Non-parametric Bayesian updating and windowing with kernel density and the kudzu algorithm
International Journal of Computational Economics and Econometrics(2022)
摘要
The concept of 'updating' parameter estimates and predictions as more data arrive is an important attraction for people adopting Bayesian methods, and essential in big data settings. Implementation via the hyperparameters of a joint prior distribution is challenging. This paper considers non-parametric updating, using a previous posterior sample as a new prior sample. Streaming data can be analysed in a moving window of time by subtracting old posterior sample(s) with appropriate weights. We evaluate three forms of kernel density, a sampling importance resampling implementation, and a novel algorithm called kudzu, which smooths density estimation trees. Methods are tested for distortion of illustrative prior distributions, long-run performance in a low-dimensional simulation study, and feasibility with a realistically large and fast dataset of taxi journeys. Kernel estimation appears to be useful in low-dimensional problems, and kudzu in high-dimensional problems, but careful tuning and monitoring is required. Areas for further research are outlined.
更多查看译文
关键词
Bayesian data analysis, big data, density estimation trees, kernel density estimation, non-parametric statistics, streaming data
AI 理解论文
溯源树
样例
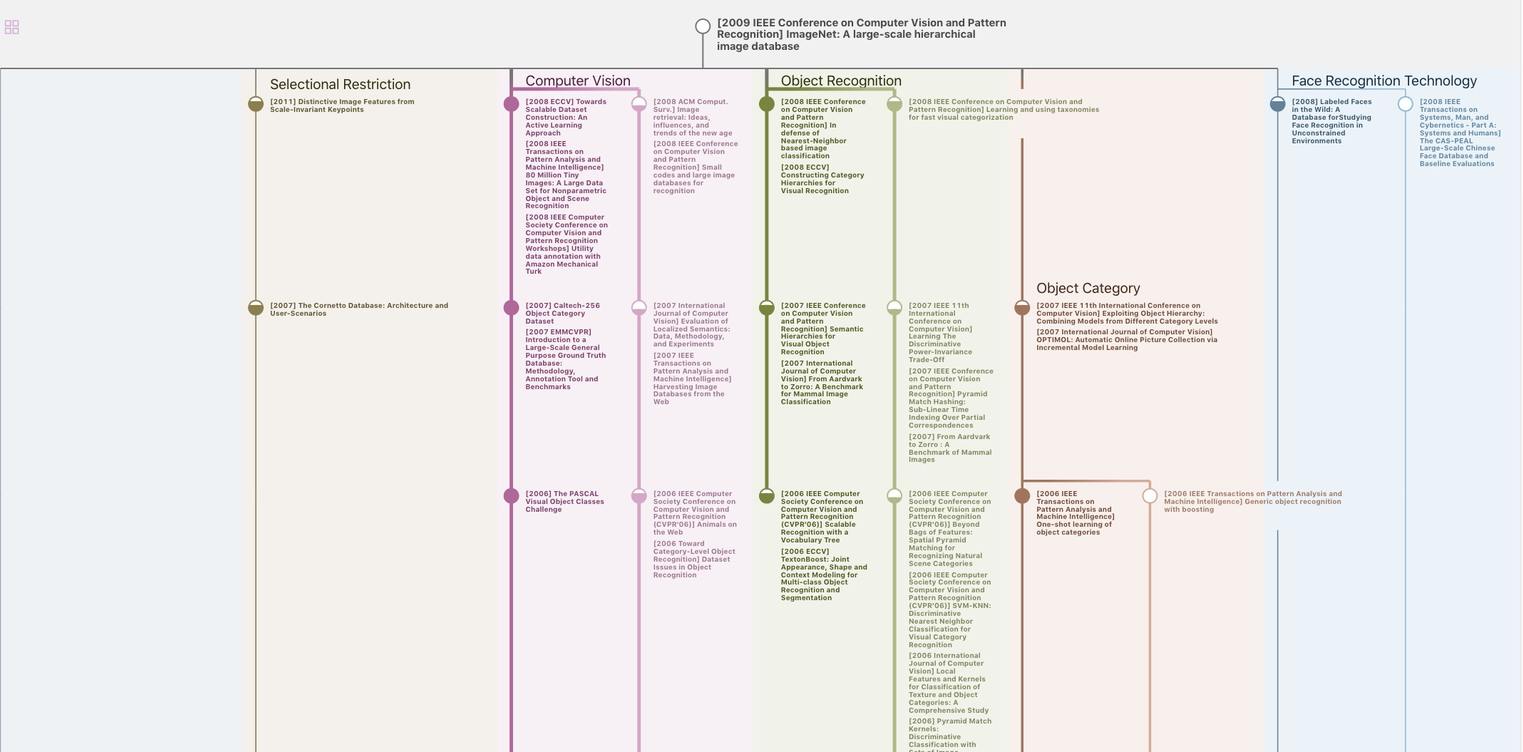
生成溯源树,研究论文发展脉络
Chat Paper
正在生成论文摘要