Improving the adversarial robustness of quantized neural networks via exploiting the feature diversity
PATTERN RECOGNITION LETTERS(2023)
Abstract
Quantized neural networks (QNNs) have become one of the most prevalent approaches in deep learning model compression due to their computational and storage efficiency. However, there is a lack of research specialized in the adversarial robustness of QNNs, which is important for applications in security-critical domains. Existing defenses focus on conventional full-precision networks, which can result in behavioral disparities and degrade the expected performance when directly transferred to QNNs. A novel defensive strateg y promotes featu r e diversity through an orthogonal constraint, which can synergize wel l with quantization. Inspired by this intuition, we propose an orthogonal regularization with quantization to improve the adversarial robustness of QNNs in this paper. Moreover, we observe that quantization serves as an implicit regularization and is able to alleviate orthogonal degeneration. The proposed orthogonal regularization with quantization is validated on several typical network architectures and benchmark datasets. The results demonstrate that the proposed method can notably enhance adversarial robustness against both white-box and black-box attacks.
MoreTranslated text
Key words
Quantized neural networks,Adversarial robustness,Orthogonal regularization,Featu r e diversity
AI Read Science
Must-Reading Tree
Example
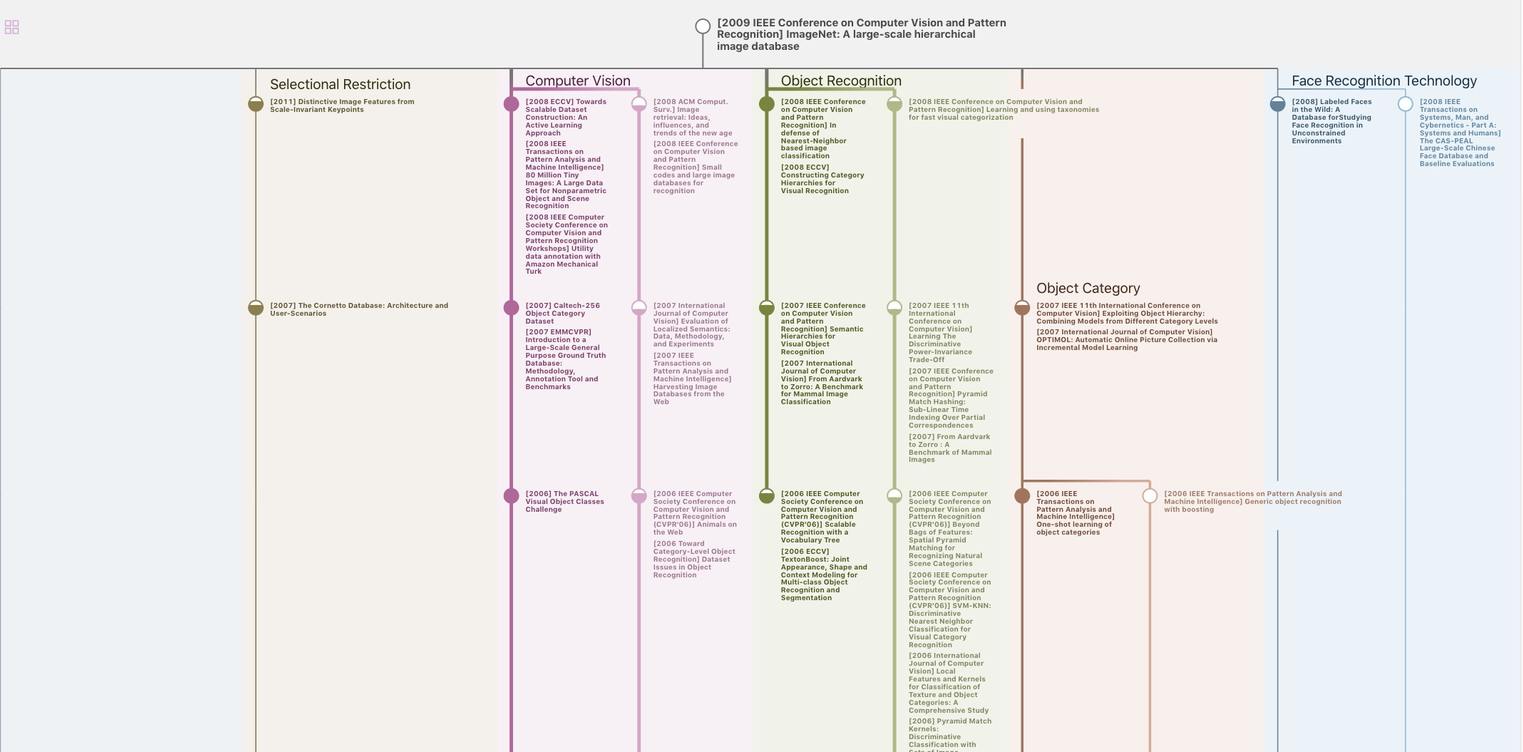
Generate MRT to find the research sequence of this paper
Chat Paper
Summary is being generated by the instructions you defined