Machine Learning for Hidden Nodes Detection in Unlicensed LTE Networks
Computer Networks(2022)
摘要
This paper analyzes and evaluates the use of Machine Learning (ML) techniques as an alternative to heuristic methods to identify UEs in Licensed-Assisted Access (LAA) networks that are being affected by collisions from hidden nodes sources. The paper analyzes different models based on supervised ML methods to classify UEs into two possible classes: UEs affected by collisions and UEs free of collisions. To implement them, we employ a set of typical parameters of the LTE standard, e.g., Channel Quality Indicator (CQI), Reference Signal Received Quality (RSRQ) and number of Physical Resource Blocks (PRB). These parameters are fed into different supervised ML models which, once optimized, obtain the best models to predict when an UE is facing interference from hidden nodes in a dynamic channel environment. Results show that classification methods based on ML techniques are capable of predicting with a high level of accuracy when a UE is affected by collisions and, consequently, if this node is located in a hidden area. Nevertheless, and despite the growing interest in applying ML techniques in an increasing number of fields, we can conclude that, in this case, the performance of the ML approach is not necessarily better than heuristic methods. For instance, it does not outperform the Dynamic Collision Detection (DCD) previously presented by the authors. In addition, the ML algorithms exhibit a higher prediction time and computational complexity, with respect to those obtained with DCD algorithm. This should be considered a drawback in some LTE-Advanced and 5G wireless network scenarios that prioritize lower latencies.
更多查看译文
关键词
LAA,CQI,RSRQ,Hidden node problem,Machine learning,Unlicensed spectrum
AI 理解论文
溯源树
样例
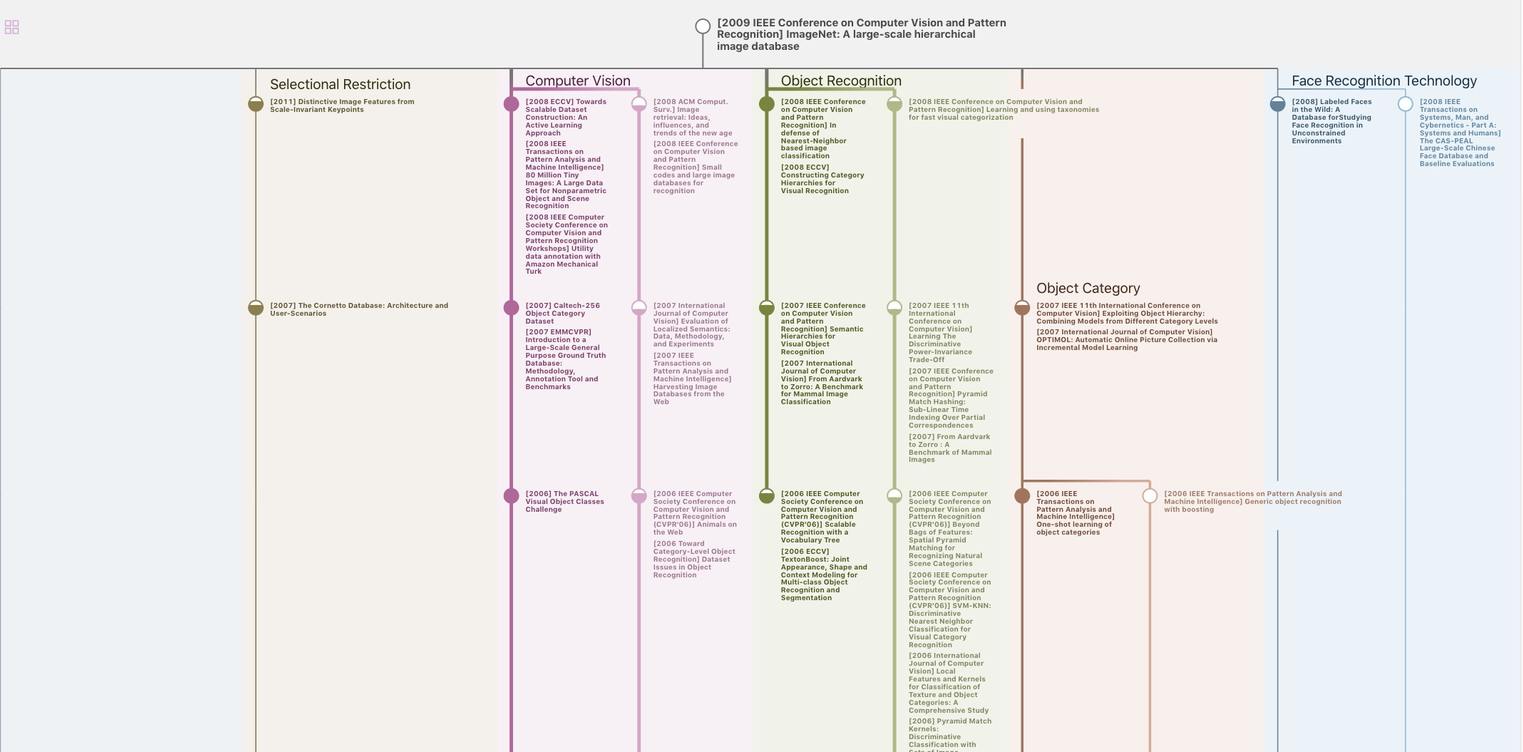
生成溯源树,研究论文发展脉络
Chat Paper
正在生成论文摘要