Adaptive Model Predictive Control by Learning Classifiers.
Conference on Learning for Dynamics & Control (L4DC)(2022)
摘要
Stochastic model predictive control has been a successful and robust control framework for many robotics tasks where the system dynamics model is slightly inaccurate or in the presence of environment disturbances. Despite the successes, it is still unclear how to best adjust control parameters to the current task in the presence of model parameter uncertainty and heteroscedastic noise. In this paper, we propose an adaptive MPC variant that automatically estimates control and model parameters by leveraging ideas from Bayesian optimization (BO) and the classical expected improvement acquisition function. We leverage recent results showing that BO can be formulated as a density ratio estimation which can be efficiently approximated by simply learning a classifier. This is then integrated into a model predictive path integral control framework yielding robust controllers for a variety of challenging robotics tasks. We demonstrate the approach on classical control problems under model uncertainty and robotics manipulation tasks.
更多查看译文
关键词
model predictive control,learning
AI 理解论文
溯源树
样例
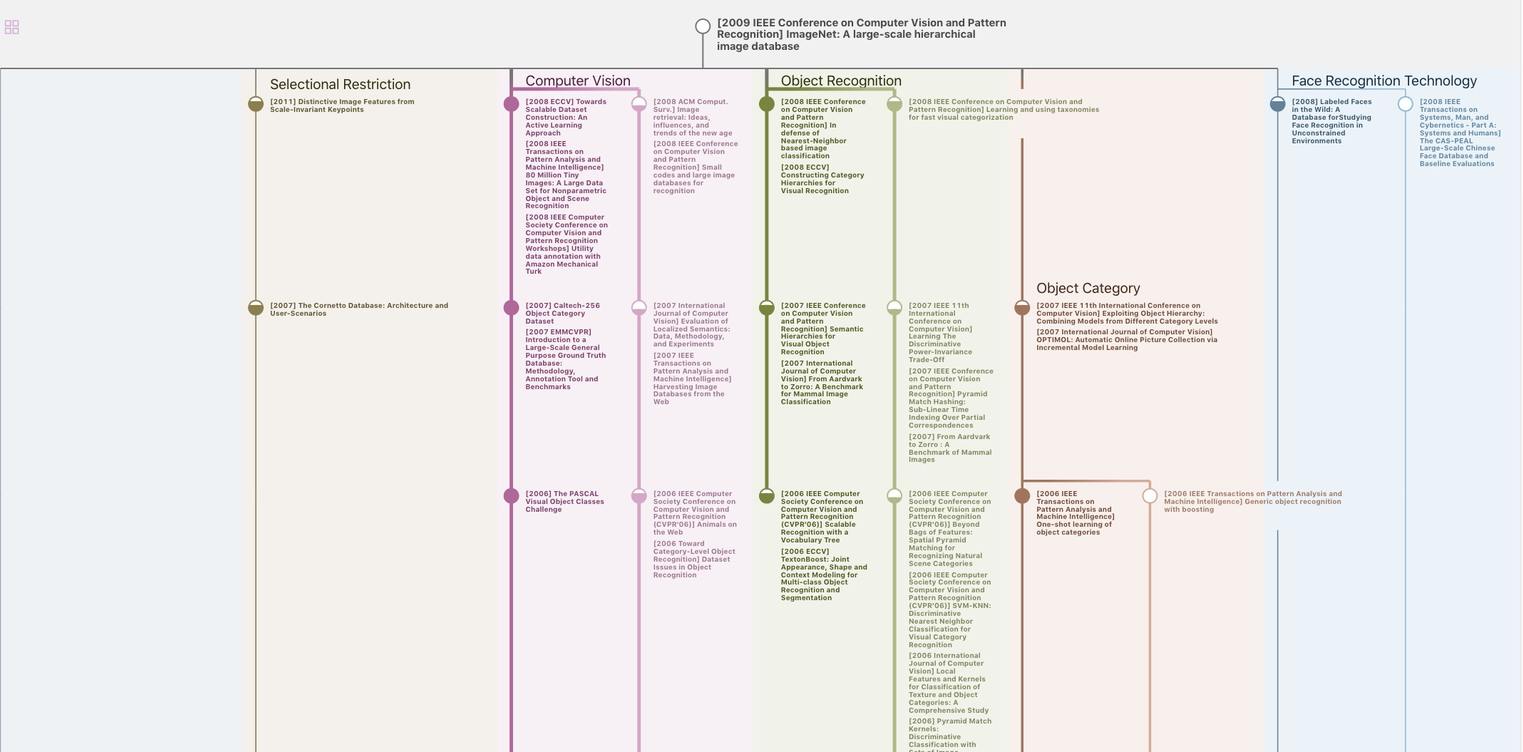
生成溯源树,研究论文发展脉络
Chat Paper
正在生成论文摘要