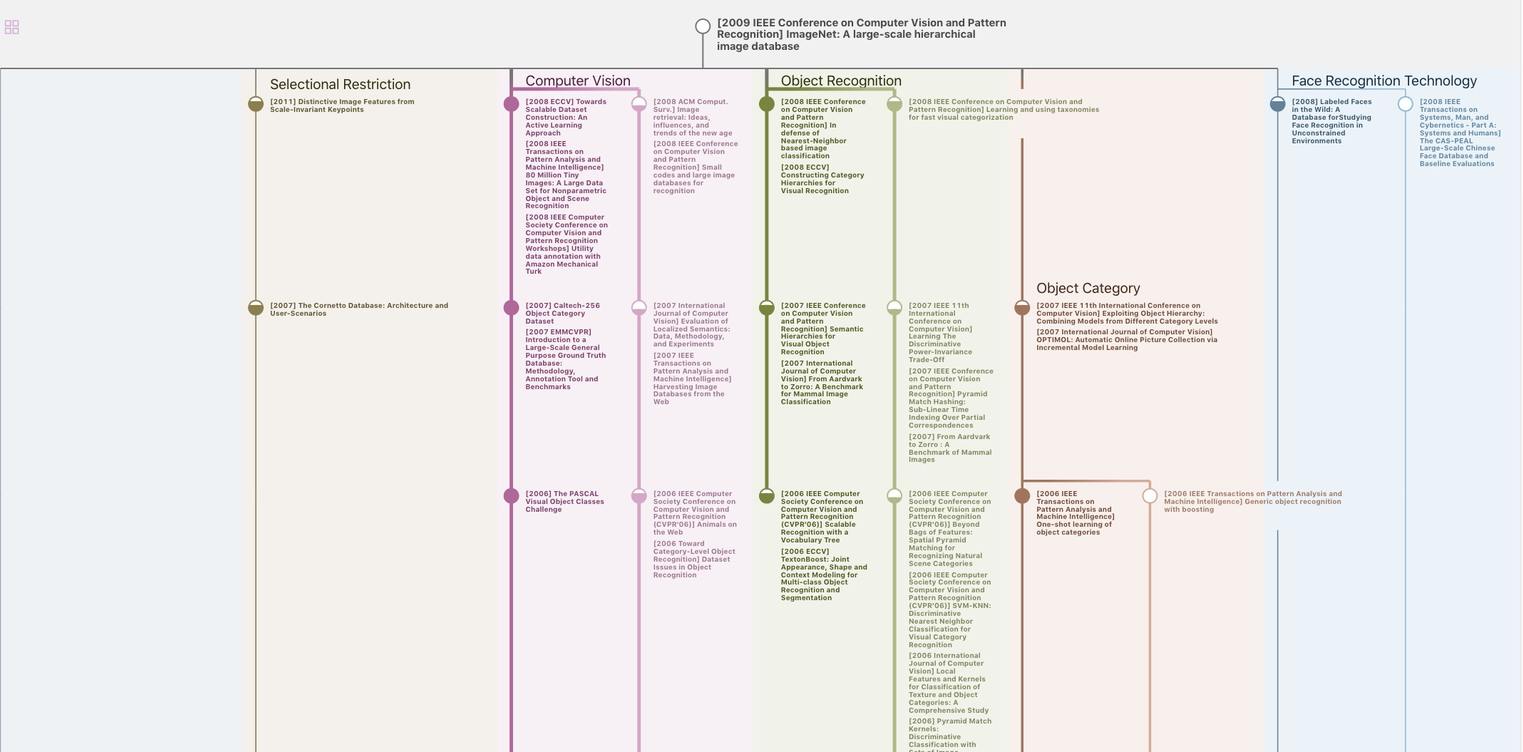
生成溯源树,研究论文发展脉络
Chat Paper
正在生成论文摘要