A configurable deep learning framework for medical image analysis
Neural Computing and Applications(2022)
摘要
Artificial intelligence-based Medical Image Analysis (AI-MIA) has achieved significant advantages in accuracy and efficiency in various biomedical applications. However, most existing deep learning (DL) models have a fixed network structure and aim at single MIA tasks, making it difficult to meet the diverse and complex MIA requirements. To improve the universality of the AI-MIA DL models, we propose a configurable DL framework, which consists of a MIA task element library and a DL model component library. We establish a MIA task element library to accurately define various potential MIA tasks for numerous diseases. In addition, we collect DL computation modules that can be used in different steps of MIA and built a DL model component library. Given a specific MIA demand of different diseases, the framework can build a personalized DL model by defining MIA tasks and configuring DL model components. Moreover, by comparing with the existing machine learning (ML)/DL models, the structure of the DL model for each configuration can be further adjusted to improve its performance. To demonstrate the effectiveness of the proposed framework, two personalized DL models are designed for upper abdominal organ detection and colon cancer cell classification, achieving high accuracy and high performance. Experimental results on actual medical image datasets show that the configurable DL framework can define specific MIA requirements for different diseases and flexibly configure DL model components to build suitable personalized DL models. Compared with the state-of-the-art methods, the significant advantage of our framework is that it can generate personalized DL models with a flexible structure and can continuously fine-tune the model structure to improve model performance.
更多查看译文
关键词
Artificial intelligence,Configurable framework,Deep learning,Medical image analysis,Personalized model
AI 理解论文
溯源树
样例
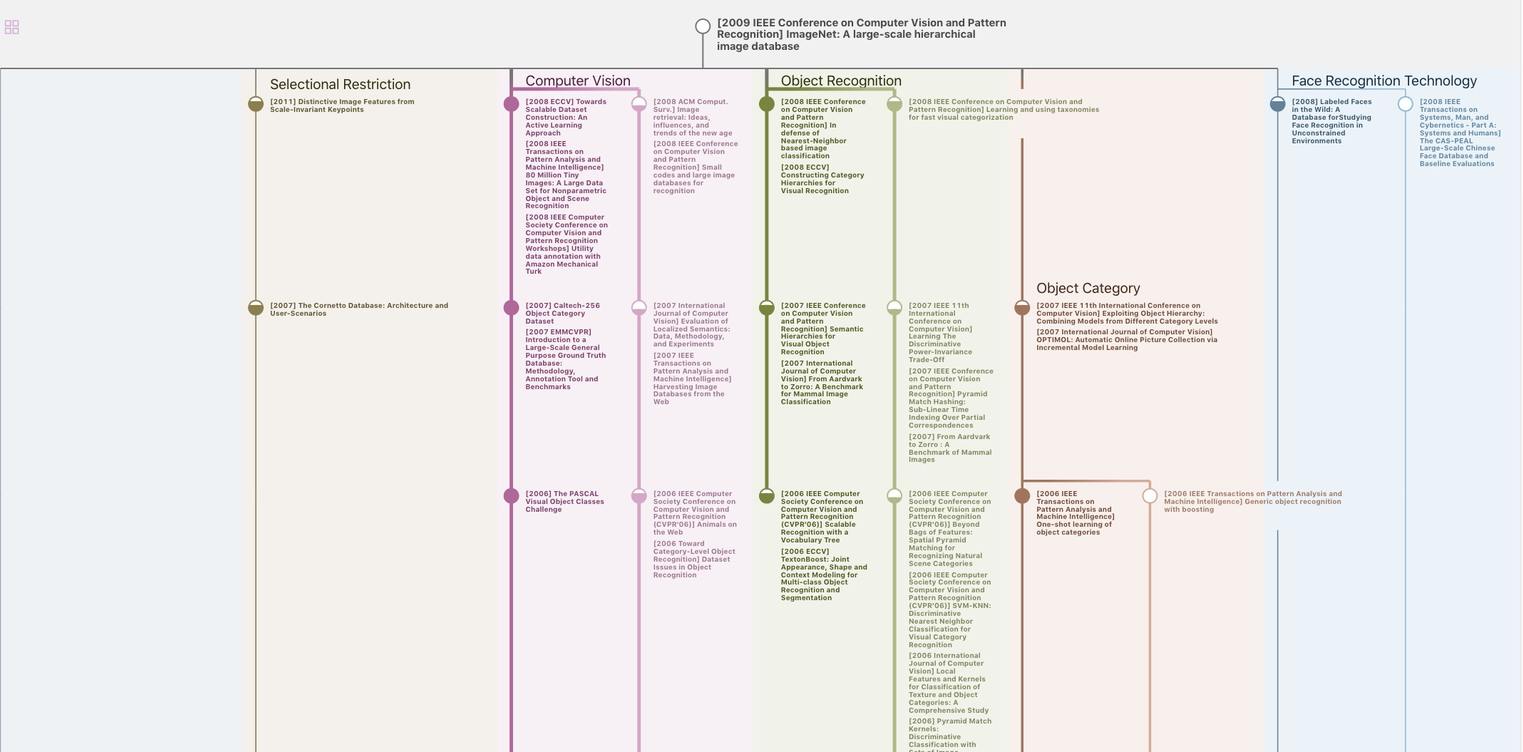
生成溯源树,研究论文发展脉络
Chat Paper
正在生成论文摘要