An Automatic Driving Control Method Based on Deep Deterministic Policy Gradient
WIRELESS COMMUNICATIONS & MOBILE COMPUTING(2022)
摘要
The traditional automatic driving behavior decision algorithm needs to manually set complex rules, resulting in long vehicle decision-making time, poor decision-making effect, and no adaptability to the new environment. As one of the main methods in the field of machine learning and intelligent control in recent years, reinforcement learning can learn reasonable and effective policies only by interacting with the environment. Firstly, this paper introduces the current research status of automatic driving technology and the current mainstream automatic driving control methods. Then, it analyzes the characteristics of convolutional neural network, reinforcement learning method (Q-learning), and deep Q network (DQN) and deep deterministic policy gradient (DDPG). Compared with the DQN algorithm based on value function, the DDPG algorithm based on action policy can well solve the continuity problem of action space. Finally, the DDPG algorithm is used to solve the control problem of automatic driving. By designing a reasonable reward function, deep convolution network, and exploration policy, the intelligent vehicle can avoid obstacles and, finally, achieve the purpose of avoiding obstacles and running the whole process in a 2D environment.
更多查看译文
AI 理解论文
溯源树
样例
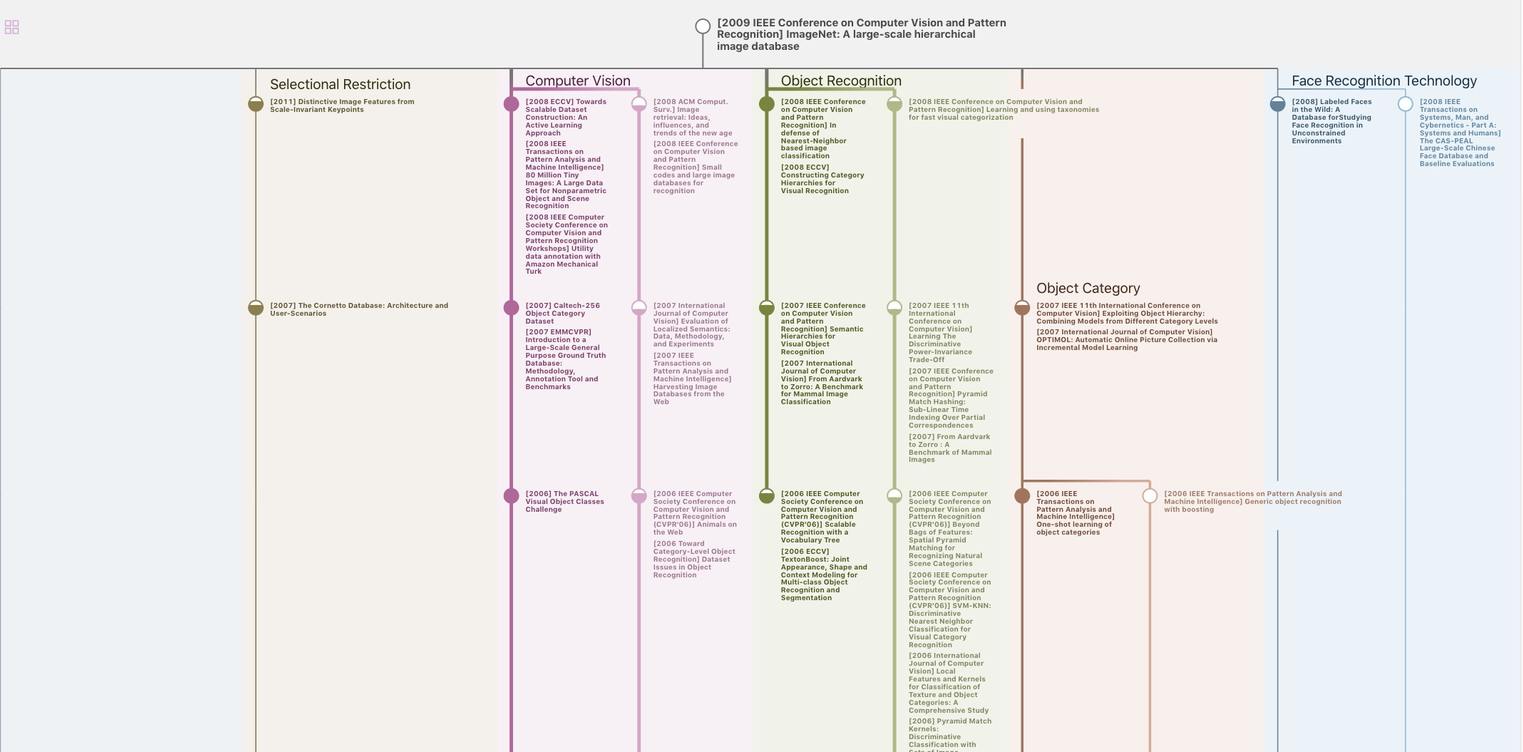
生成溯源树,研究论文发展脉络
Chat Paper
正在生成论文摘要