SelfTune: Metrically Scaled Monocular Depth Estimation through Self-Supervised Learning
2022 IEEE INTERNATIONAL CONFERENCE ON ROBOTICS AND AUTOMATION (ICRA 2022)(2022)
Abstract
Monocular depth estimation in the wild inherently predicts depth up to an unknown scale. To resolve scale ambiguity issue, we present a learning algorithm that leverages monocular simultaneous localization and mapping (SLAM) with proprioceptive sensors. Such monocular SLAM systems can provide metrically scaled camera poses. Given these metric poses and monocular sequences, we propose a self-supervised learning method for the pre-trained supervised monocular depth networks to enable metrically scaled depth estimation. Our approach is based on a teacher-student formulation which guides our network to predict high-quality depths. We demonstrate that our approach is useful for various applications such as mobile robot navigation and is applicable to diverse environments. Our full system shows improvements over recent self-supervised depth estimation and completion methods on EuRoC, OpenLORIS, and ScanNet datasets.
MoreTranslated text
AI Read Science
Must-Reading Tree
Example
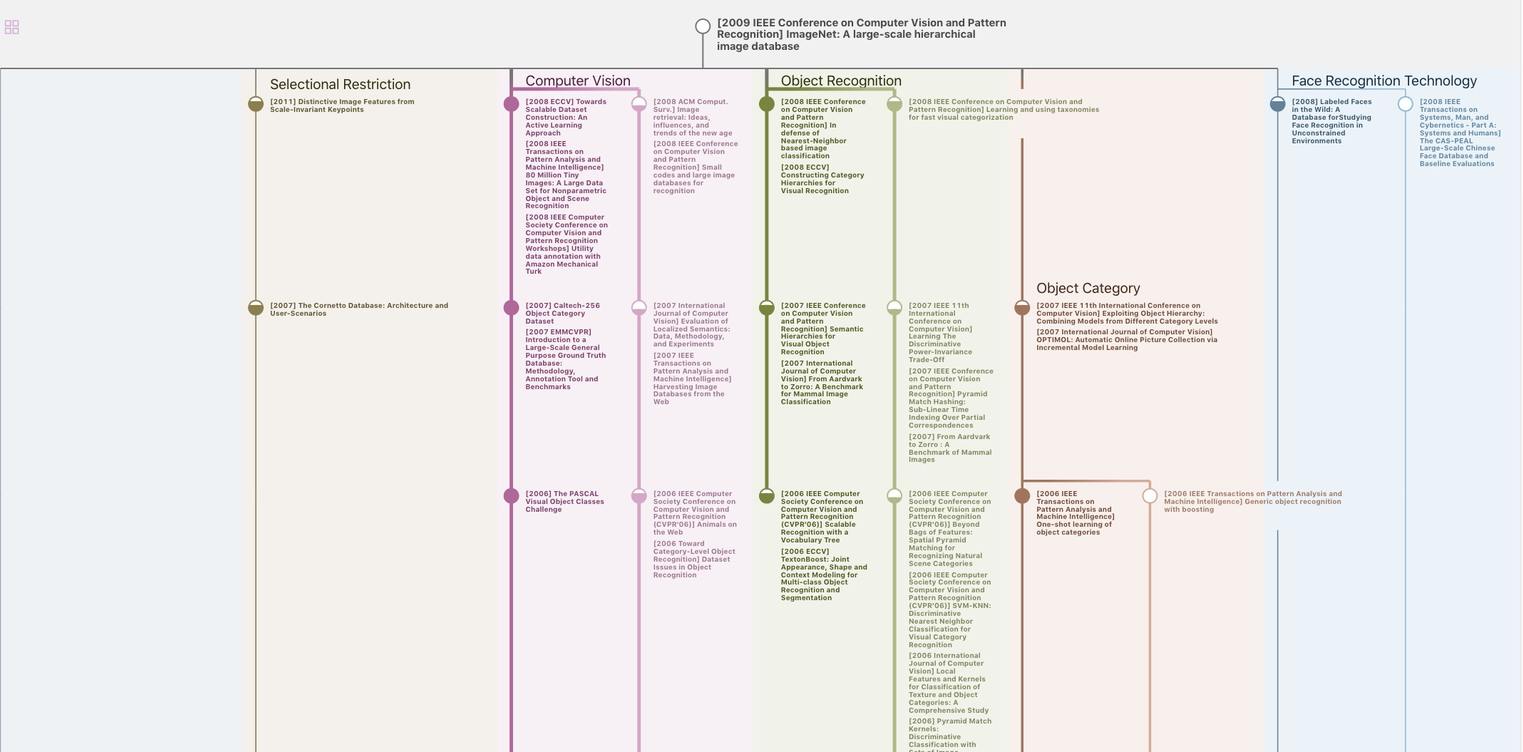
Generate MRT to find the research sequence of this paper
Chat Paper
Summary is being generated by the instructions you defined