Machine-guided exploration and calibration of astrophysical simulations (vol 515, pg 693, 2022)
Monthly Notices of the Royal Astronomical Society(2023)
摘要
We apply a novel method with machine learning to calibrate sub-grid models within numerical simulation codes to achieve convergence with observations and between different codes. It utilizes active learning and neural density estimators. The hyper parameters of the machine are calibrated with a well-defined projectile motion problem. Then, using a set of 22 cosmological zoom simulations, we tune the parameters of a popular star formation and feedback model within Enzo to match observations. The parameters that are adjusted include the star formation efficiency, coupling of thermal energy from stellar feedback, and volume into which the energy is deposited. This number translates to a factor of more than three improvements over manual calibration. Despite using fewer simulations, we obtain a better agreement to the observed baryon makeup of a Milky Way (MW)-sized halo. Switching to a different strategy, we improve the consistency of the recommended parameters from the machine. Given the success of the calibration, we then apply the technique to reconcile metal transport between grid-based and particle-based simulation codes using an isolated galaxy. It is an improvement over manual exploration while hinting at a less-known relation between the diffusion coefficient and the metal mass in the halo region. The exploration and calibration of the parameters of the sub-grid models with a machine learning approach is concluded to be versatile and directly applicable to different problems.
更多查看译文
关键词
galaxies: evolution,galaxies: formation,galaxies: haloes
AI 理解论文
溯源树
样例
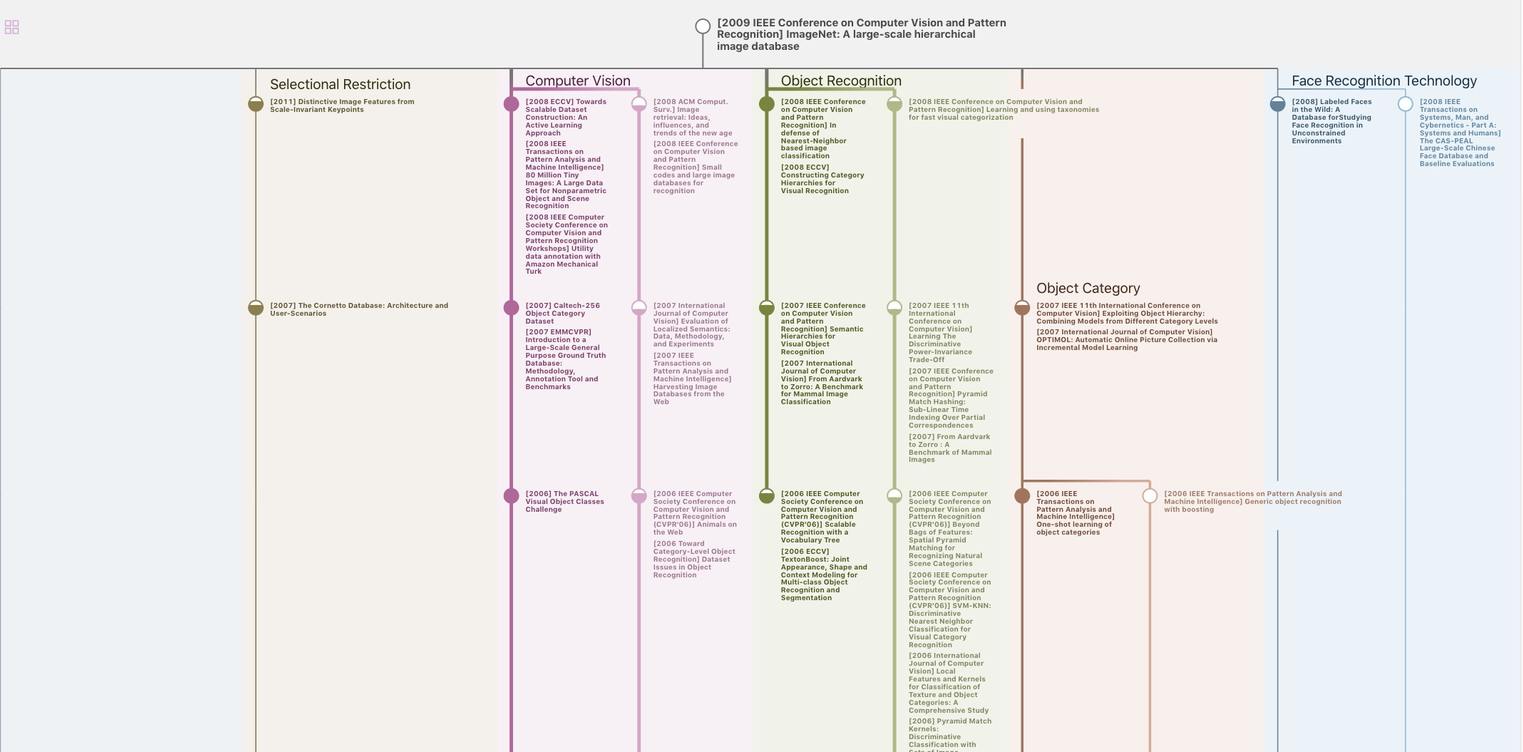
生成溯源树,研究论文发展脉络
Chat Paper
正在生成论文摘要