ConWST: Non-native Multi-source Knowledge Distillation for Low Resource Speech Translation
Communications in Computer and Information ScienceCognitive Systems and Information Processing(2022)
摘要
In the absence of source speech information, most end-to-end speech translation (ST) models showed unsatisfactory results. So, for low-resource non-native speech translation, we propose a self-supervised bidirectional distillation processing system. It improves speech ST performance by using a large amount of untagged speech and text in a complementary way without adding source information. The framework is based on an attentional Sq2sq model, which uses wav2vec2.0 pre-training to guide the Conformer encoder for reconstructing the acoustic representation. The decoder generates a target token by fusing the out-of-domain embeddings. We investigate the use of Byte pair encoding (BPE) and compare it with several fusion techniques. Under the framework of ConWST, we conducted experiments on language transcription from Swahili to English. The experimental results show that the transcription under the framework has a better performance than the baseline model, which seems to be one of the best transcriptional methods.
更多查看译文
关键词
End-to-End ST,wav2vec2.0,Low resource,Self-supervised
AI 理解论文
溯源树
样例
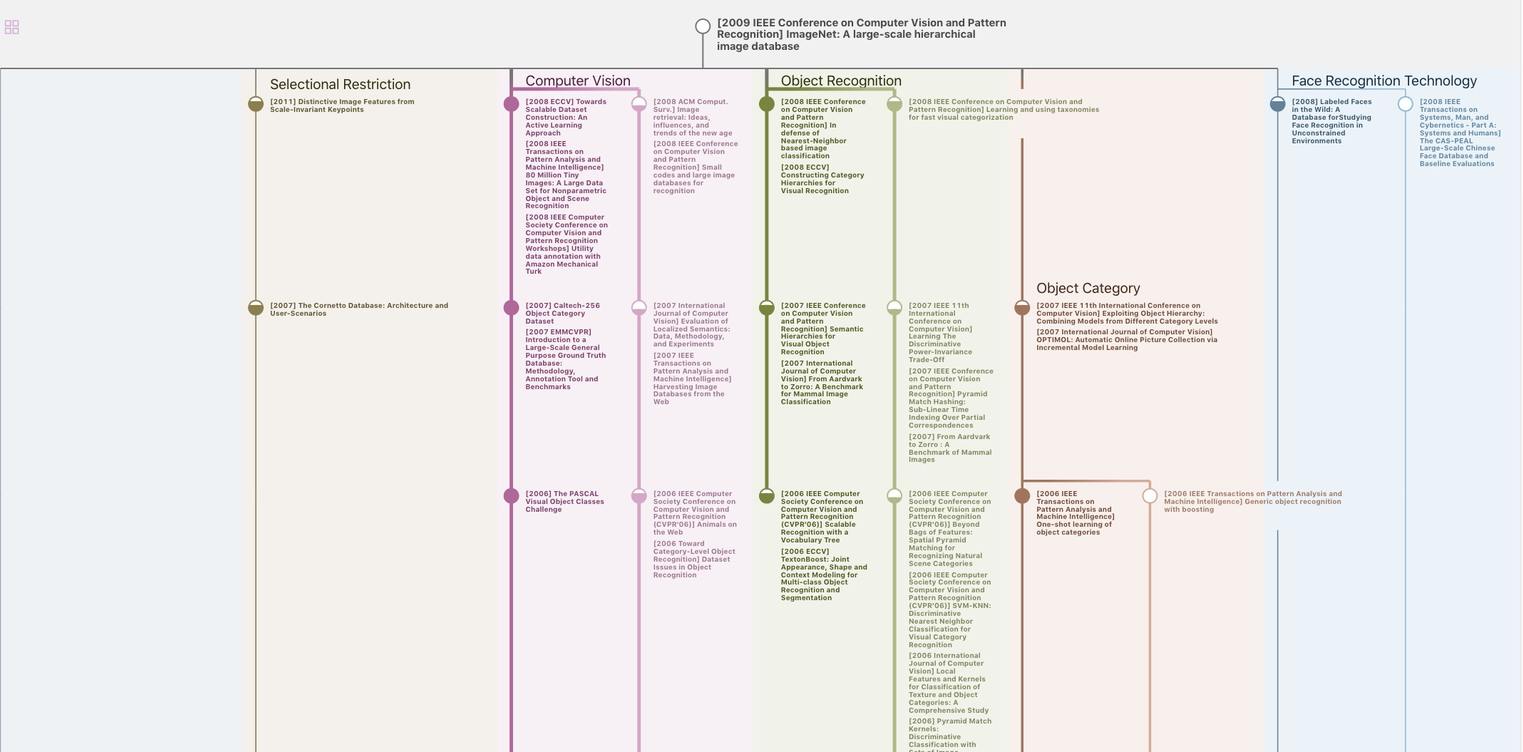
生成溯源树,研究论文发展脉络
Chat Paper
正在生成论文摘要