A Hybrid Approach to Histopathological Diagnosis of Paratuberculosis in Sheep with Image Classification and Explainable Artificial Intelligence with Grad-CAM
SSRN Electronic Journal(2022)
摘要
Abstract Paratuberculosis is a chronic, progressive, and economically important disease. The diagnosis of the disease, especially in the early stage, is difficult. Deep learning is an emerging method in the histopathological diagnosis of images. Deep Learning is the system that can perform automatic feature extraction in training sets containing tagged images and that enables the use of multi-layered neural networks, unlike machine learning. In this article, deep learning methods were used for the diagnosis of paratuberculosis in sheep’s gut images. Normal intestinal histological images were used as control data. This study revealed that the deep learning method can be used with high accuracy for the histopathological diagnosis of paratuberculosis. According to Deep Learning models, the highest accuracy is proposed model Fastai/VGG-16 which has Classification Accuracy 98,36 not only this one but also the Matthews Correlation Coefficient (MCC) is 0,967. According to classification accuracy, Gradient-weighted Class Activation Mapping (Grad-CAM) was used to better understand the explainability and safety level of the model. The aim of this study, build a model that was developed to help pathologists diagnose paratuberculosis disease by transferring the diagnostic information of pathologists to deep learning models and also present an explainable model for paratuberculosis, an image-based diagnostic problem in which deep learning processes can be applied effectively.
更多查看译文
关键词
paratuberculosis,histopathological diagnosis,sheep,image classification,grad-cam
AI 理解论文
溯源树
样例
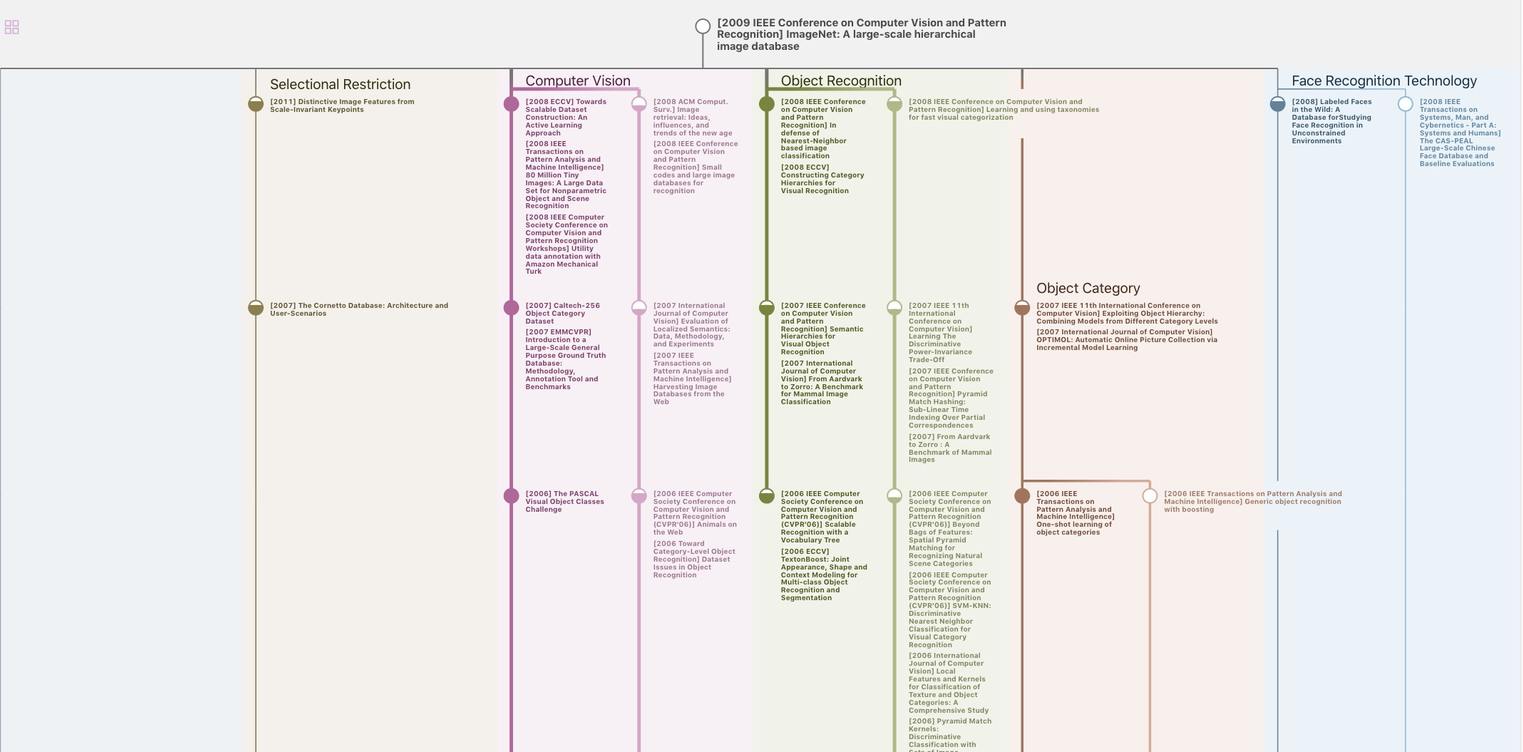
生成溯源树,研究论文发展脉络
Chat Paper
正在生成论文摘要