Dental Material Detection based on Faster Regional Convolutional Neural Networks and Shape Features
Neural Processing Letters(2022)
Abstract
In this paper, a novel approach is proposed to detect the dental materials in dental Panoramic images. In general, dental Panoramic images are examined by the dentists to detect the dental materials of the patients. Examining the dental Panoramic images is a time-consuming procedure and necessitates highly skilled dentists. Thus, computer imaging and decision support systems based on machine learning are in demand for dental applications. In this study, a semi-automatic, image processing and machine learning based approach is proposed for detection of dental materials in dental Panoramic images. More specifically, the dental materials namely dental filling, dental bridge and tooth crown are targeted by the proposed approach. Within the approach developed, the dental Panoramic images used as input are initially pre-processed for noise removal and tooth region is determined using a deep learning approach. To this end, a faster Regional Convolutional Neural Networks (RCNN) method is employed. A tooth region correction procedure is applied after application of faster RCNN to determine the exact location of the tooth region as faster RCNN may produce more tooth regions than one correct tooth region. Graph cut (GC) based image segmentation is applied to segment the tooth region into foreground and background regions. Shape based feature extraction and classification are applied to determine the tooth problem. A dataset has been established in dental faculty hospital of private dental clinics. The proposed approach is simple yet quite effective. In all classification methods, the average accuracy scores were around 90%.
MoreTranslated text
Key words
Dental material detection,Faster RCNN,Shape Features,Segmentation,Classification
AI Read Science
Must-Reading Tree
Example
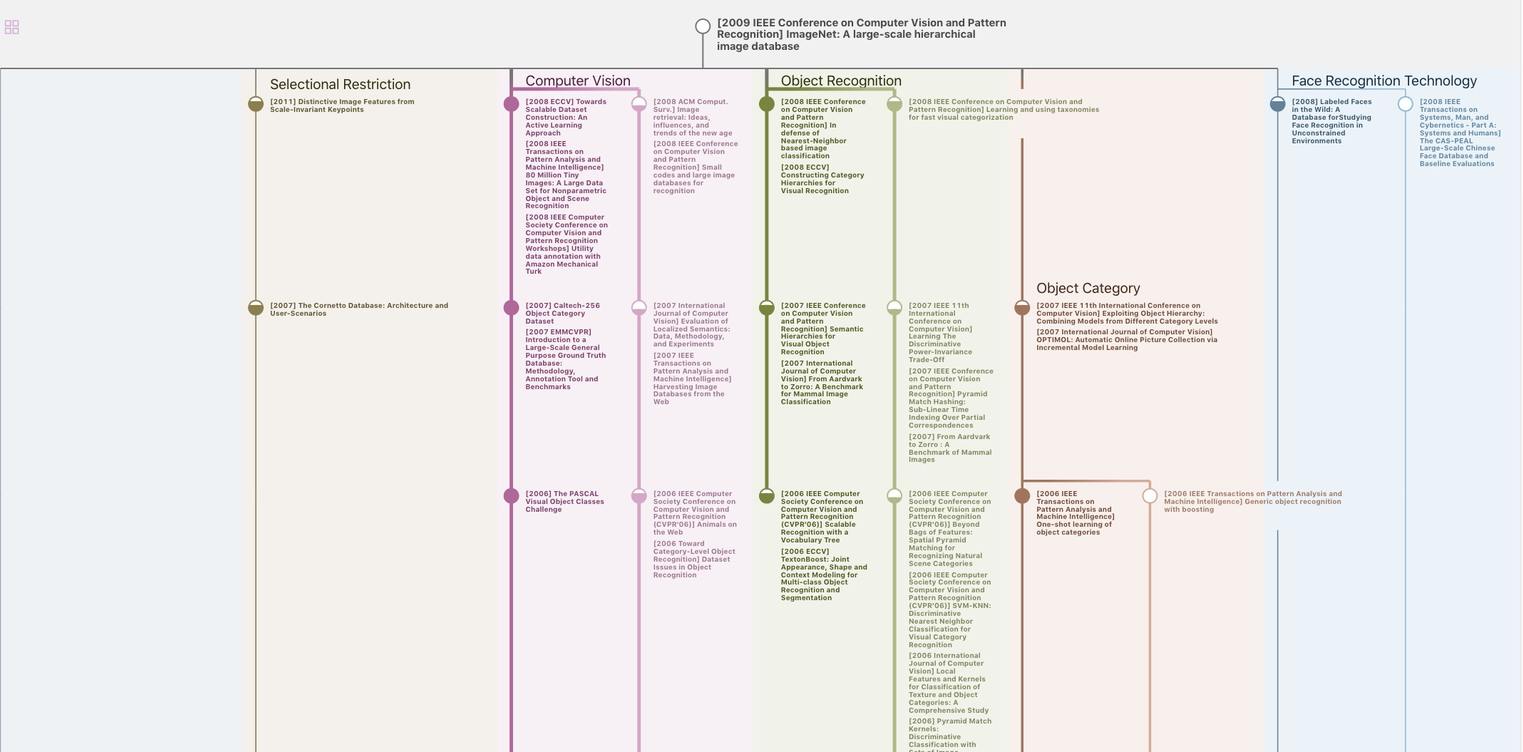
Generate MRT to find the research sequence of this paper
Chat Paper
Summary is being generated by the instructions you defined