Quantitative Analysis of Different Land-use and Land-cover Classifiers using Hyperspectral Dataset
2021 Sixth International Conference on Image Information Processing (ICIIP)(2021)
摘要
Remote sensing plays a vital role in analyzing land-use and land-cover (LULC) areas using hyperspectral imagery which was not feasible with multispectral imagery. The analysis of LULC is an important factor for environmental application. Classification is one of the methods used for the categorization of different types of class cateogries. The performance evaluation of various classifiers using hyperspectral dataset is essential to be performed for the categorization of various classes. Therefore, the main aim of this paper is to analyze and implement the various supervised classifiers such as random forest (RF), neural network (NN), and minimum distance classifier (MDC). The hyperspectral dataset has been used for these classifiers over a part of Haryana and Uttar Pradesh states, India. The results have shown that NN (88%) algorithm has achieved higher accuracy than other algorithms. These experimental outcomes highlights the potential of NN in handling complex problems and useful in the mapping of changes over LULC using hyperspectral imagery. This study is beneficial in applications such as crop monitoring, mapping seasonal variations, and snow detection.
更多查看译文
关键词
Hyperspectral,Land-use (LU) random forest (RF),neural network (NN),minimum distance classifier (MDC)
AI 理解论文
溯源树
样例
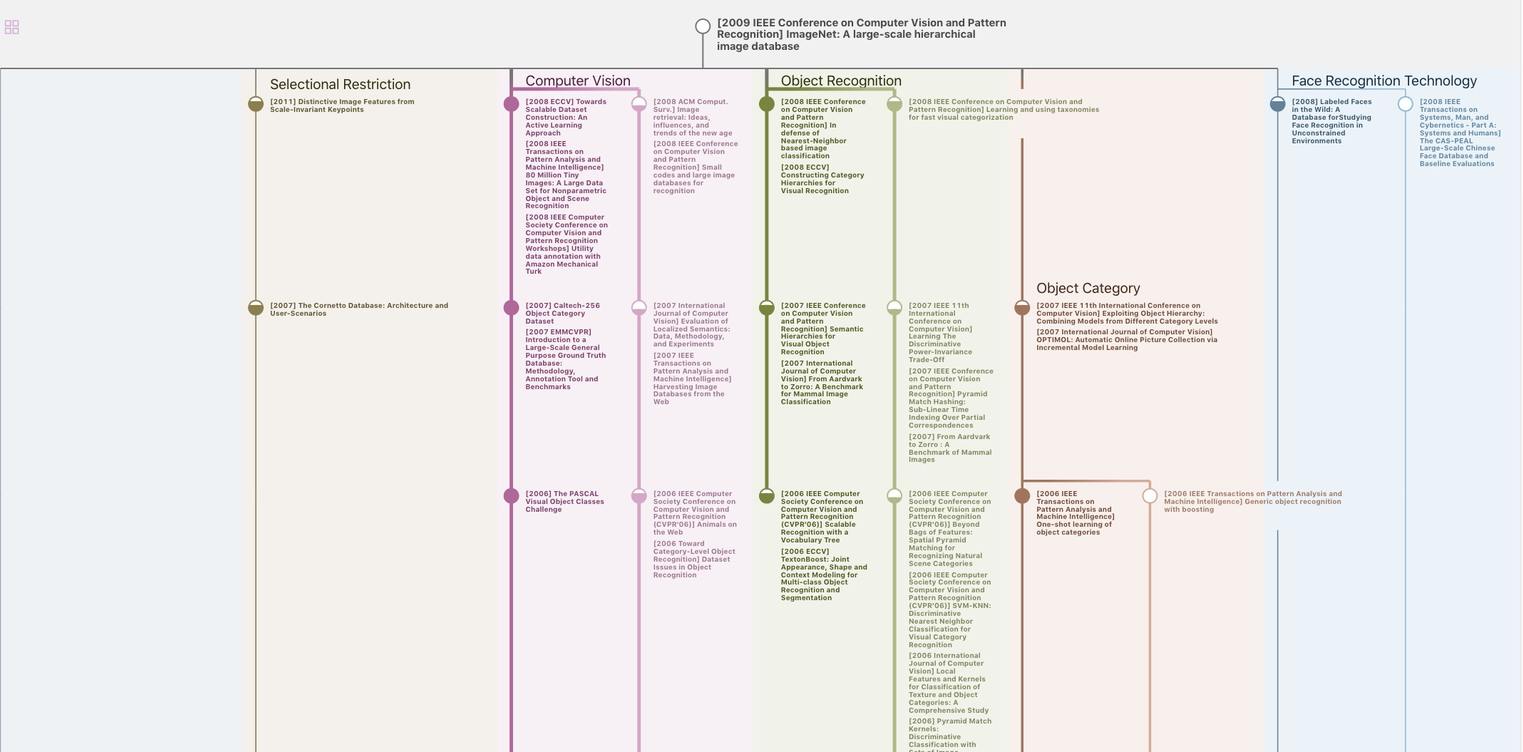
生成溯源树,研究论文发展脉络
Chat Paper
正在生成论文摘要