Comparative Evaluation of Machine Learning Inference Machines on Edge-class Devices
25th Pan-Hellenic Conference on Informatics(2021)
摘要
Computer science and engineering have evolved rapidly over the last decade offering innovative Machine Learning frameworks and high-performance hardware devices. Executing data analytics at the edge promises to transform the mobile computing paradigm by bringing intelligence next to the end user. However, it remains an open question to explore if, and to what extent, today's Edgeclass devices can support ML frameworks and which is the best configuration for efficient task execution. This paper provides a comparative evaluation of Machine Learning inference machines on Edge-class compute engines. The testbed consists of two hardware compute engines (i.e., CPU-based Raspberry Pi 4 and Google Edge TPU accelerator) and two inference machines (i.e., TensorFlow-Lite and Arm NN). Through an extensive set of experiments in our bespoke testbed, we compared three setups using TensorFlow-Lite ML framework, in terms of accuracy, execution time, and energy efficiency. Based on the results, an optimized configuration of the workload parameters can increase accuracy by 10%, and in addition, the class of the Edge compute engine in combination with the inference machine affects execution time by 86% and power consumption by almost 145%.
更多查看译文
关键词
edge computing, machine learning, inference machine
AI 理解论文
溯源树
样例
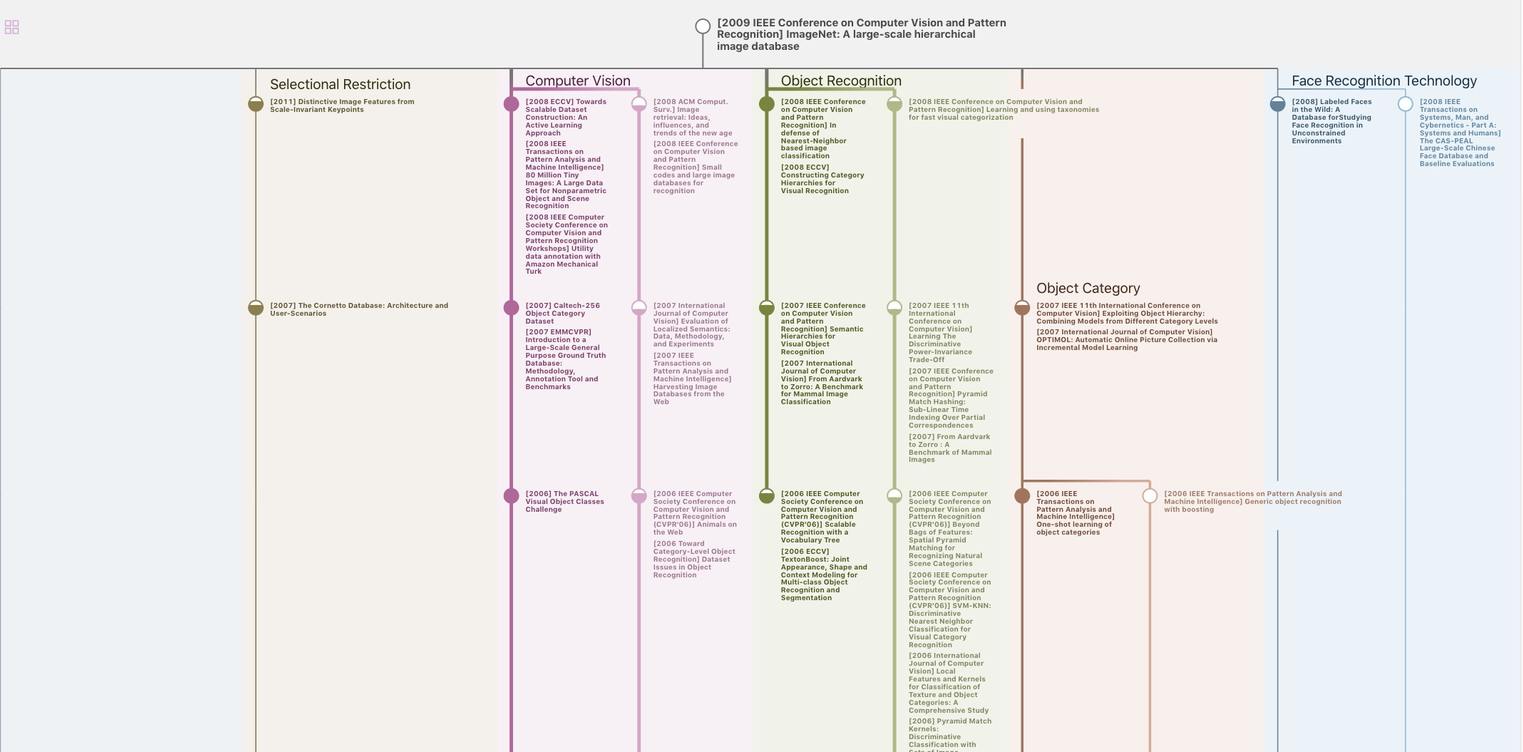
生成溯源树,研究论文发展脉络
Chat Paper
正在生成论文摘要