FCNet: A feature context network based on ensemble framework for image retrieval
IET COMPUTER VISION(2022)
摘要
In order to train an end-to-end model for better performance in distinguishing superclasses and make the model more sensitive to inter-class differences, the authors propose a Feature Context Network (FCNet), a novel ensemble framework for image retrieval based on clustering (EFC) and metric learning. In the authors' approach, the EFC framework consists of one common feature extractor and multiple learning branches. This tree-like structure not only promotes the stability of the training process but also keeps the diversity by using multiple branches. Each branch is learnt by an independent random re-labelling operation that distributes original classes into superclasses. In such a way, the framework thus can accelerate the training process. To make the EFC framework focus on the different attributes across the classes, the authors utilize k-means clustering to divide the training set into subsets and train the model sequentially with those subsets. Meanwhile, to reduce the impact of over-fitting problems, the authors design a branch weight structure during training. Further, FCNet fusions different scales of the neighbourhood information and consequently makes the feature map learn richer information with the different receptive fields. Simultaneously, it can reduce the layer of the network and the number of model parameters. Extensive experiments demonstrate that this approach outperforms the tested representative methods on CARS-196, CUB-200-2011 datasets.
更多查看译文
关键词
computer vision,convolution,image retrieval,learning (artificial intelligence)
AI 理解论文
溯源树
样例
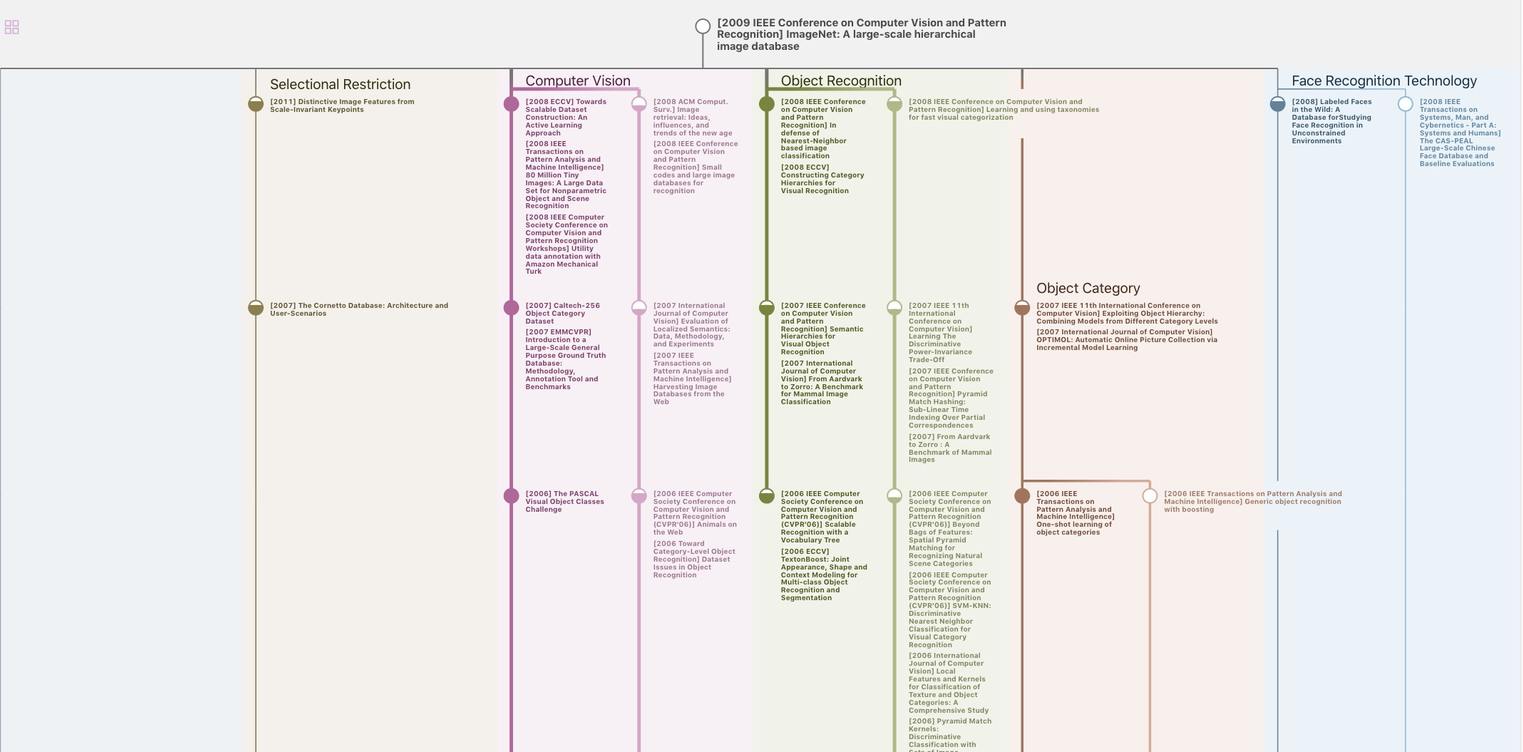
生成溯源树,研究论文发展脉络
Chat Paper
正在生成论文摘要