Lessons Learned from a Distributed RF-EMF Sensor Network
Italian National Conference on Sensors(2022)
关键词
radiofrequency electromagnetic fields (RF-EMF),spatiotemporal exposure assessment,sensor node,distributed sensor network
AI 理解论文
溯源树
样例
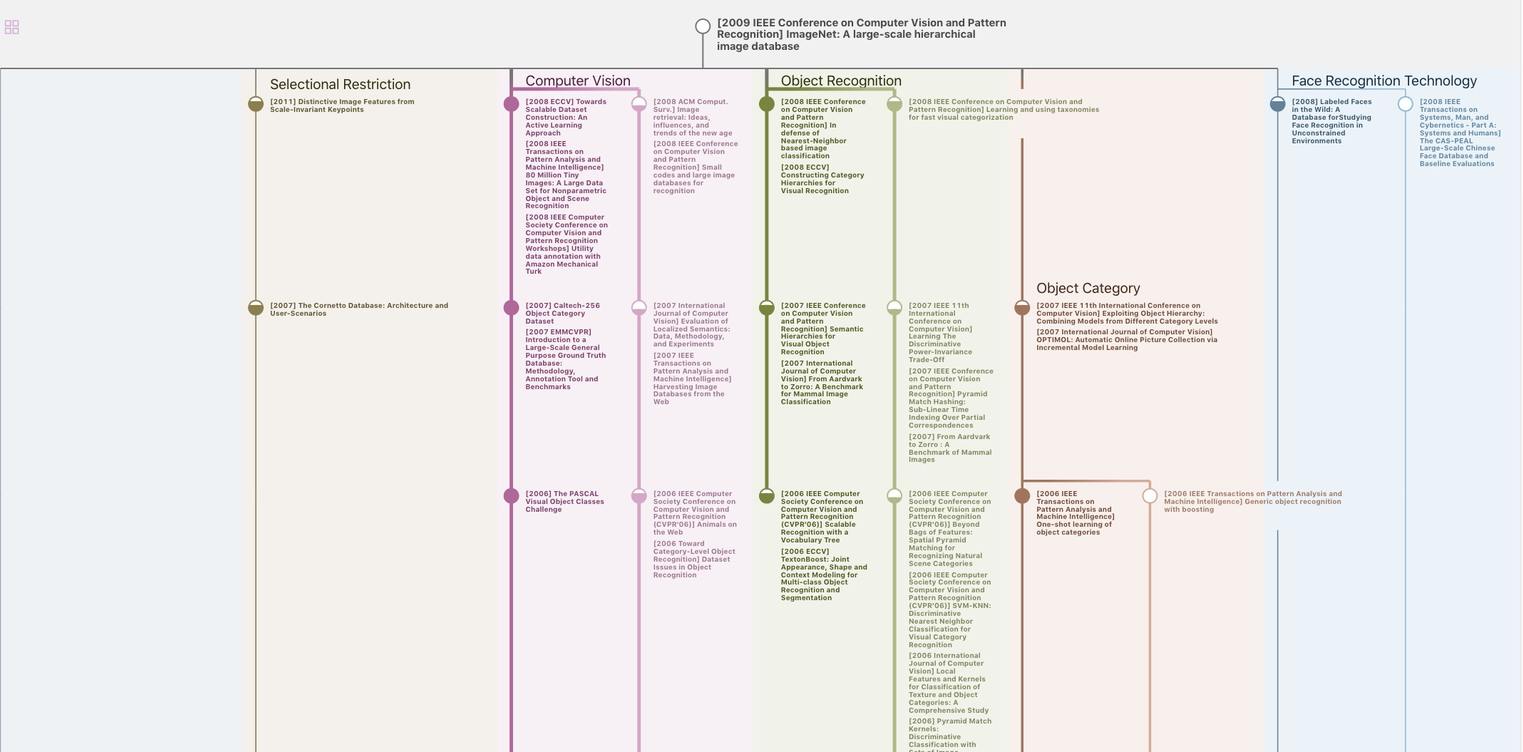
生成溯源树,研究论文发展脉络
Chat Paper
正在生成论文摘要