Deformable VisTR: Spatio Temporal Deformable Attention for Video Instance Segmentation.
IEEE International Conference on Acoustics, Speech, and Signal Processing (ICASSP)(2022)
摘要
Video instance segmentation (VIS) task requires classifying, segmenting, and tracking object instances over all frames in a video clip. Recently, VisTR has been proposed as end-to-end transformer-based VIS framework, while demonstrating state-of-the-art performance. However, VisTR is slow to converge during training, requiring around 1000 GPU hours due to the high computational cost of its transformer attention module. To improve the training efficiency, we propose Deformable VisTR, leveraging spatio-temporal deformable attention module that only attends to a small fixed set of key spatio-temporal sampling points around a reference point. This enables Deformable VisTR to achieve linear computation in the size of spatio-temporal feature maps. Moreover, it can achieve on par performance as the original VisTR with 10$\times$ less GPU training hours. We validate the effectiveness of our method on the Youtube-VIS benchmark. Code is available at https://github.com/skrya/DefVIS.
更多查看译文
关键词
video instance segmentation,deformable convolution,efficient framework
AI 理解论文
溯源树
样例
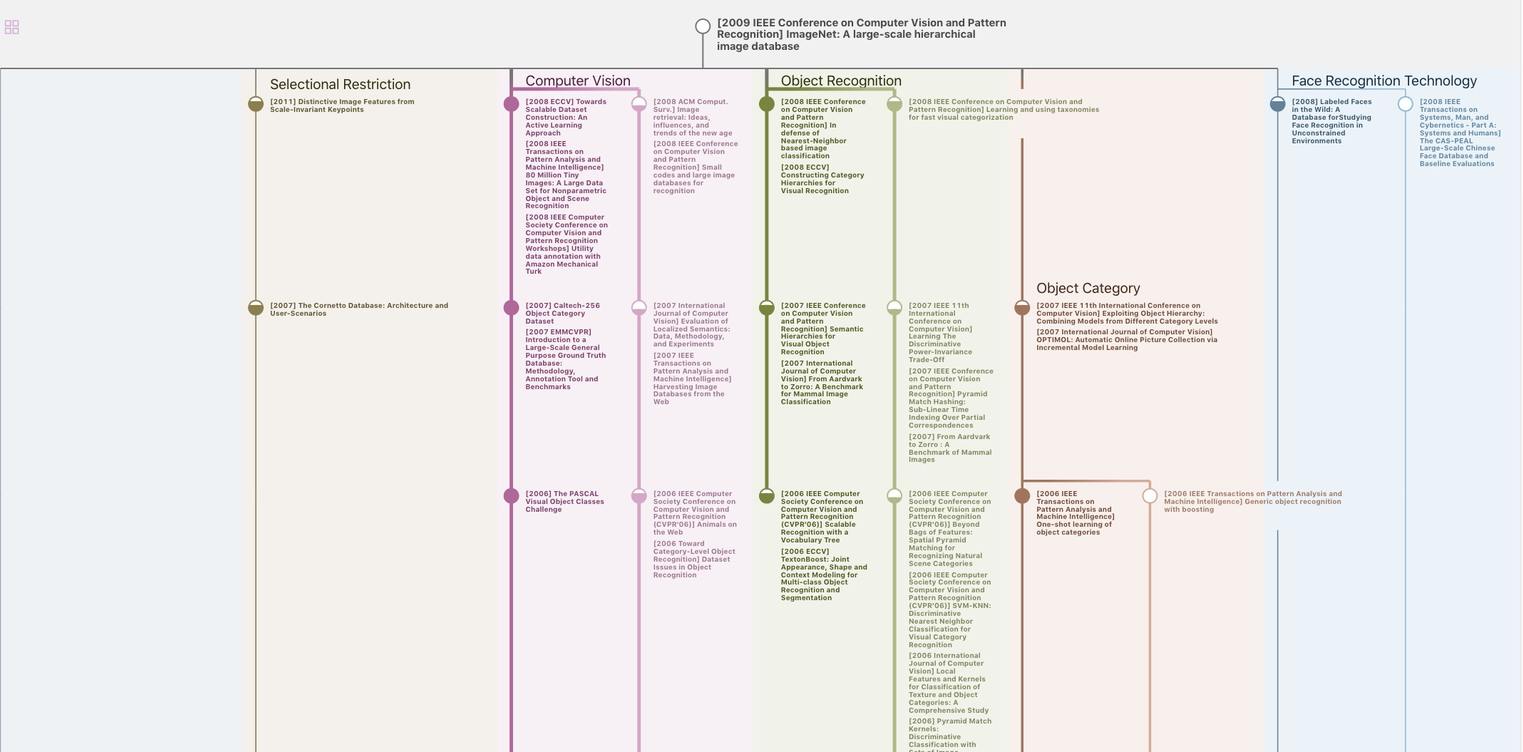
生成溯源树,研究论文发展脉络
Chat Paper
正在生成论文摘要