A geometrical viewpoint on the benign overfitting property of the minimum $l_2$-norm interpolant estimator
arxiv(2022)
摘要
Practitioners have observed that some deep learning models generalize well even with a perfect fit to noisy training data [5,45,44]. Since then many theoretical works have revealed some facets of this phenomenon [4,2,1,8] known as benign overfitting. In particular, in the linear regression model, the minimum $l_2$-norm interpolant estimator $\hat\beta$ has received a lot of attention [1,39] since it was proved to be consistent even though it perfectly fits noisy data under some condition on the covariance matrix $\Sigma$ of the input vector. Motivated by this phenomenon, we study the generalization property of this estimator from a geometrical viewpoint. Our main results extend and improve the convergence rates as well as the deviation probability from [39]. Our proof differs from the classical bias/variance analysis and is based on the self-induced regularization property introduced in [2]: $\hat\beta$ can be written as a sum of a ridge estimator $\hat\beta_{1:k}$ and an overfitting component $\hat\beta_{k+1:p}$ which follows a decomposition of the features space $\mathbb{R}^p=V_{1:k}\oplus^\perp V_{k+1:p}$ into the space $V_{1:k}$ spanned by the top $k$ eigenvectors of $\Sigma$ and the ones $V_{k+1:p}$ spanned by the $p-k$ last ones. We also prove a matching lower bound for the expected prediction risk. The two geometrical properties of random Gaussian matrices at the heart of our analysis are the Dvoretsky-Milman theorem and isomorphic and restricted isomorphic properties. In particular, the Dvoretsky dimension appearing naturally in our geometrical viewpoint coincides with the effective rank from [1,39] and is the key tool to handle the behavior of the design matrix restricted to the sub-space $V_{k+1:p}$ where overfitting happens.
更多查看译文
AI 理解论文
溯源树
样例
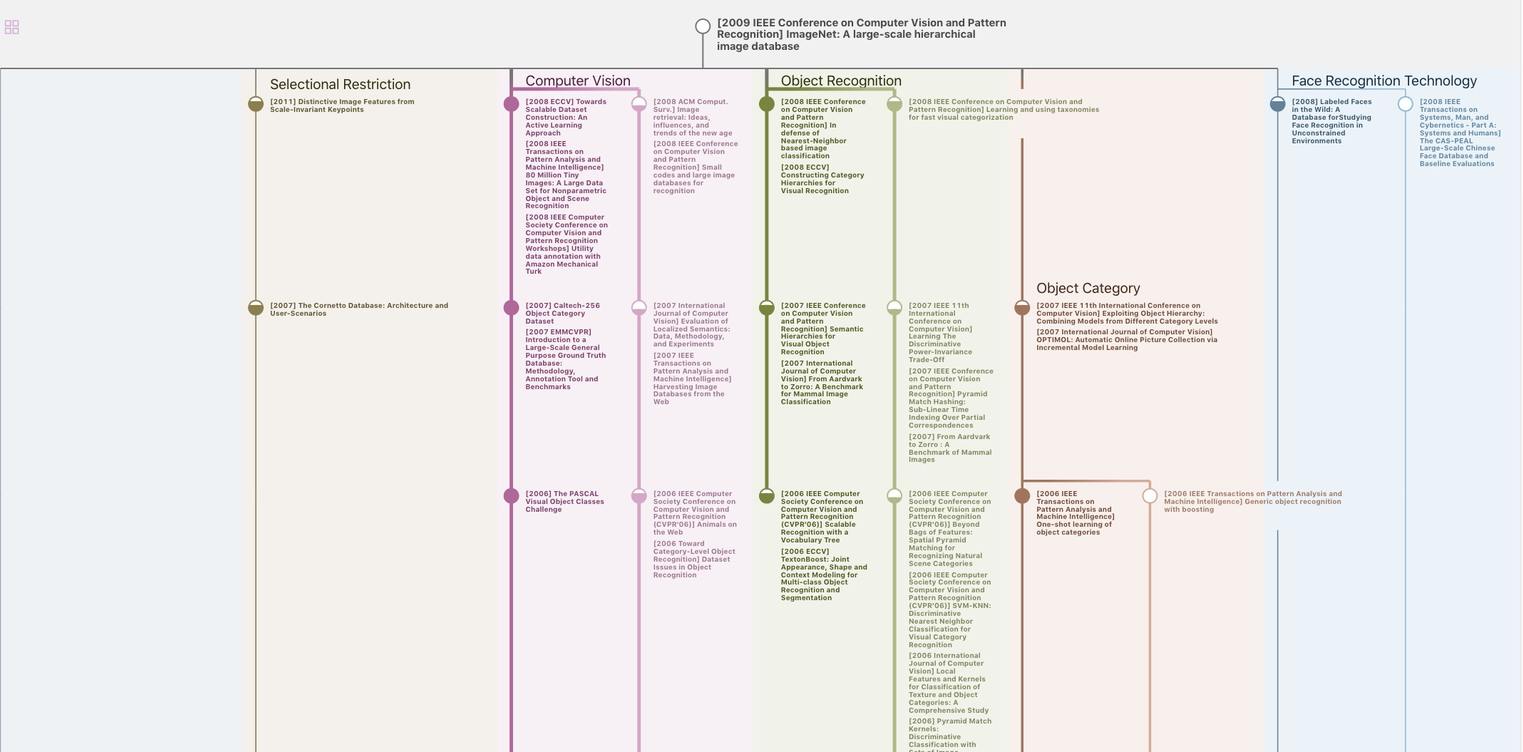
生成溯源树,研究论文发展脉络
Chat Paper
正在生成论文摘要