Relational attention-based Markov logic network for visual navigation
The Journal of Supercomputing(2022)
摘要
We argue the agent’s low generalization problem for searching target object in challenging visual navigation could be solved by "how" and "where" allowing the agent utilizing the scene priors. Although, recent works endow scene priors as fixed spatial features to provide good generalization in novel environment. However, these priors cannot adapt to new scenes. How to build scene priors and where to use the priors in visual navigation has not been well explored. We propose visual relationship detection module to adaptively build relational scene graph as priors. Besides, in order to use priors, we propose Graph attention Markov logical inference Network (GMN) module, which encodes the scene priors and performs precise action inference. GMN updates the graph structure in an unknown scene and estimates the shortest path in scene graph, whose emission probabilities from path to actions are pointwised by action samples in reinforcement learning to get optimal navigation policy. The whole navigation framework is driven by unsupervised reinforcement learning (RL) to exploit the environment. We conduct experiments on the AI2THOR virtual environment, and the results outperform the current most state-of-the-art both in SPL (Success weighted by Path Length) and success rate.
更多查看译文
关键词
Graph attention network,Visual navigation,Markov logical network,Visual relationship Detection
AI 理解论文
溯源树
样例
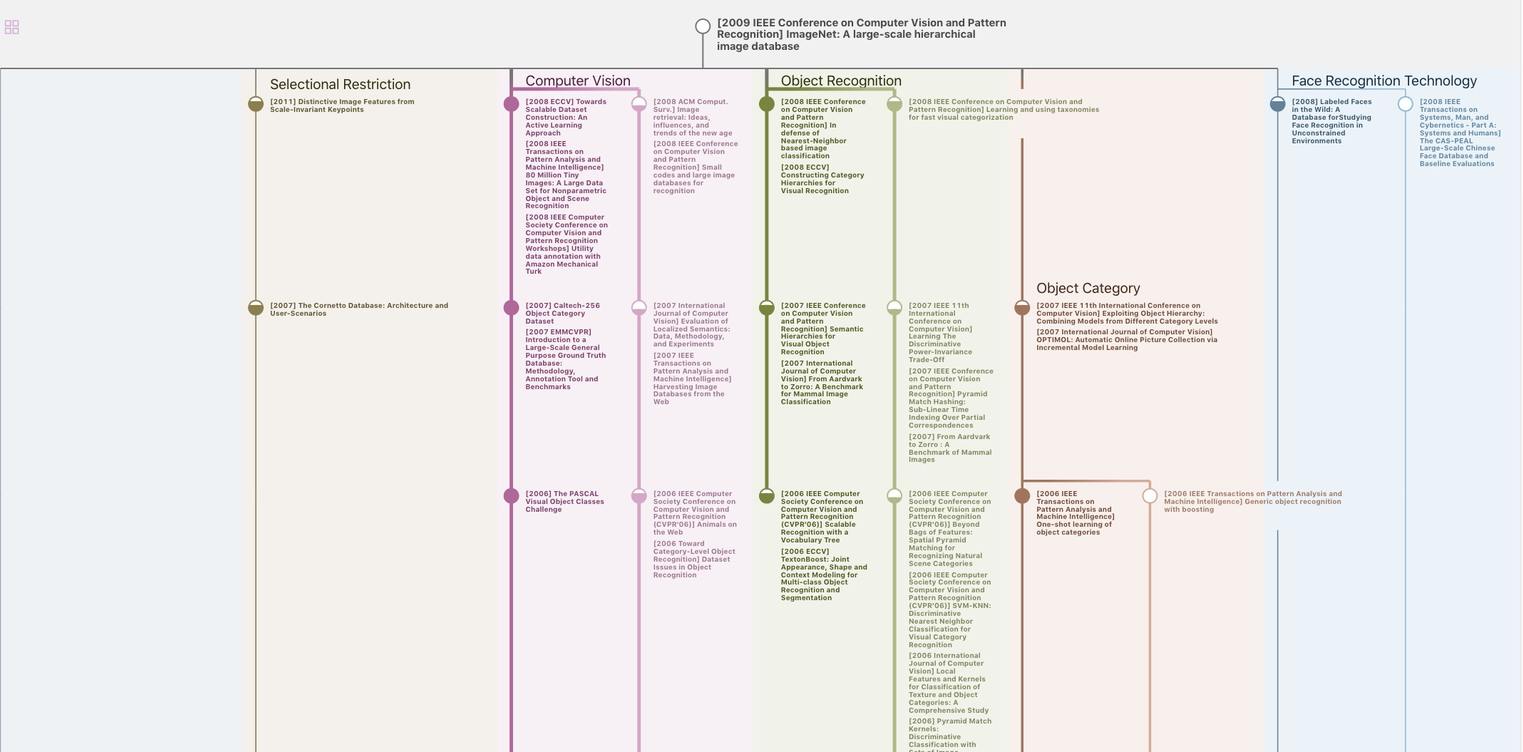
生成溯源树,研究论文发展脉络
Chat Paper
正在生成论文摘要