Sequential Attention-Based Distinct Part Modeling for Balanced Pedestrian Detection
IEEE TRANSACTIONS ON INTELLIGENT TRANSPORTATION SYSTEMS(2022)
摘要
Despite pedestrian detectors having made significant progress by introducing convolutional neural networks, their performance still suffers degradation, especially in occlusion scenes with more false positives (FPs) and false negatives (FNs). To alleviate the problem, we propose a novel Sequential Attention-based Distinct Part Modeling (SA-DPM) for balanced pedestrian detection. It takes one step further in constructing more robust representation that supports detection with fewer FNs and FPs. Specifically, the Sequential Attention serves as one internal perception process that captures several distinct part areas step by step from each pedestrian proposal (full-body). Different from the previous either-or feature selection, the following Joint Learning attempts to seek a reasonable trade-off between part and full-body features, and combines both features for more accurate classification and regression. Evaluation on the widely used pedestrian datasets including Caltech and Citypersons shows that the proposed SA-DPM achieves promising performance for both non-occluded and occluded pedestrian detection tasks, especially on Caltech Heavy Occlusion set, which yields a new state-of-the-art miss rate by 30.18% and outperforms the second best detector by 6.32%.
更多查看译文
关键词
Feature extraction,Detectors,Proposals,Task analysis,Sun,Intelligent transportation systems,Annotations,Pedestrian detection,occlusion handling,part attention
AI 理解论文
溯源树
样例
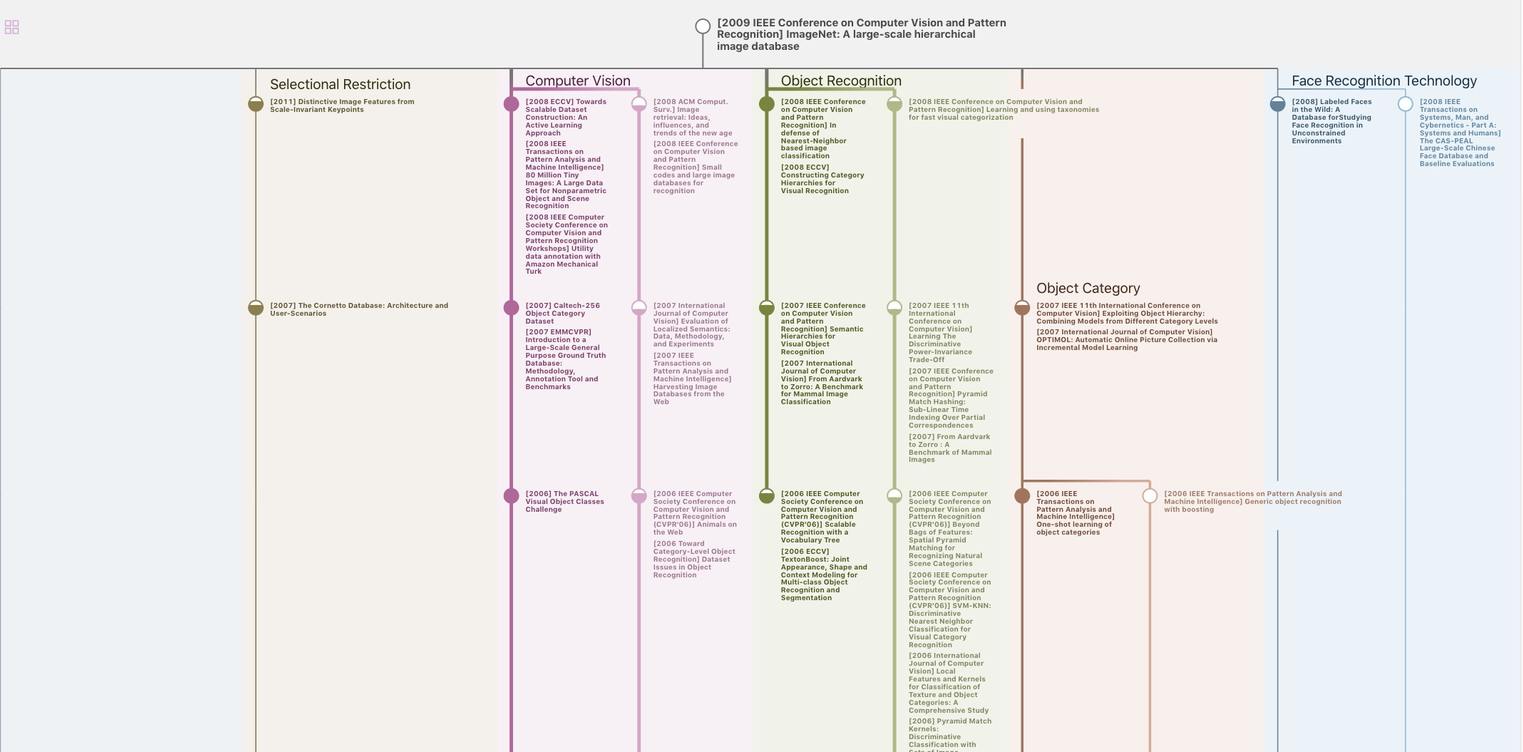
生成溯源树,研究论文发展脉络
Chat Paper
正在生成论文摘要