Semantic Segmentation of Metoceanic Processes Using SAR Observations and Deep Learning
REMOTE SENSING(2022)
摘要
Through the Synthetic Aperture Radar (SAR) embarked on the satellites Sentinel-1A and Sentinel-1B of the Copernicus program, a large quantity of observations is routinely acquired over the oceans. A wide range of features from both oceanic (e.g., biological slicks, icebergs, etc.) and meteorologic origin (e.g., rain cells, wind streaks, etc.) are distinguishable on these acquisitions. This paper studies the semantic segmentation of ten metoceanic processes either in the context of a large quantity of image-level groundtruths (i.e., weakly-supervised framework) or of scarce pixel-level groundtruths (i.e., fully-supervised framework). Our main result is that a fully-supervised model outperforms any tested weakly-supervised algorithm. Adding more segmentation examples in the training set would further increase the precision of the predictions. Trained on 20 x 20 km imagettes acquired from the WV acquisition mode of the Sentinel-1 mission, the model is shown to generalize, under some assumptions, to wide-swath SAR data, which further extents its application domain to coastal areas.
更多查看译文
关键词
SAR,segmentation,metocean,deep learning,supervised learning,weakly-supervised learning,Sentinel-1
AI 理解论文
溯源树
样例
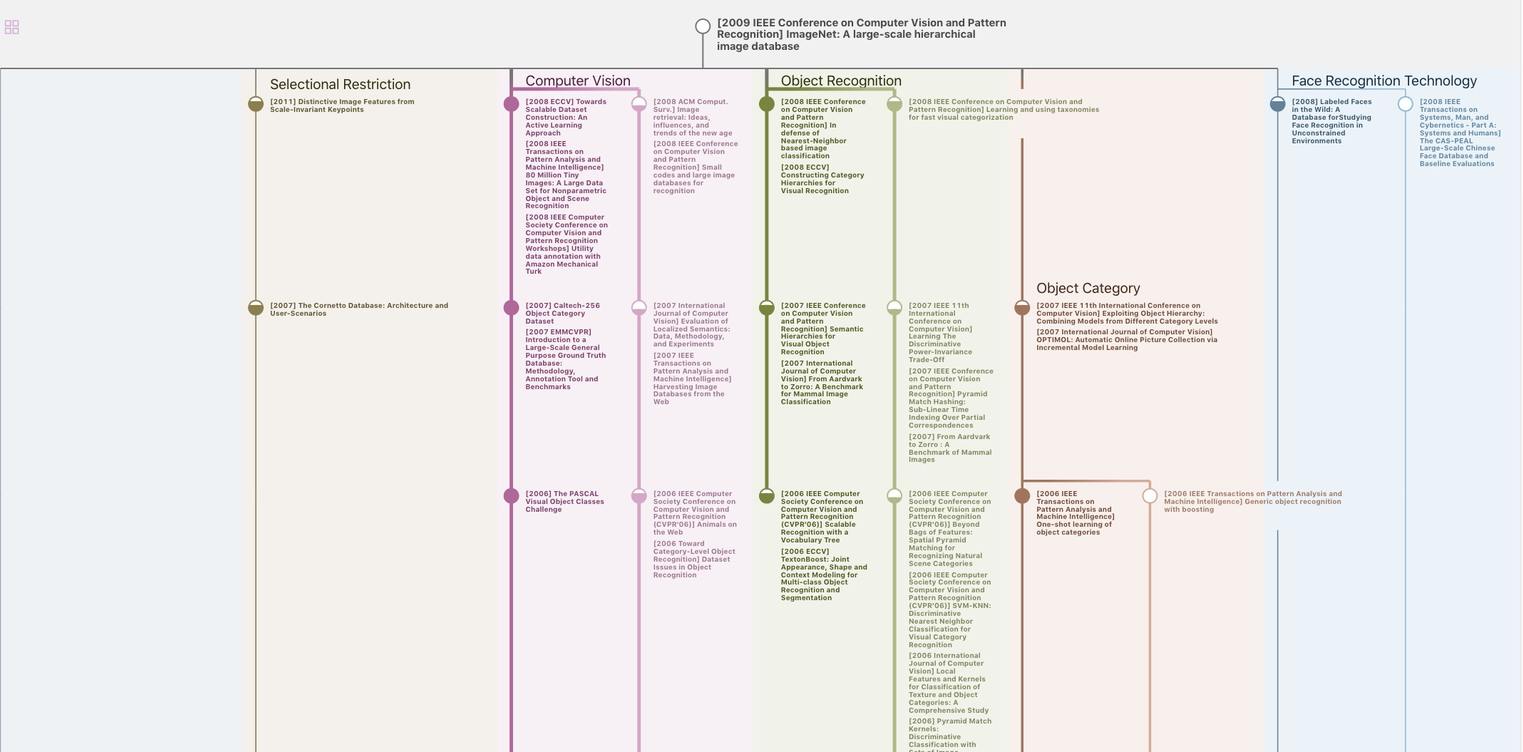
生成溯源树,研究论文发展脉络
Chat Paper
正在生成论文摘要