Forest Disturbance Detection with Seasonal and Trend Model Components and Machine Learning Algorithms
REMOTE SENSING(2022)
摘要
Forest disturbances reduce the extent of natural habitats, biodiversity, and carbon sequestered in forests. With the implementation of the international framework Reduce Emissions from Deforestation and forest Degradation (REDD+), it is important to improve the accuracy in the estimation of the extent of forest disturbances. Time series analyses, such as Breaks for Additive Season and Trend (BFAST), have been frequently used to map tropical forest disturbances with promising results. Previous studies suggest that in addition to magnitude of change, disturbance accuracy could be enhanced by using other components of BFAST that describe additional aspects of the model, such as its goodness-of-fit, NDVI seasonal variation, temporal trend, historical length of observations and data quality, as well as by using separate thresholds for distinct forest types. The objective of this study is to determine if the BFAST algorithm can benefit from using these model components in a supervised scheme to improve the accuracy to detect forest disturbance. A random forests and support vector machines algorithms were trained and verified using 238 points in three different datasets: all-forest, tropical dry forest, and temperate forest. The results show that the highest accuracy was achieved by the support vector machines algorithm using the all-forest dataset. Although the increase in accuracy of the latter model vs. a magnitude threshold model is small, i.e., 0.14% for sample-based accuracy and 0.71% for area-weighted accuracy, the standard error of the estimated total disturbed forest area was 4352.59 ha smaller, while the annual disturbance rate was also smaller by 1262.2 ha year(-1). The implemented approach can be useful to obtain more precise estimates in forest disturbance, as well as its associated carbon emissions.
更多查看译文
关键词
random forests,support vector machines,tropical dry forest,forest cover change,time series analyses,Breaks for Additive Season and Trend (BFAST)
AI 理解论文
溯源树
样例
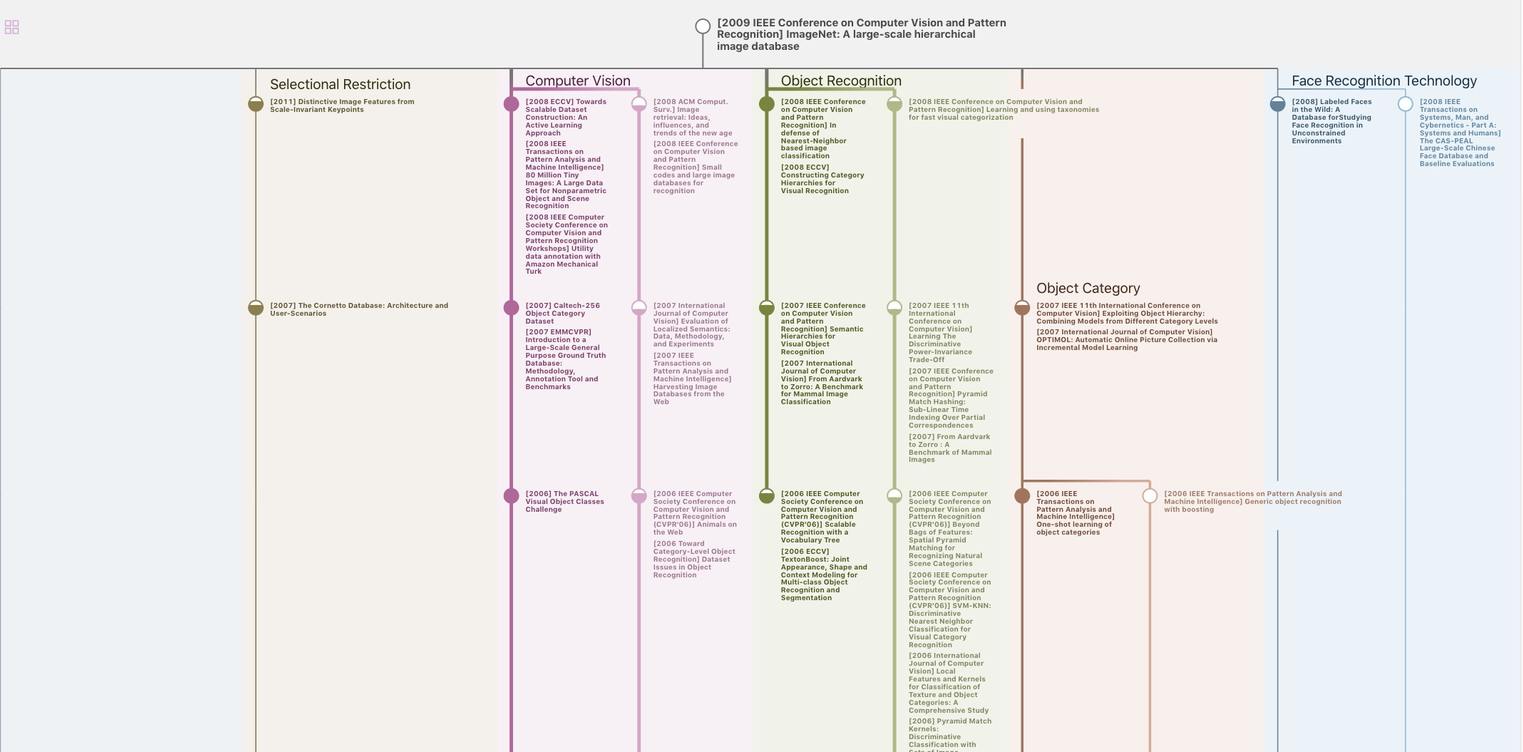
生成溯源树,研究论文发展脉络
Chat Paper
正在生成论文摘要