Abstract TMP70: Comparing Machine Learning Algorithms And Regression Models For Predicting Functional Outcome In The Stratis Registry
Stroke(2022)
摘要
Background: Machine learning algorithms have emerged as powerful predictive tools in the field of acute ischemic stroke. Here, we examine the predictive performance of a machine algorithm compared to logistic regression for predicting functional outcomes in the prospective Systematic Evaluation of Patients Treated With Neurothrombectomy Devices for Acute Ischemic Stroke (STRATIS) Registry. Methods: The STRATIS Registry was a prospective, observational study of the use of the Solitaire device in acute ischemic stroke patients. Patients with posterior circulation stroke or missing 90-day mRS were excluding from the analysis. A statistical algorithm (logistic regression) and a machine learning algorithm (decision tree) were implemented on the preprocessed dataset using 10-fold cross-validation method where 80% of the data were fed into the models to be trained and the remaining 20% were utilized in the test phase to evaluate the performance of the models for prediction of 90-day mRS score as dichotomous output (0-2=1, 3-6=0). Results: Of the 938 STRATIS patients, 702 with 90-day mRS were included. The machine learning model outperformed the logistic regression model with a 0.92±0.026 Area Under Curve (AUC) score compared to a 0.88±0.028 AUC score obtained by implementing logistic regression. Conclusion: Our machine learning model delivered improved performance in comparison with the statistical model in predicting 90-day functional outcome. More studies are needed to understand and externally validate the predictive capacity of our machine learning model.
更多查看译文
AI 理解论文
溯源树
样例
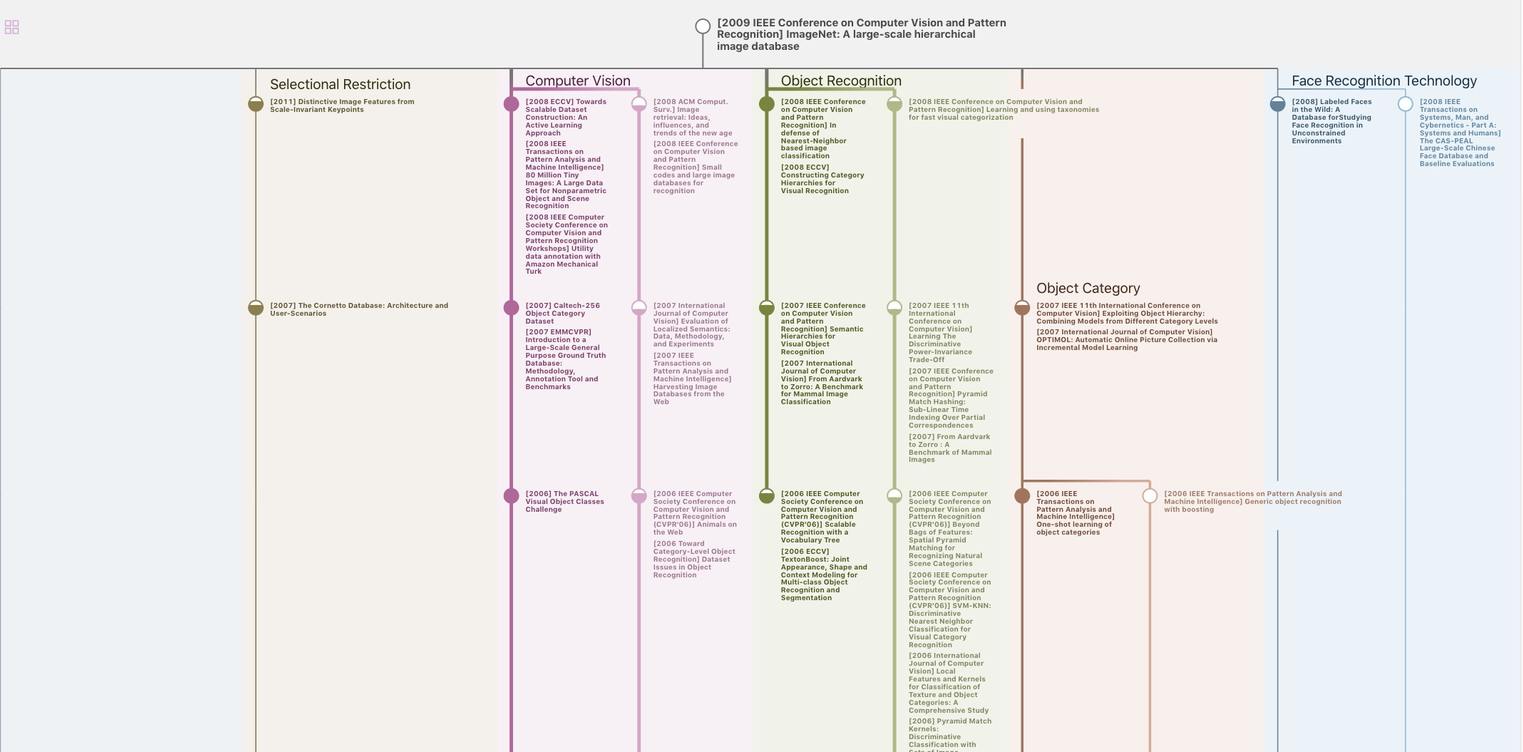
生成溯源树,研究论文发展脉络
Chat Paper
正在生成论文摘要