JSENet: A deep convolutional neural network for joint image super-resolution and enhancement
Neurocomputing(2022)
摘要
Super-resolution (SR) and image enhancement (IE) are two common and important tasks in image processing. Due to poor equipment, illumination, and photographic skills, many low-resolution and low-quality (in terms of color, and contrasts) photos are captured in our daily life. To make the photos more visually pleasing, we usually enlarge and retouch them in two separate steps. In this paper, we propose a new Joint Image Super-resolution and Enhancement Network (JSENet), which is arguably the first end-to-end method based on deep learning for joint SR and IE. Compared with simply conducting these two tasks in parallel or sequentially, JSENet employs the bilateral learning framework to integrate the two tasks together seamlessly. Meanwhile, two lightweight modules are designed for restoring details and generating color transformation coefficients respectively, which ensures that our JSENet can be deployed in real-world applications. Extensive experiments are conducted to demonstrate that JSENet outperforms the state-of-the-art SR-IE methods.
更多查看译文
关键词
Convolutional neural network,Image super-resolution,Image enhancement
AI 理解论文
溯源树
样例
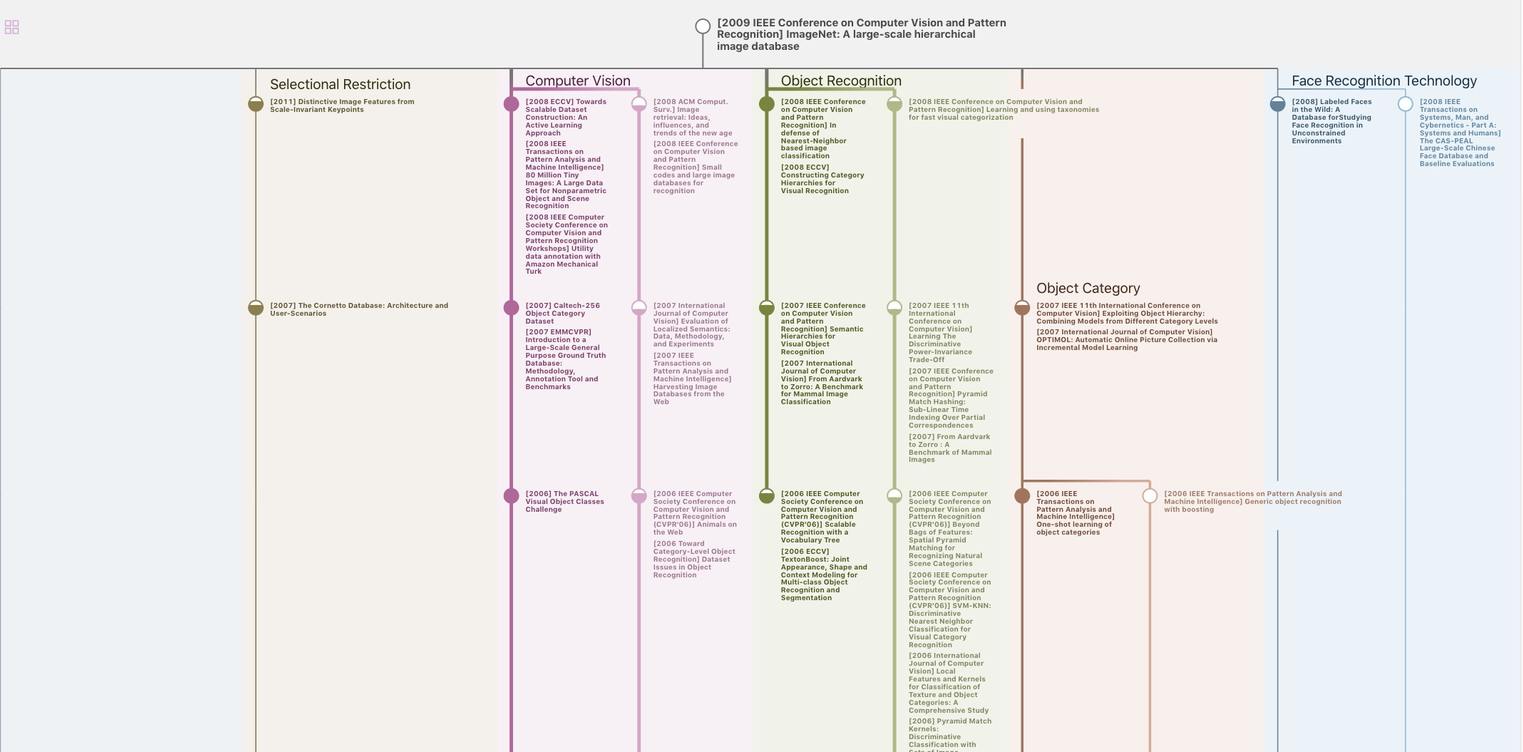
生成溯源树,研究论文发展脉络
Chat Paper
正在生成论文摘要