Inversion of low to medium frequency velocities and densities from AVO data using invertible neural networks
Geophysics(2022)
摘要
AVO (amplitude variation with offset) inversion and neural networks are widely used to invert elastic parameters. With more constraints from well log data, neural network-based inversion may estimate elastic parameters with greater precision and resolution than traditional AVO inversion, however, neural network approaches necessitate a massive number of reliable training samples. Furthermore, because the lack of low-frequency information in seismic gathers leads to multiple solutions of the inverse problem, both inversions rely heavily on proper low-frequency initial models. To mitigate the dependence of inversions on accurate training samples and initial models, we propose solving inverse problems with the recently developed invertible neural networks (INNs). Unlike conventional neural networks, which address the ambiguous inverse issues directly, INNs learn definite forward modeling and use additional latent variables to increase the uniqueness of solutions. Motivated by the newly developed neural networks, we propose an INN-based AVO inversion method, which can reliably invert low to medium frequency velocities and densities with randomly generated easy-to-access datasets rather than trustworthy training samples or well-prepared initial models. Tests on synthetic and field data show that our method is feasible, anti-noise capable, and practicable.
更多查看译文
关键词
invertible neural networks,avo data,inversion,neural networks,medium-frequency
AI 理解论文
溯源树
样例
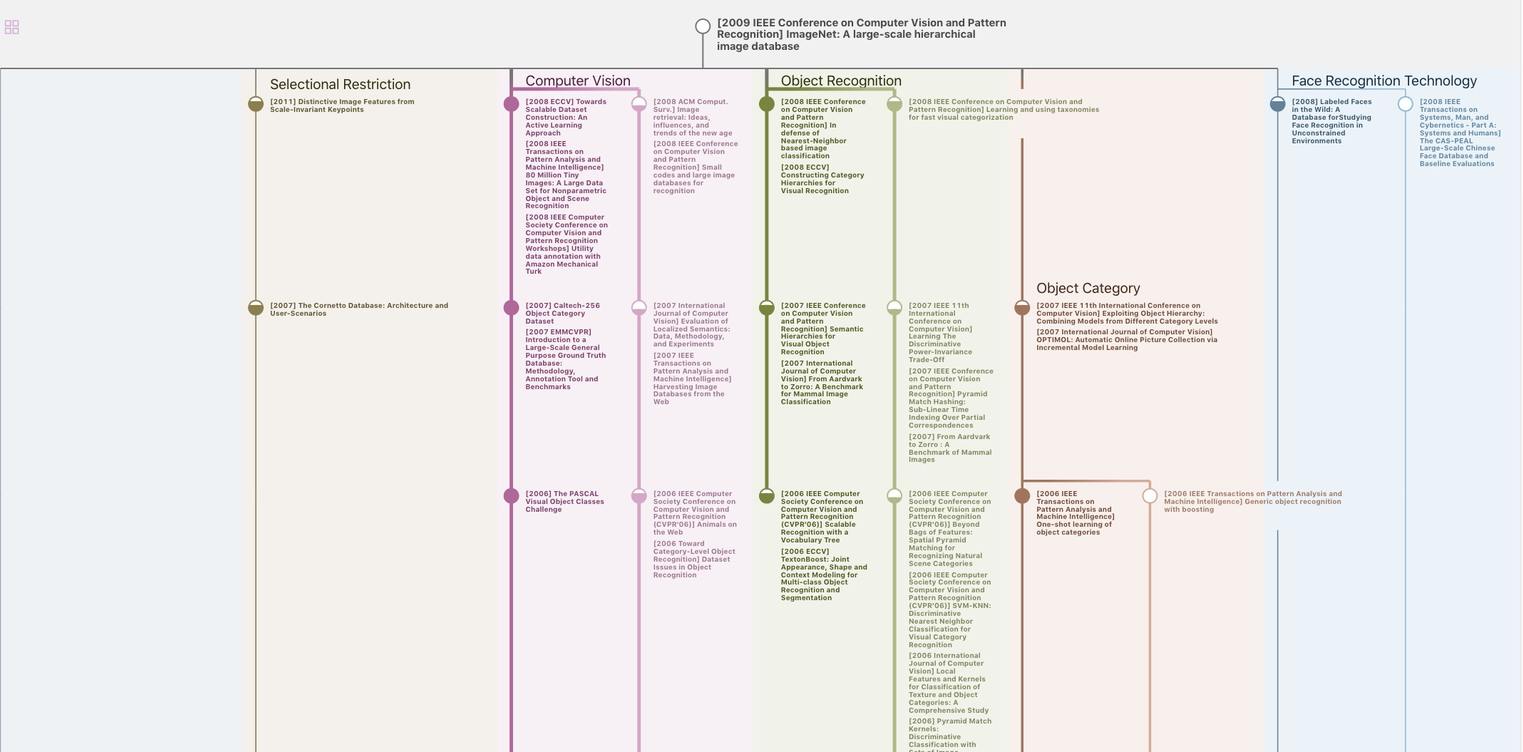
生成溯源树,研究论文发展脉络
Chat Paper
正在生成论文摘要