Iterative Corresponding Geometry: Fusing Region and Depth for Highly Efficient 3D Tracking of Textureless Objects
IEEE Conference on Computer Vision and Pattern Recognition(2022)
摘要
Tracking objects in 3D space and predicting their 6DoF pose is an essential task in computer vision. State-of-the-art approaches often rely on object texture to tackle this problem. However, while they achieve impressive results, many objects do not contain sufficient texture, violating the main underlying assumption. In the following, we thus propose ICG, a novel probabilistic tracker that fuses region and depth information and only requires the object geometry. Our method deploys correspondence lines and points to iteratively refine the pose. We also implement robust occlusion handling to improve performance in real-world settings. Experiments on the YCB-Video, OPT, and Choi datasets demonstrate that, even for textured objects, our approach outperforms the current state of the art with respect to accuracy and robustness. At the same time, ICG shows fast convergence and outstanding efficiency, requiring only 1.3 ms per frame on a single CPU core. Finally, we analyze the influence of individual components and discuss our performance compared to deep learning-based methods. The source code of our tracker is publicly available 1 1 https://github.com/DLR-RM/3DObjectTracking.
更多查看译文
关键词
Pose estimation and tracking, Motion and tracking, Optimization methods, Robot vision, Statistical methods
AI 理解论文
溯源树
样例
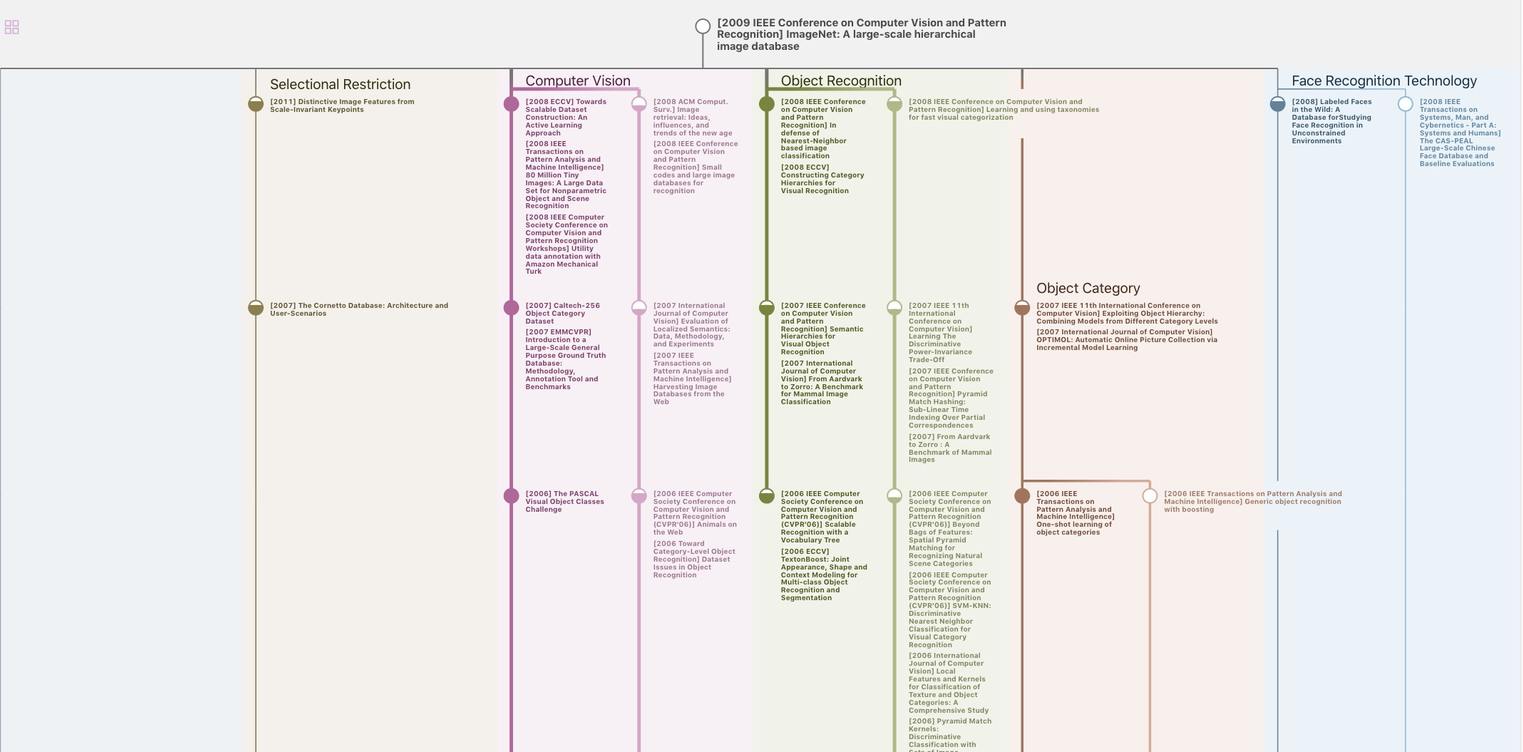
生成溯源树,研究论文发展脉络
Chat Paper
正在生成论文摘要