Enhanced machine learning methods for nonlinear harmonic source modelling
CIRED 2021 - The 26th International Conference and Exhibition on Electricity Distribution(2021)
摘要
Power electronic-based devices inject harmonic perturbations in electrical networks and are thus a concern for network operators who must ensure that the harmonic injections and the resulting voltage disturbances are kept within acceptable limits. In this paper, we show that time domain (TD) methods can be useful in preliminary harmonic source modelling to derive the simulated models. Using TD SIMULINK models of our devices, we find the most influential internal and external parameters to be considered when the device is operating in a pre-existing harmonic environment and we generate datasets that can be used for training machine learning (ML) algorithms. We propose here three ML techniques to predict the harmonic currents originating from most widespread power electronic devices such as Light Emitting Diodes (LED) lamps and Electric Vehicle (EV) chargers, and compare them with TD models. The accuracy of all these models regarding their harmonic currents prediction capability is assessed under constantly evolving supply conditions.
更多查看译文
关键词
nonlinear harmonic source modelling,machine learning
AI 理解论文
溯源树
样例
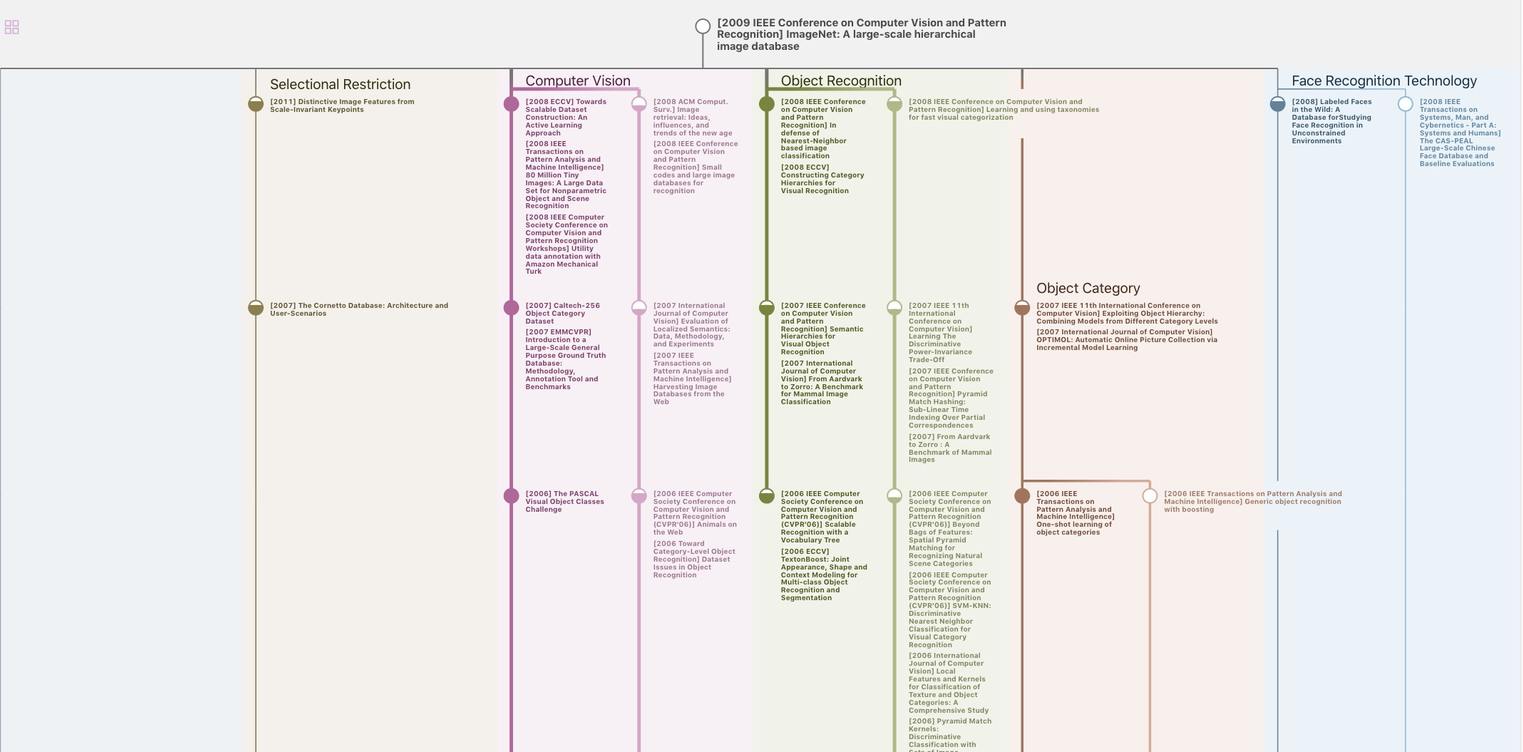
生成溯源树,研究论文发展脉络
Chat Paper
正在生成论文摘要