On the role of data in PAC-Bayes bounds
24TH INTERNATIONAL CONFERENCE ON ARTIFICIAL INTELLIGENCE AND STATISTICS (AISTATS)(2021)
摘要
The dominant term in PAC-Bayes bounds is often the Kullback-Leibler divergence between the posterior and prior. For so-called linear PAC-Bayes risk bounds based on the empirical risk of a fixed posterior kernel, it is possible to minimize the expected value of the bound by choosing the prior to be the expected posterior, which we call the oracle prior on the account that it is distribution dependent. In this work, we show that the bound based on the oracle prior can be suboptimal: In some cases, a stronger bound is obtained by using a data-dependent oracle prior, i.e., a conditional expectation of the posterior, given a subset of the training data that is then excluded from the empirical risk term. While using data to learn a prior is a known heuristic, its essential role in optimal bounds is new. In fact, we show that using data can mean the difference between vacuous and nonvacuous bounds. We apply this new principle in the setting of nonconvex learning, simulating data-dependent oracle priors on MNIST and Fashion MNIST with and without held-out data, and demonstrating new nonvacuous bounds in both cases.
更多查看译文
关键词
bounds,data,pac-bayes
AI 理解论文
溯源树
样例
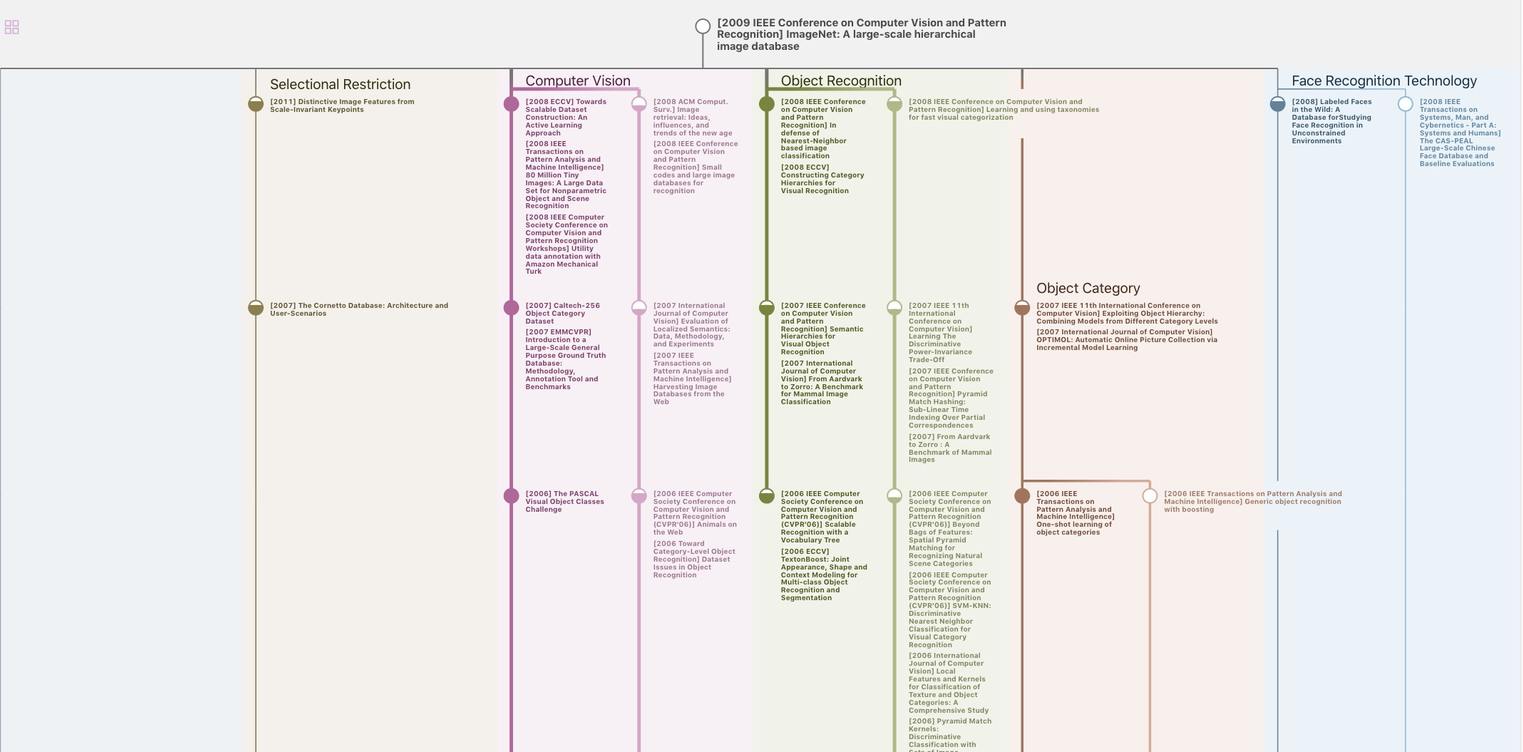
生成溯源树,研究论文发展脉络
Chat Paper
正在生成论文摘要