Using machine learning to detect PII from attributes and supporting activities of information assets
The Journal of Supercomputing(2022)
Abstract
Since the implementation of the EU General Data Protection Regulation (“GDPR”) and similar legislation on personal data protection in Taiwan, enterprises must now provide adequate protection for their customers’ personal data. Many enterprises use automated personally identifiable information (“PII”) scanning systems to process PII to ensure full compliance with the law. However, personal data saved in non-electronic form cannot be detected by these automated scanning systems, resulting in PII not being able to be accurately identified. We propose a random forest (“RF”) approach to detect unidentified PII to close the loopholes. Relevant peripheral information attributes of PII are identified and used in our study for machine learning and modeling to establish a model for detecting PII that otherwise cannot be detected by automated scanners. Our study shows that the F1-measure of our proposed model achieves at least 90%, a higher accuracy rate than that of automated scanners in detecting PII in an enterprise’s inventory of information assets. Finally, the results of the experiment in our study show that our proposed model can shorten the time required for detecting PII by 100 times and increase the F1-measure by 2% when compared with the PII detection conducted manually.
MoreTranslated text
Key words
Personally Identifiable Information,Machine Learning,Time Evaluation
AI Read Science
Must-Reading Tree
Example
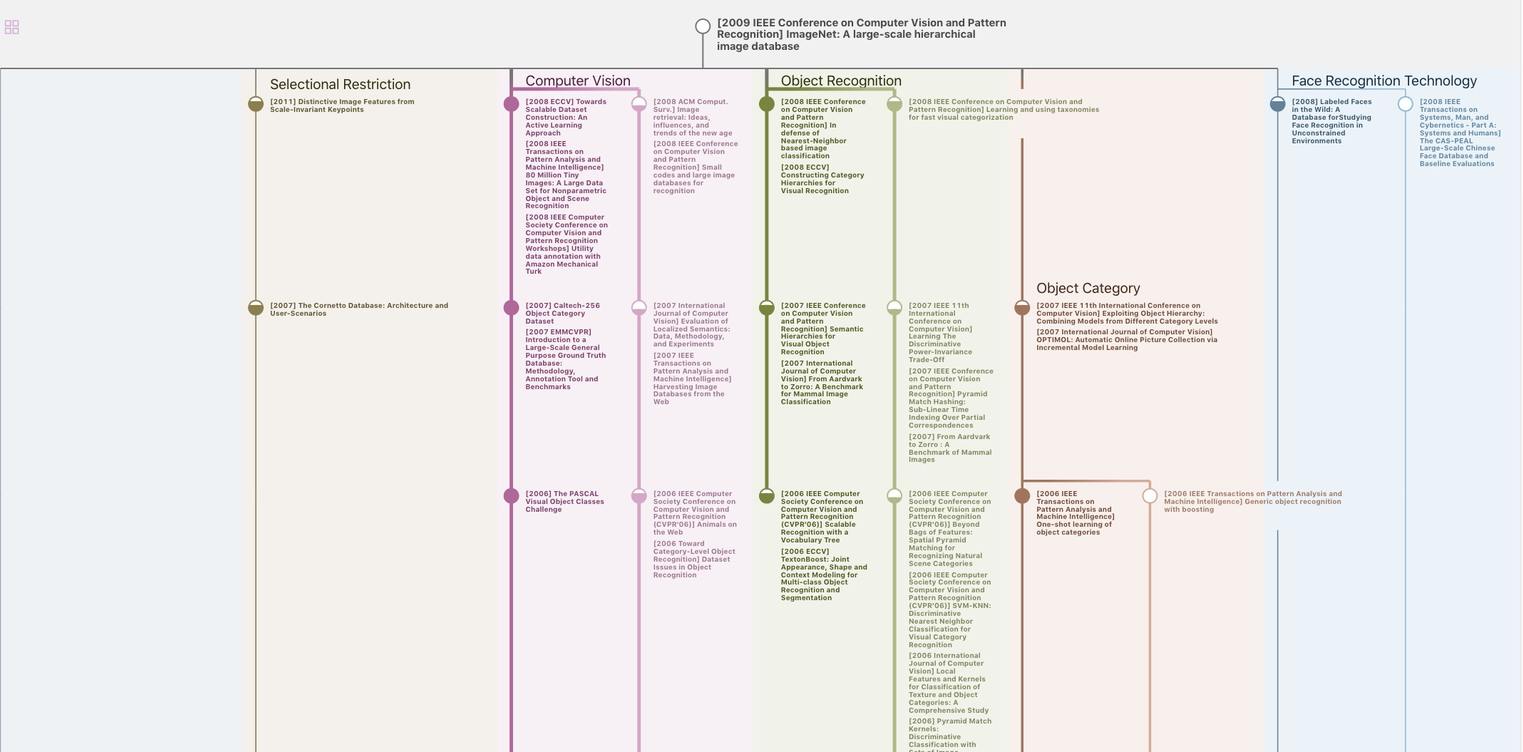
Generate MRT to find the research sequence of this paper
Chat Paper
Summary is being generated by the instructions you defined