The Role of ImageNet Classes in Fr\'echet Inception Distance
arXiv (Cornell University)(2023)
Abstract
Fr\'echet Inception Distance (FID) is the primary metric for ranking models in data-driven generative modeling. While remarkably successful, the metric is known to sometimes disagree with human judgement. We investigate a root cause of these discrepancies, and visualize what FID "looks at" in generated images. We show that the feature space that FID is (typically) computed in is so close to the ImageNet classifications that aligning the histograms of Top-$N$ classifications between sets of generated and real images can reduce FID substantially -- without actually improving the quality of results. Thus, we conclude that FID is prone to intentional or accidental distortions. As a practical example of an accidental distortion, we discuss a case where an ImageNet pre-trained FastGAN achieves a FID comparable to StyleGAN2, while being worse in terms of human evaluation.
MoreTranslated text
Key words
imagenet classes
AI Read Science
Must-Reading Tree
Example
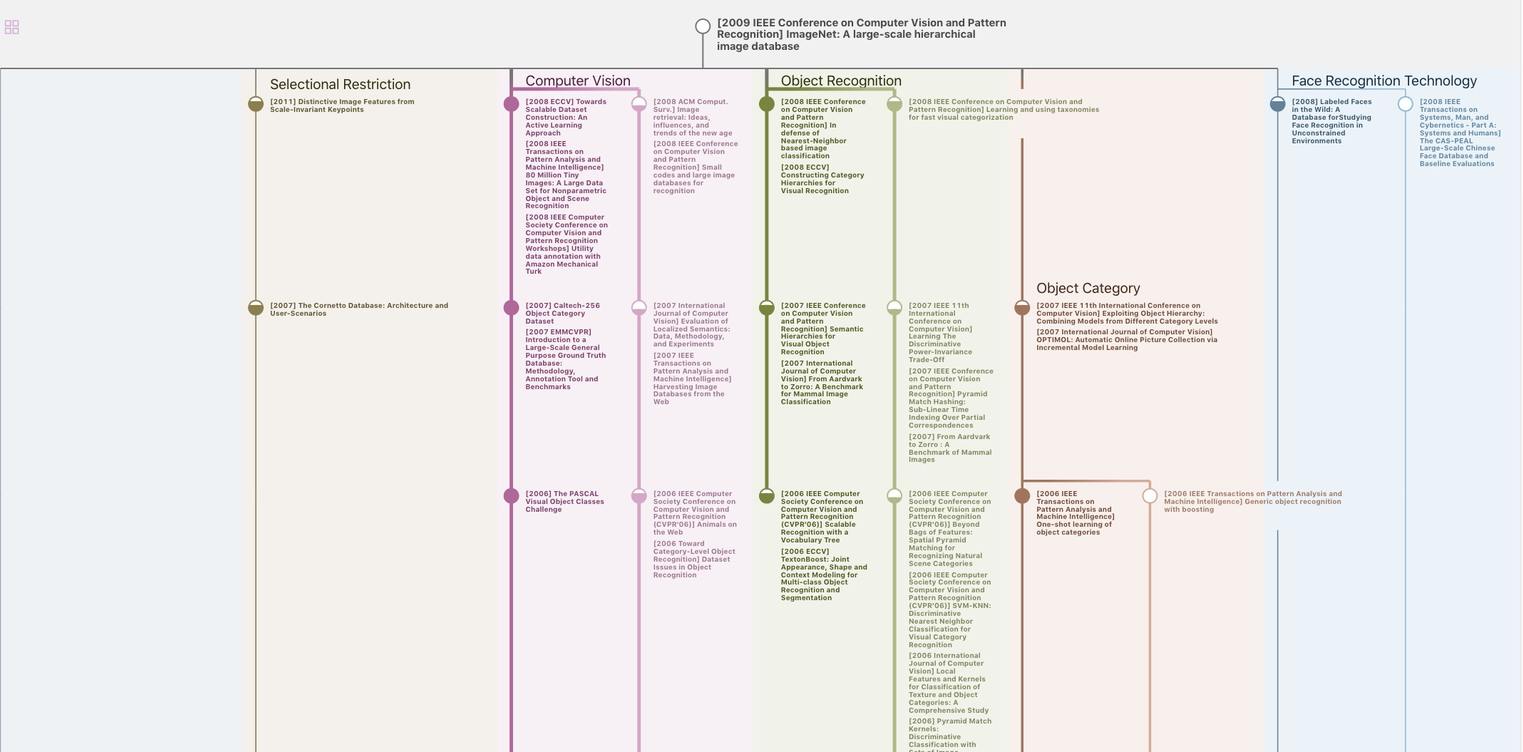
Generate MRT to find the research sequence of this paper
Chat Paper
Summary is being generated by the instructions you defined