No Free Lunch Theorem for Security and Utility in Federated Learning
ACM Transactions on Intelligent Systems and Technology(2023)
摘要
AbstractIn a federated learning scenario where multiple parties jointly learn a model from their respective data, there exist two conflicting goals for the choice of appropriate algorithms. On one hand, private and sensitive training data must be kept secure as much as possible in the presence of semi-honest partners; on the other hand, a certain amount of information has to be exchanged among different parties for the sake of learning utility. Such a challenge calls for the privacy-preserving federated learning solution, which maximizes the utility of the learned model and maintains a provable privacy guarantee of participating parties’ private data.This article illustrates a general framework that (1) formulates the trade-off between privacy loss and utility loss from a unified information-theoretic point of view, and (2) delineates quantitative bounds of the privacy-utility trade-off when different protection mechanisms including randomization, sparsity, and homomorphic encryption are used. It was shown that in general there is no free lunch for the privacy-utility trade-off, and one has to trade the preserving of privacy with a certain degree of degraded utility. The quantitative analysis illustrated in this article may serve as the guidance for the design of practical federated learning algorithms.
更多查看译文
关键词
Federated learning,privacy-preserving computing,security,utility,trade-off,divergence,optimization
AI 理解论文
溯源树
样例
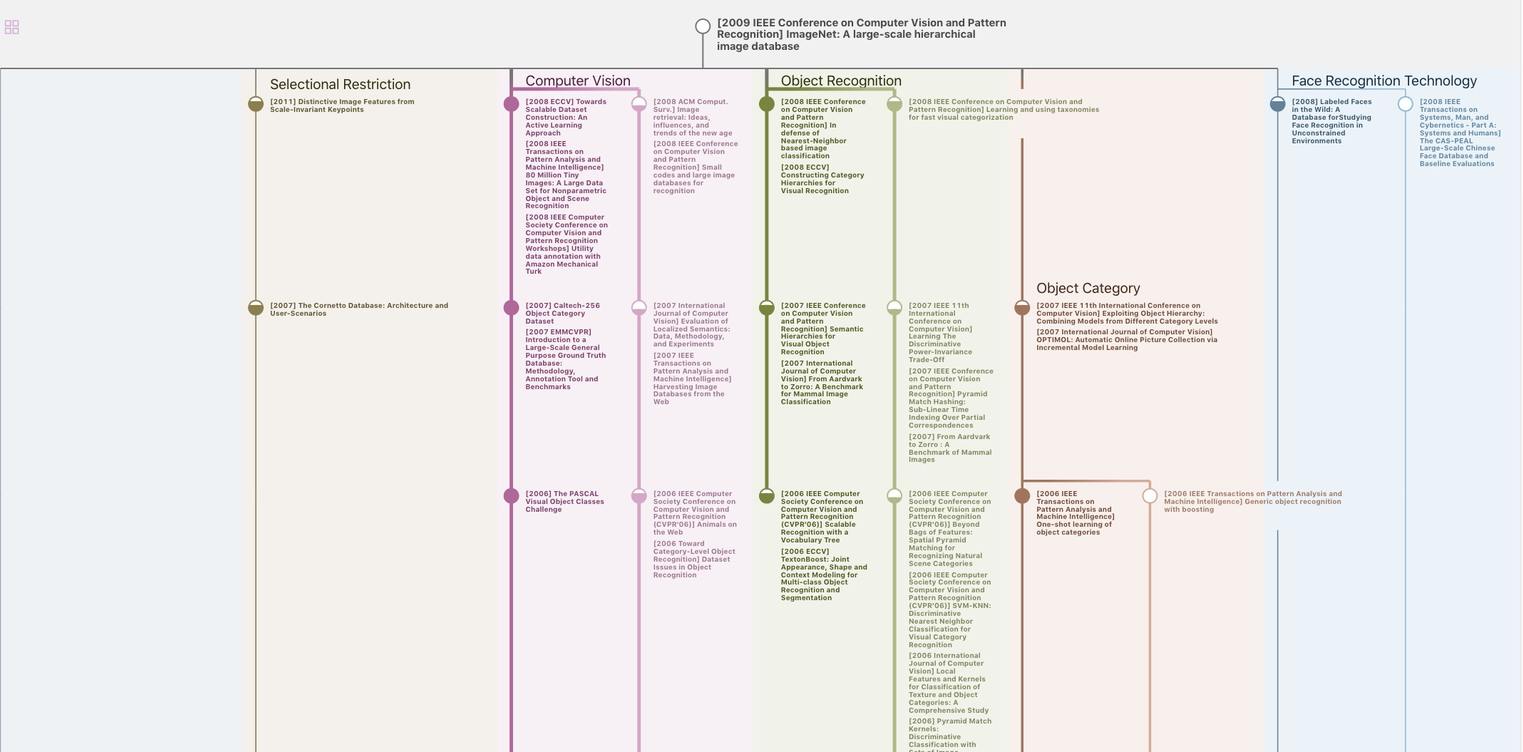
生成溯源树,研究论文发展脉络
Chat Paper
正在生成论文摘要