From Individual to Whole: Reducing Intra-class Variance by Feature Aggregation
International Journal of Computer Vision(2022)
摘要
The recording process of observation is influenced by multiple factors, such as viewpoint, illumination, and state of the object-of-interest etc.Thus, the image observation of the same object may vary a lot under different conditions. This leads to severe intra-class variance which greatly challenges the discrimination ability of the vision model. However, the current prevailing softmax loss for visual recognition only pursues perfect inter-class separation in the feature space. Without considering the intra-class compactness, the learned model easily collapses when it encounters the instances that deviate a lot from their class centroid. To resist the intra-class variance, we start by organizing the input instances as a graph. From this viewpoint, we find that the normalized cut on the graph is a favorable surrogate metric of the intra-class variance within the training batch. Inspired by the equivalence between the normalized cut and random walk, we propose a feature aggregation scheme using transition probabilities as guidance. By imposing supervision on the aggregated features, we can constrain the transition probabilities to form a graph partition consistent with the given labels. Thus, the normalized cut as well as intra-class variance can be well suppressed. To validate the effectiveness of this idea, we instantiate it in spatial, temporal, and spatial-temporal scenarios. Experimental results on corresponding benchmarks demonstrate that the proposed feature aggregation leads to significant improvement in performance. Our method is on par with, or even better than current state-of-the-arts in both tasks.
更多查看译文
关键词
Feature aggregation,Deep learning,Intra-class variance,Person re-identification,Video object detection
AI 理解论文
溯源树
样例
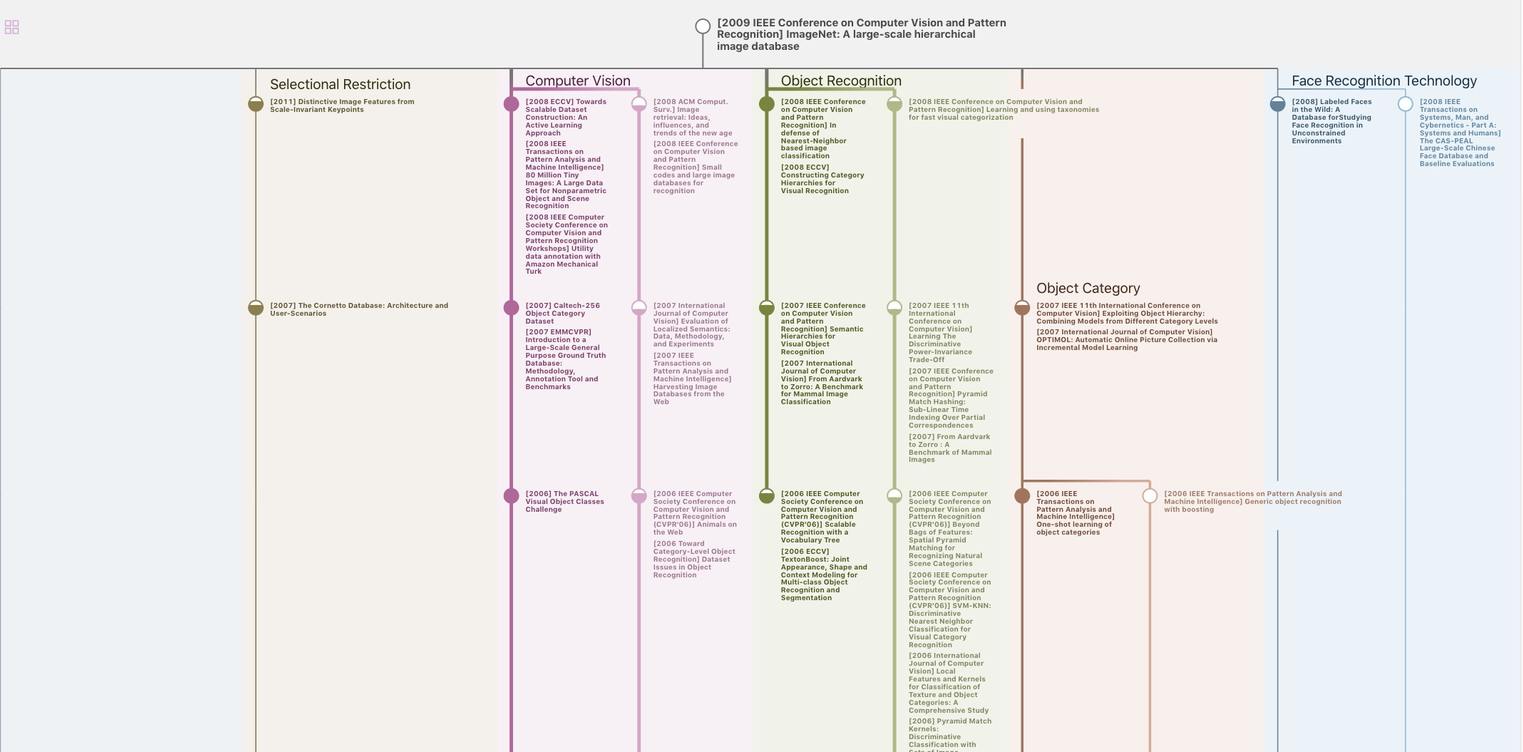
生成溯源树,研究论文发展脉络
Chat Paper
正在生成论文摘要