Deep Online Video Stabilization Using IMU Sensors
IEEE Transactions on Multimedia(2023)
摘要
In this paper, we propose a deep learning based sensor-driven method for online video stabilization. This method utilizes the Euler angles and acceleration values estimated from the gyroscope and accelerator to assist stable video reconstruction. We introduce two simple sub-networks for trajectory optimization. The first network exploits real unstable trajectories and camera acceleration values to detect shooting scenarios. This network also generates an attention mask to adaptively choose scenario-specific features. Then the second network predicts smooth camera paths based on real unstable trajectories using long short-term memory (LSTM) under the supervision of the above mask. The output of the trajectory optimization network is filtered with a two-step modification process to guarantee smoothness. The real and smoothed camera paths are then utilized as guidance to generate stable frames in a projective manner. We also capture videos with sensor data covering seven typical shooting scenarios and design a ground truth generation method to construct pseud-labels. Moreover, the trajectory smoothing network allows the use of 3- or 10-frame buffers as future information to construct a lookahead filter. Experimental results show that our online method could outperform other state-of-the-art offline methods in several shaky video clips with fewer buffer frames for both general and low-quality videos. Furthermore, our method could effectively reduce running times without performing image content analysis, and the stabilization efficiency reaches 25 fps on 1080p videos.
更多查看译文
关键词
LSTM, IMU, online video stabilization
AI 理解论文
溯源树
样例
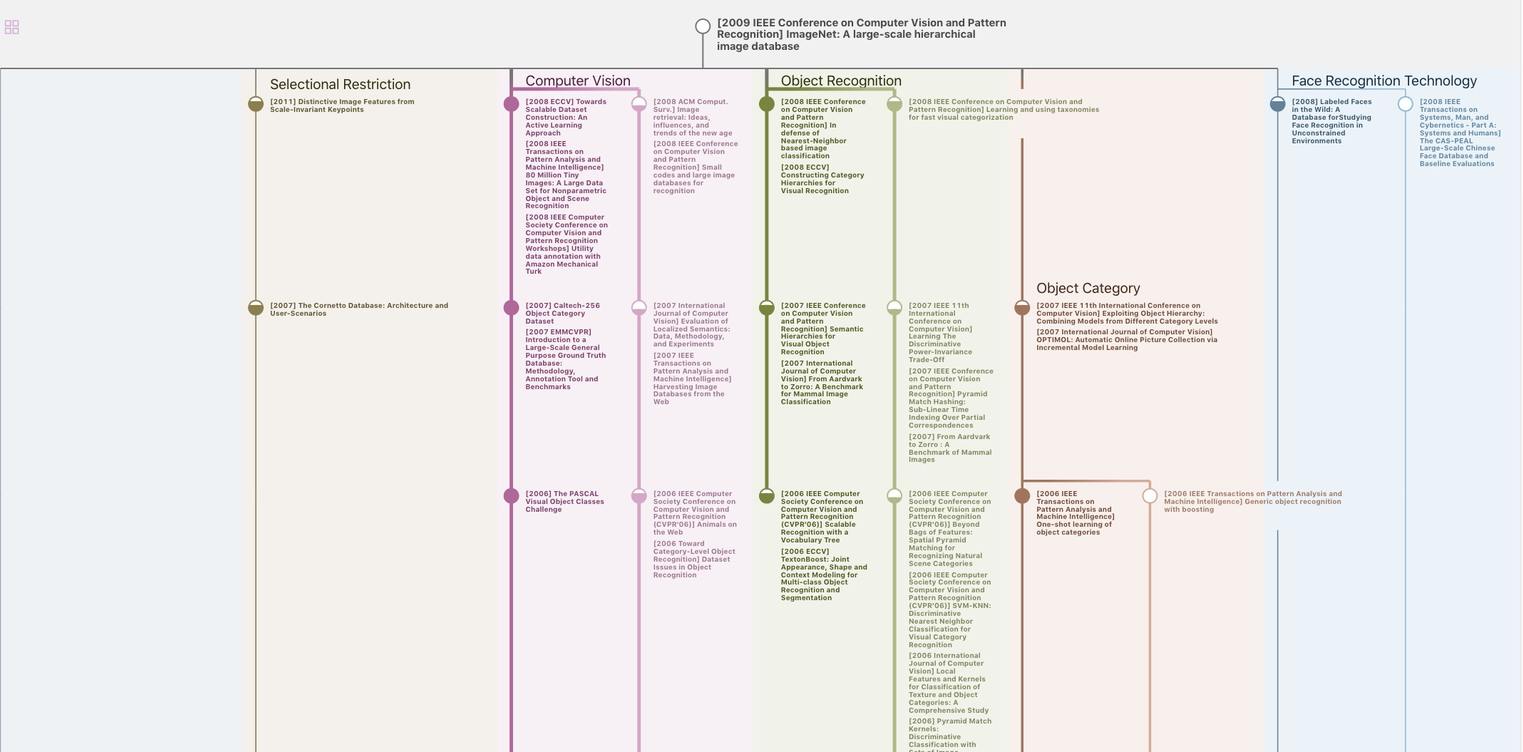
生成溯源树,研究论文发展脉络
Chat Paper
正在生成论文摘要