Response Prediction Based on Temporal and Spatial Deep Learning Model for Intelligent Structural Health Monitoring
IEEE Internet of Things Journal(2022)
摘要
Machine learning models have recently demonstrated the ability to mine structural health monitoring data. While existing machine learning models can provide better performance than the previous univariate time-series model, it is still an open challenge of fully mining the spatial and temporal characteristics of the structural response to increase their accuracy. In addition, the heterogeneous correlation is always missed in traditional models. This article proposes a heterogeneous structural response prediction (HSRP) framework based on the deep learning model to improve the performance. The HSRP framework cannot only make full use of spatial and temporal correlations but also mine the correlation between heterogeneous responses. Motivated by recent studies in machine learning, an attention module is introduced to learn the correlation between different responses and initial the weights of sensors and past response. The convolutional neural network is also implemented to extract the spatial features and the long short-term memory network is used to extract the weekly, daily, and hourly patterns of structural response. A real-world data set collected from a bridge is used to evaluate the performance of the proposed model on single-step prediction and multistep prediction. The experimental results show that the proposed model outperforms several widely used benchmark models. Furthermore, additional experiments and evaluations are implemented to investigate the sensitivity and robustness of the proposed model.
更多查看译文
关键词
Deep neural network,intelligent structural health monitoring system (ISHMS),Internet of Things,prediction
AI 理解论文
溯源树
样例
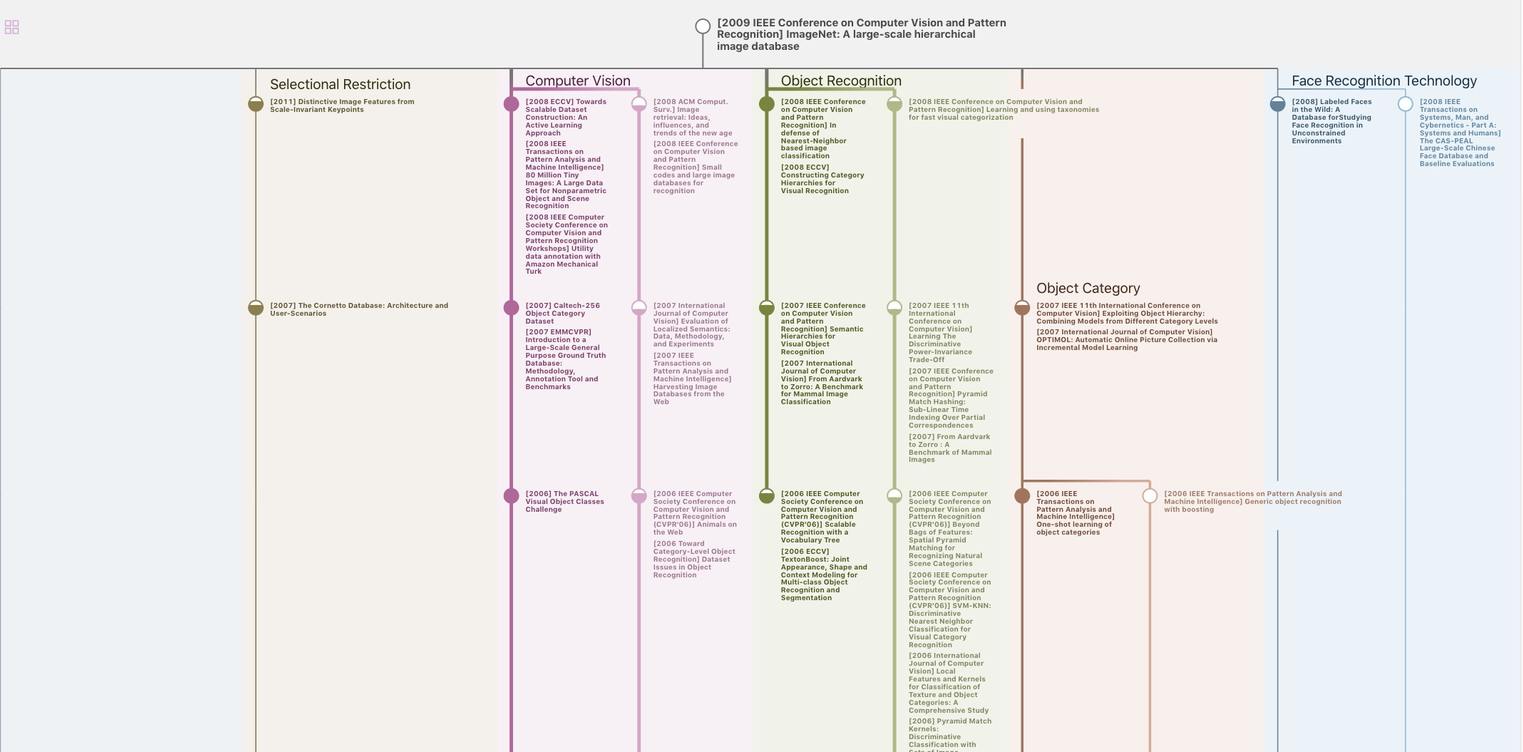
生成溯源树,研究论文发展脉络
Chat Paper
正在生成论文摘要