Noise-Suppressing Neural Dynamics for Time-Dependent Constrained Nonlinear Optimization With Applications
IEEE Transactions on Systems, Man, and Cybernetics: Systems(2022)
摘要
Up to date, the existing methods for nonlinear optimization with time-dependent parameters can be classified into two types: 1) static methods are capable of handling inequality constraints but may generate large lagging errors in the solution of the intrinsically time-dependent constrained nonlinear optimization (TDCNO) problem due to the hypothesis of short-time invariance and 2) time-variant methods, e.g., zeroing neural networks, are able to remedy the lagging error but fail to solve the TDCNO problem under inequality constraints. To resolve this contradiction, a noise-suppressing neural dynamics (NSND) model is proposed to solve the TDCNO problem subject to both equality and inequality constraints via the nonlinear complementary problem (NCP) function. The proposed method allows inequality constraints for unknown variables, removes the short-time invariance hypothesis, and further eliminates lagging errors during the solving process in the presence of noises. Besides, the rapid convergence, global stability, and noise processing of the NSND model are verified by the theoretical analyses. Simulation results of illustrative examples, including dimensionality reduction on principal component analyses (PCA) and a robot motion control, show that the NSND model outperforms the existing models for the TDCNO problem.
更多查看译文
关键词
Constrained nonlinear optimization,dimensionality reduction on principal component analyses (PCA),equality and inequality constraints,neural dynamics,noise suppression,robot arm control
AI 理解论文
溯源树
样例
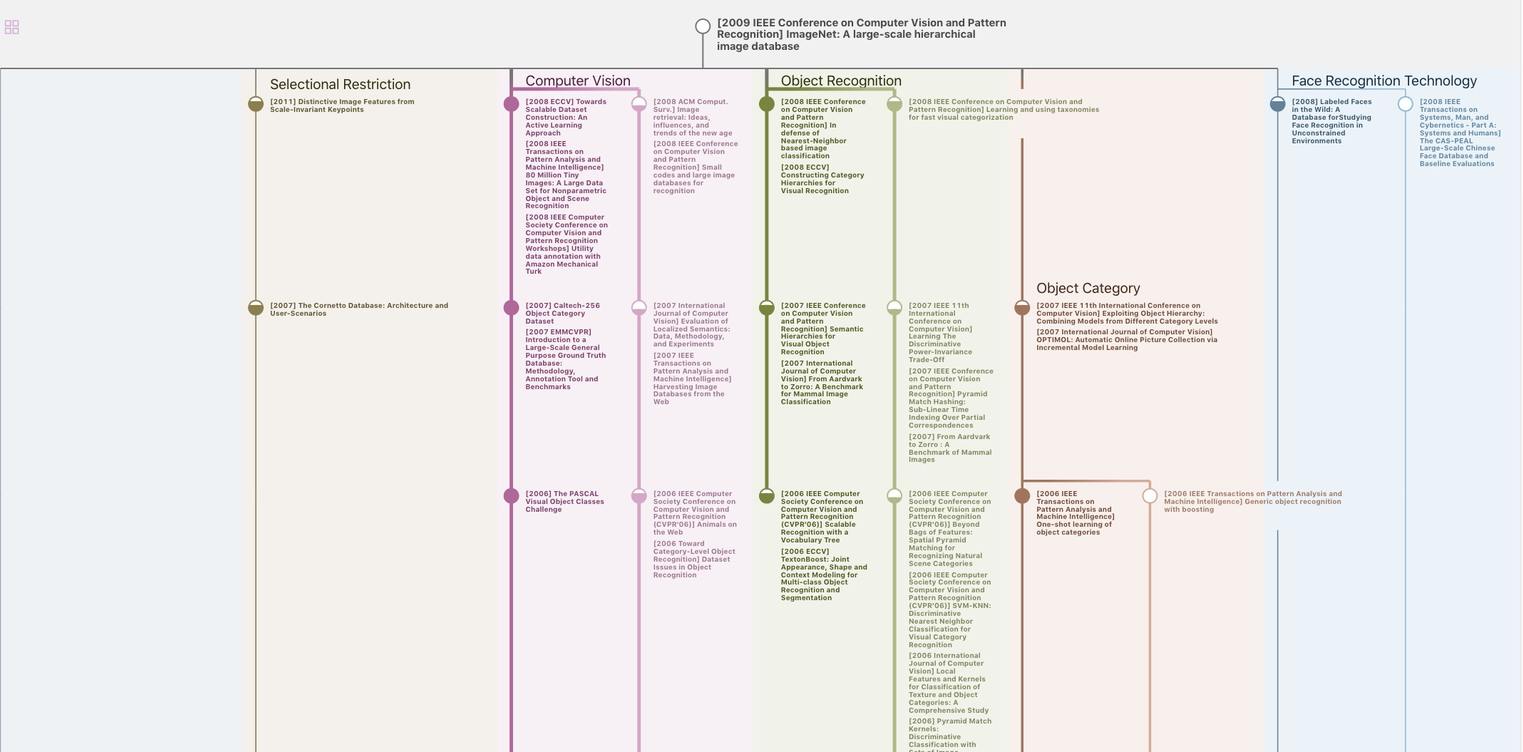
生成溯源树,研究论文发展脉络
Chat Paper
正在生成论文摘要