FSO-LSTM IDS: Hybrid Optimized and Ensembled Deep-Learning Network-Based Intrusion Detection System for Smart Networks
The Journal of supercomputing/Journal of supercomputing(2022)
摘要
The Internet of Things (IoT) has achieved exponential growth worldwide. Although the IoT is used by millions of users, these networks are handicapped by attacks such as denial of service, man in the middle, and spoofing. These attacks threaten the entire IoT ecosystem and affect the integrity and security of the user. Hence, the prediction and identification of novel network attacks in an IoT network remains a challenge for researchers. Recently, machine learning and deep learning have played a pivotal role in predicting and classifying different attacks in an IoT network. However, these algorithms suffer from computational complexity as the number of attacks increases. Hence, a novel hybrid optimized long short-term memory (LSTM) approach is proposed. Whereas a convolutional neural network is used to extract the temporal and spatial correlated features of the IoT network, the optimized LSTM is used to predict the different attacks in the network. Furthermore, firefly swarm optimization is integrated with LSTM to reduce the computational overhead, which in turn increases the prediction accuracy. Nearly 19,00,503 real-time normal and attack data were collected from the experimental simulation setup based on the OMNET++–Python–IoT framework. Extensive experimentation was carried out to evaluate the proposed algorithm, and various metrics, such as accuracy, sensitivity, specificity, and F1-score, were calculated and compared with state-of-the-art learning-based network intrusion detection systems. Furthermore, other benchmarks, such as the CIDCC-15, UNSW-NB15, and NSL-KDD datasets, were used to evaluate the performance of the different deep-learning-based intrusion detection systems. The results demonstrate that the proposed deep-learning method outperforms other classical learning models with low complexity and high prediction performance.
更多查看译文
关键词
Internet of Things,DoS,MIM,Optimized LSTM,OMNET++,Python,IoT framework
AI 理解论文
溯源树
样例
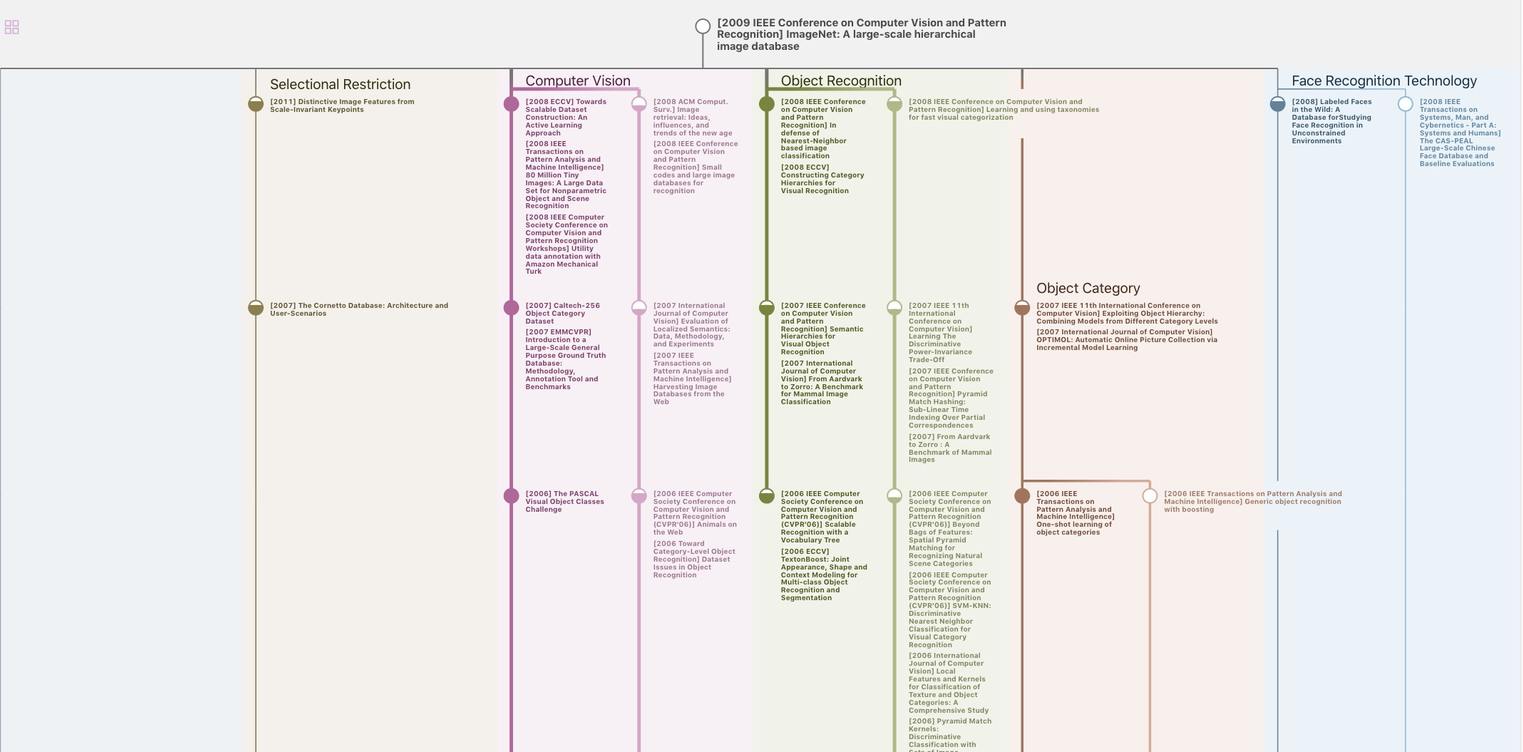
生成溯源树,研究论文发展脉络
Chat Paper
正在生成论文摘要