Identification and analysis of offenders causing hit and run accidents using classification algorithms
INTERNATIONAL JOURNAL OF INJURY CONTROL AND SAFETY PROMOTION(2022)
Abstract
Hit-and-run crashes are significant concern for many countries. Due to lack of information of offending vehicles it is difficult to understand dynamics of these crashes to have a prevention plan. The paper aims to identify the impacting vehicle in hit-and-run crashes. We studied fatal road crashes of New Delhi for eleven years (2006-2016) and found that approximately 40% fatal crashes are hit-and-run with unknown impacting vehicles. We proposed a framework using eleven different machine learning-based classification algorithms - Logistic-Regression, KNN, SVM-Linear and RBF-Kernel, Naive-Bayes, Random-Forest, DecisionTree, AdaBoost, Multilayer-Perceptron, CART and Linear-Discriminant-Analysis. We found SVM-linear-kernel gave best results. Results reveal that cars, buses, and heavy vehicles are involved vehicles in hit-and-run crashes. Buses were primary cause leading to 39% of hit-and-run during 2006-2009 thereafter cars increased drastically. Our framework is robust and scalable to any city. The outcomes provide inputs to traffic engineers for better policy prescription and road user safety.
MoreTranslated text
Key words
Hit and run accidents, road user safety, road Accidents, accident prevention, machine learning models, accident prediction, support vector machine
AI Read Science
Must-Reading Tree
Example
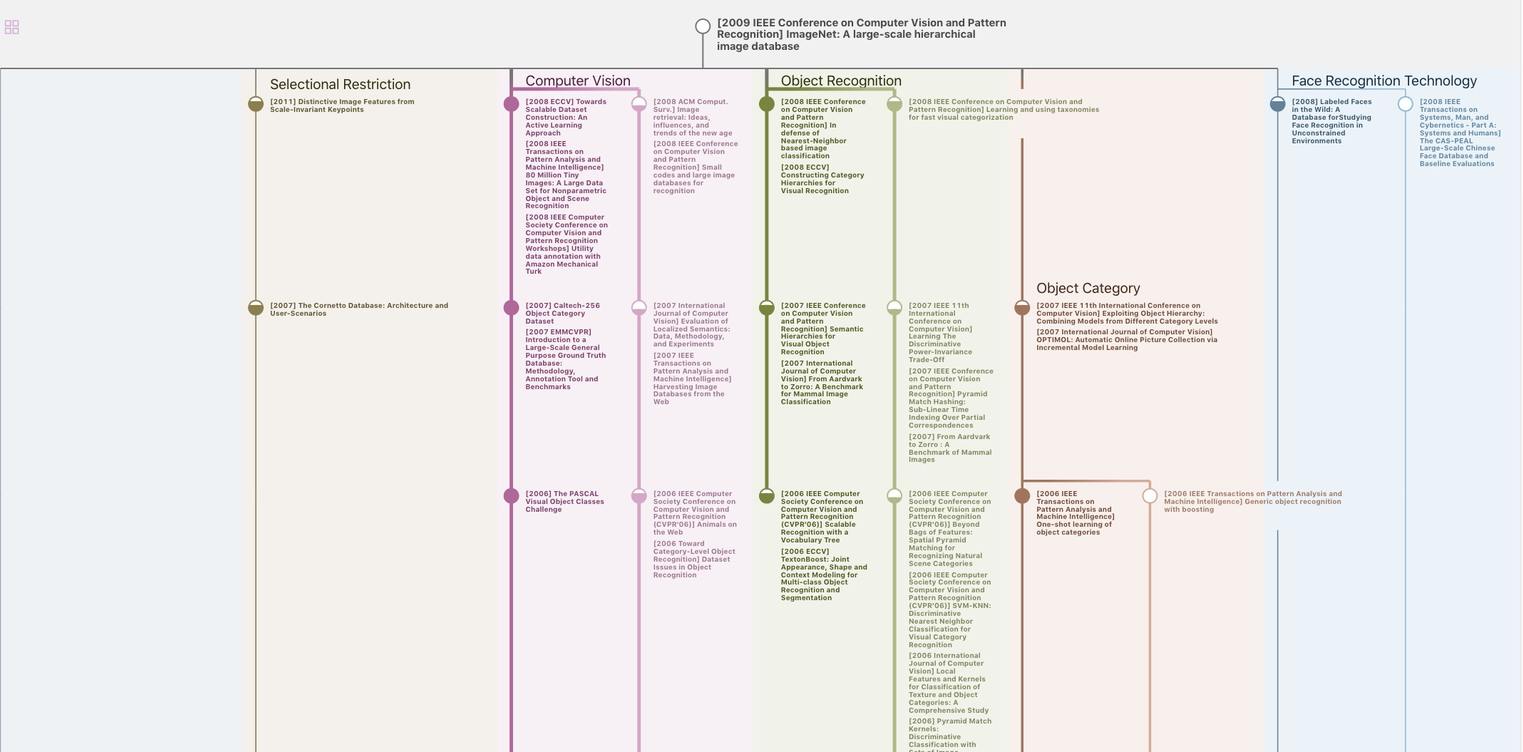
Generate MRT to find the research sequence of this paper
Chat Paper
Summary is being generated by the instructions you defined