Airfoil design and surrogate modeling for performance prediction based on deep learning method
PHYSICS OF FLUIDS(2022)
Abstract
Aiming at the problems of a long design period and imperfect surrogate modeling in the field of airfoil design optimization, a convolutional neural network framework for airfoil design and performance prediction (DPCNN) is established based on the deep learning method. The airfoil profile parameterization, physical field prediction, and performance prediction are achieved. The results show that the DPCNN framework can generate substantial perfect airfoil profiles with only three geometric parameters. It has significant advantages such as good robustness, great convergence, fast computation speed, and high prediction accuracy compared with the conventional machine learning method. When the train size is 0.1, the predicted results can be obtained within 5 ms. The prediction absolute errors of physical field of most sample points are lower than 0.002, and the relative errors of aerodynamic performance parameters are lower than 2.5%. Finally, the optimization attempt of operating parameters is completed by gradient descent method, which shows good stability and convergence. Overall, the DPCNN framework in this paper has outstanding advantages in time cost and prediction accuracy.
MoreTranslated text
Key words
deep learning method,surrogate modeling,performance prediction,deep learning
AI Read Science
Must-Reading Tree
Example
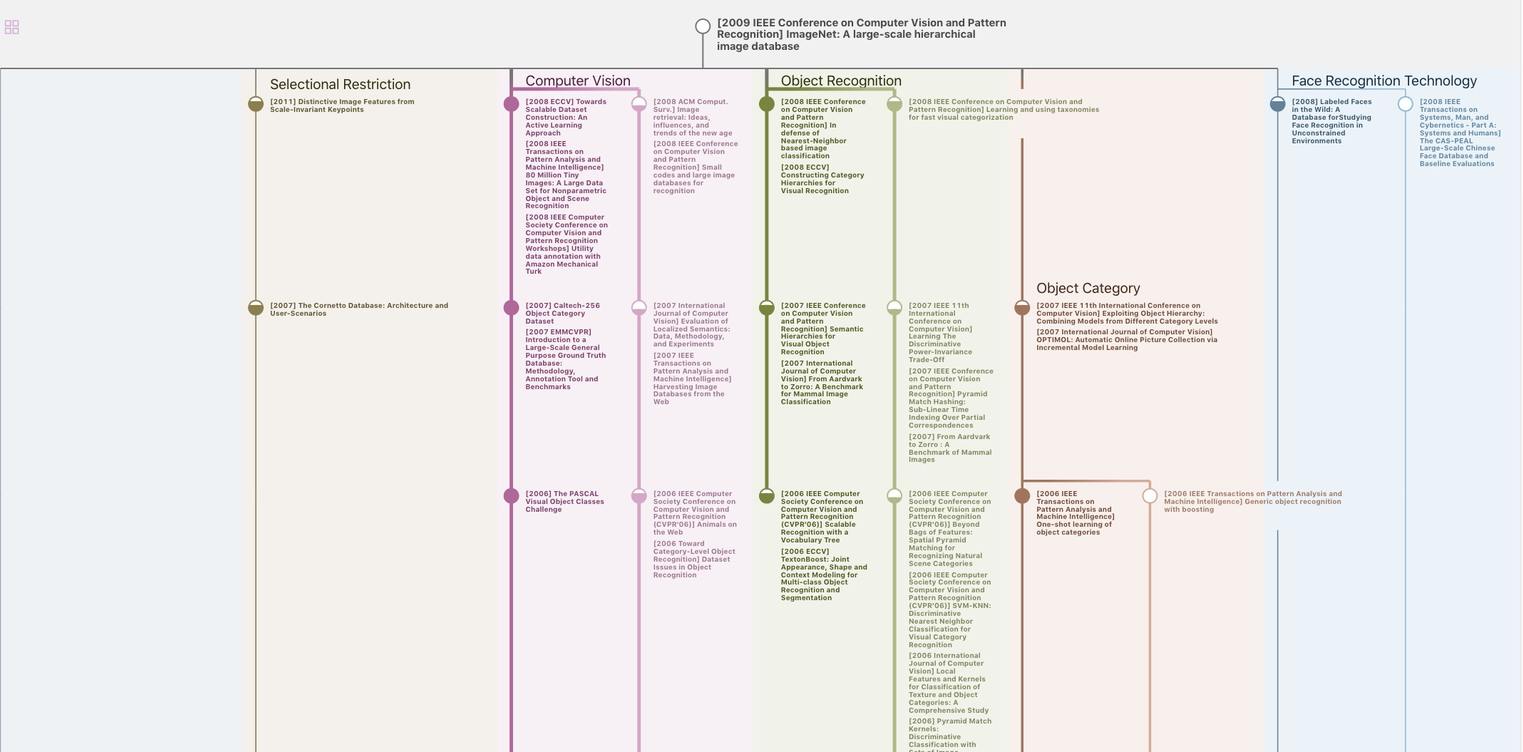
Generate MRT to find the research sequence of this paper
Chat Paper
Summary is being generated by the instructions you defined