L 2 error estimates for polynomial discrete penalized least-squares approximation on the sphere from noisy data
Journal of Computational and Applied Mathematics(2022)
摘要
We consider discrete penalized least-squares approximation on the unit sphere S d, where the minimizer is sought in the space P L ( S d ) of spherical polynomials of degree ≤ L. The penalized least-squares functional is the sum of two terms both involving the weights and the nodes of a positive weight quadrature rule with polynomial degree of exactness at least 2 L. The first one is a discrete least-squares functional measuring the squared weighted ℓ 2 discrepancy between the noisy data and the approximation. The second term is the product of a regularization parameter λ ≥ 0 times a penalization term that can be interpreted as an approximation of a squared semi-norm in the Sobolev (Hilbert) space H s ( S d ). The approximation can be computed directly via a summation process and does not require the solving of a linear system. For λ = 0 (which is only appropriate if there is almost no noise), our approximation becomes a case of hyperinterpolation. As λ > 0 increases, less weight is given to data fitting and more weight is given to keeping the approximation smooth. We derive L 2 ( S d ) error estimates for the approximation of functions from the Sobolev Hilbert space H s ( S d ), where s > d / 2, from noisy data for the regularization parameter λ chosen (i) as λ = 0 (hyperinterpolation), (ii) for general λ > 0, and (iii) with Morozov’s discrepancy principle (an a posteriori parameter choice strategy). The L 2 ( S d ) error estimates in case (i) and (iii) are in a sense order-optimal. Numerical experiments explore the approximation method and illustrate the theoretical results.
更多查看译文
关键词
primary,secondary,L 2 error estimates,Morozov’s discrepancy principle,Penalized least-squares approximation,Positive weight quadrature rules,Sphere,Spherical polynomials
AI 理解论文
溯源树
样例
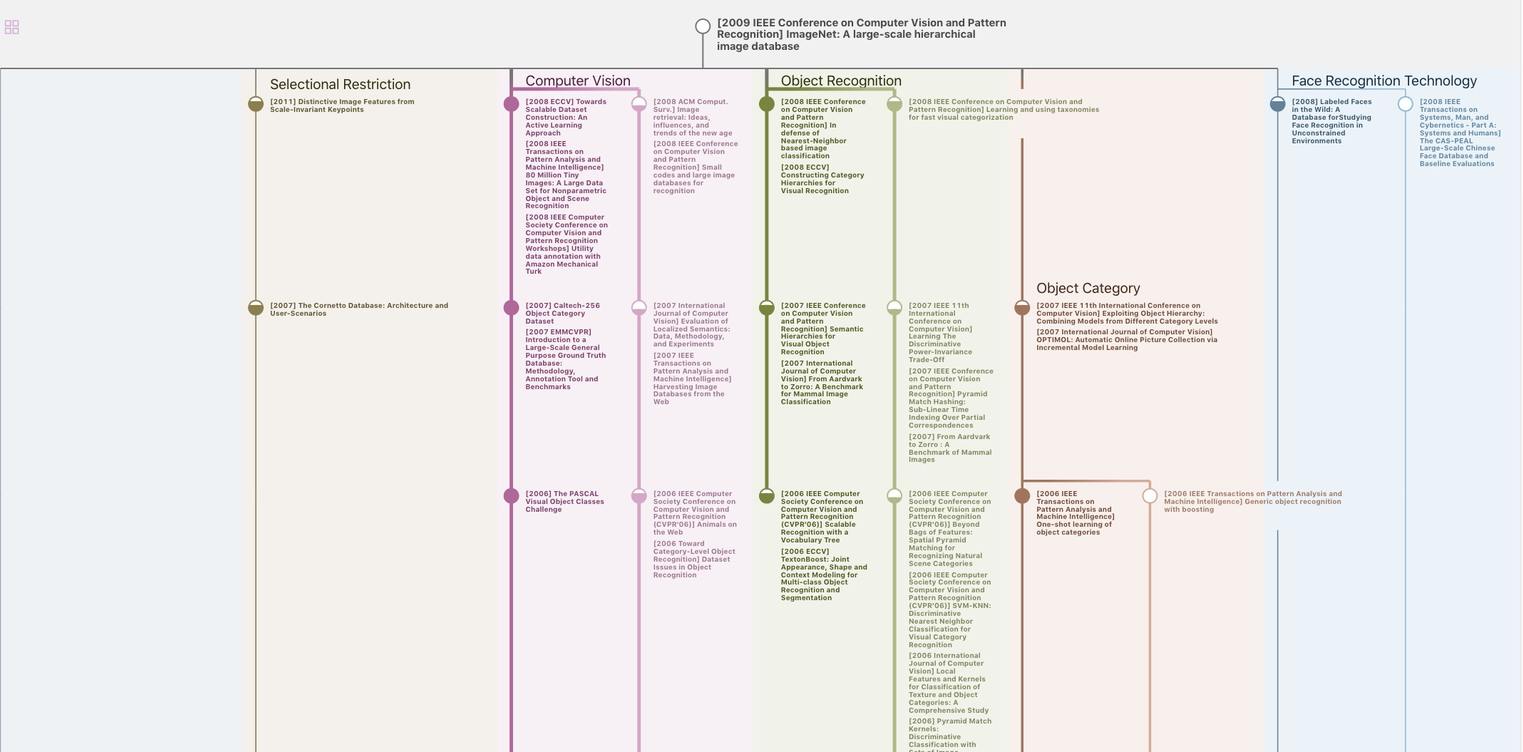
生成溯源树,研究论文发展脉络
Chat Paper
正在生成论文摘要