Prediction of IIoT traffic using a modified whale optimization approach integrated with random forest classifier
The Journal of Supercomputing(2022)
摘要
Several organizations are implementing large-scale Internet of Things (IoT)-based ecosystems, such as Industrial IoT (IIoT). Such systems are vulnerable to new threats and intrusions because of the nature of their networks. It is necessary to secure such systems by developing feature selection integrated with robust machine learning models. In this paper, a two-phase IIoT traffic prediction model is built for detecting normal and anomalous IIoT behavior. The system is divided into two phases. In the first phase, multi-objective non-dominated sorting with whale optimization approach (MNSWOA) and ideal point method (IPM) is implemented for feature selection. The key quality attributes are retrieved for effective classification. In the second phase, the feature selection results are sent to the random forest (RF) classifier for traffic prediction. Experimental results on IIoT datasets, namely CTU-13, AWID and Gas–Water show that the proposed technique MNSWOA–IPM–RF obtains enhanced performance in terms of accuracy, true positive rate, true negative rate and area under the ROC curve. Also, comparisons indicate that the proposed MNSWOA–IPM–RF model has superior results than benchmark multi-objective optimization techniques.
更多查看译文
关键词
Accuracy,Feature selection,Multi-objective,Evolutionary computation,Random forest,Whale optimization
AI 理解论文
溯源树
样例
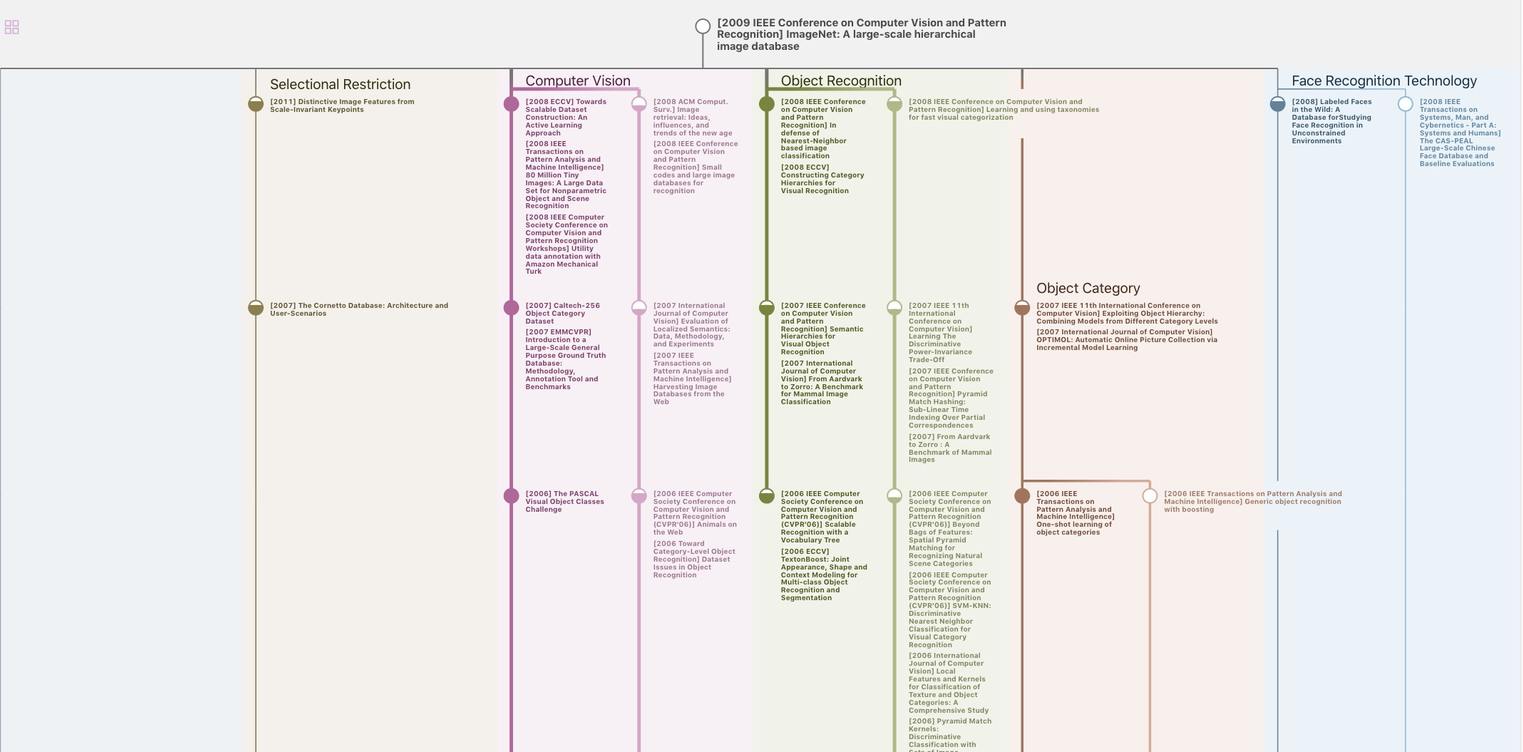
生成溯源树,研究论文发展脉络
Chat Paper
正在生成论文摘要