Monte-Carlo Convolutions on Foveated Images
Proceedings of the 17th International Joint Conference on Computer Vision, Imaging and Computer Graphics Theory and Applications(2022)
摘要
Foveated vision captures a visual scene at space-variant resolution. This makes the application of parameterized convolutions to foveated images difficult as they do not have a dense-grid representation in cartesian space. Log-polar space is frequently used to create a dense grid representation of foveated images, however this image representation may not be appropriate for all applications. In this paper we rephrase the convolution operation as the Monte-Carlo estimation of the filter response of the foveated image and a continuous filter kernel, an idea that has seen frequent use for deep learning on point clouds. We subsume our convolution operation into a simple CNN architecture that processes foveated images in cartesian space. We evaluate our system in the context of image classification and show that our approach significantly outperforms an equivalent CNN processing a foveated image in log-polar space.
更多查看译文
关键词
Foveated, Convolution, Retina, Implict, Neural, Representations
AI 理解论文
溯源树
样例
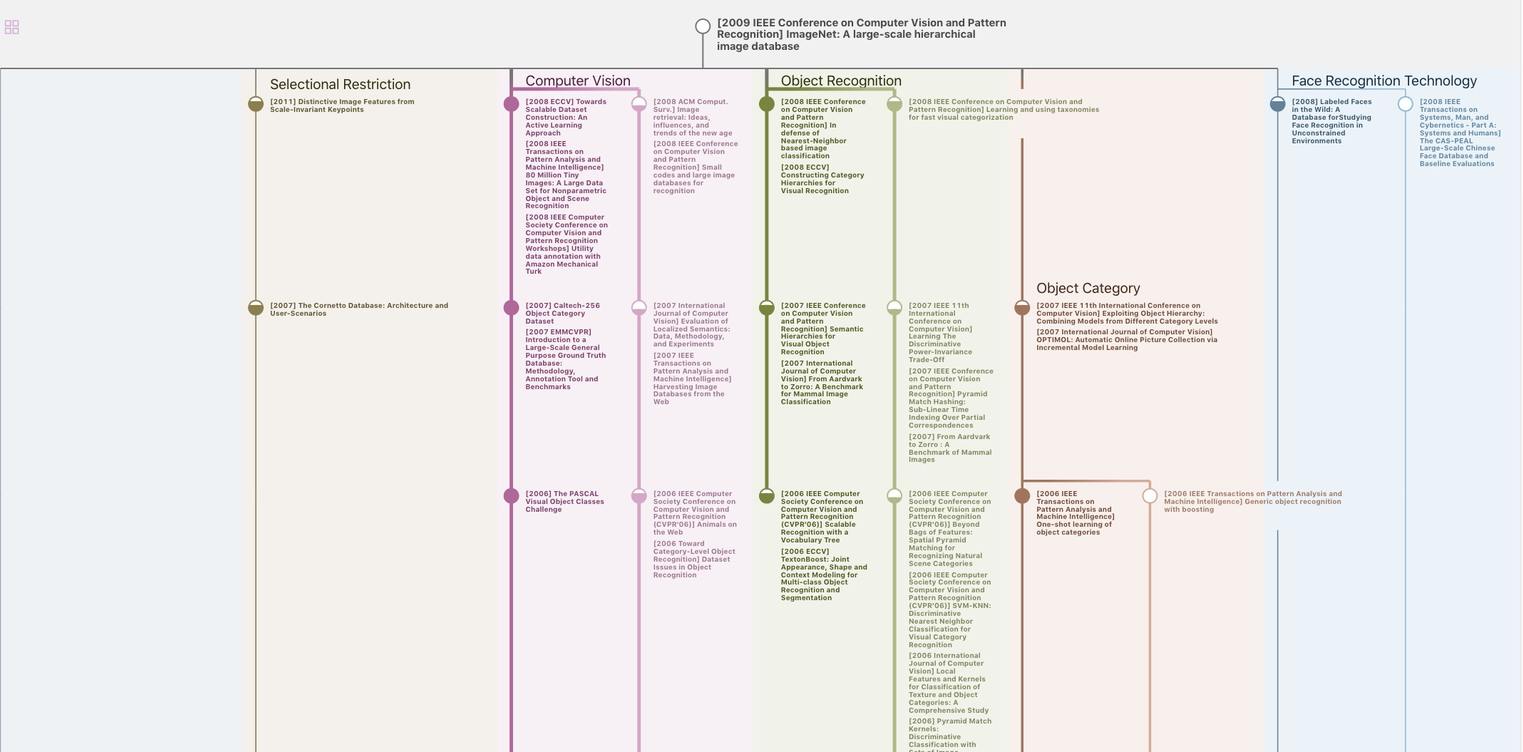
生成溯源树,研究论文发展脉络
Chat Paper
正在生成论文摘要